How Does ChatGPT Work?
Written By
Published On
Read Time
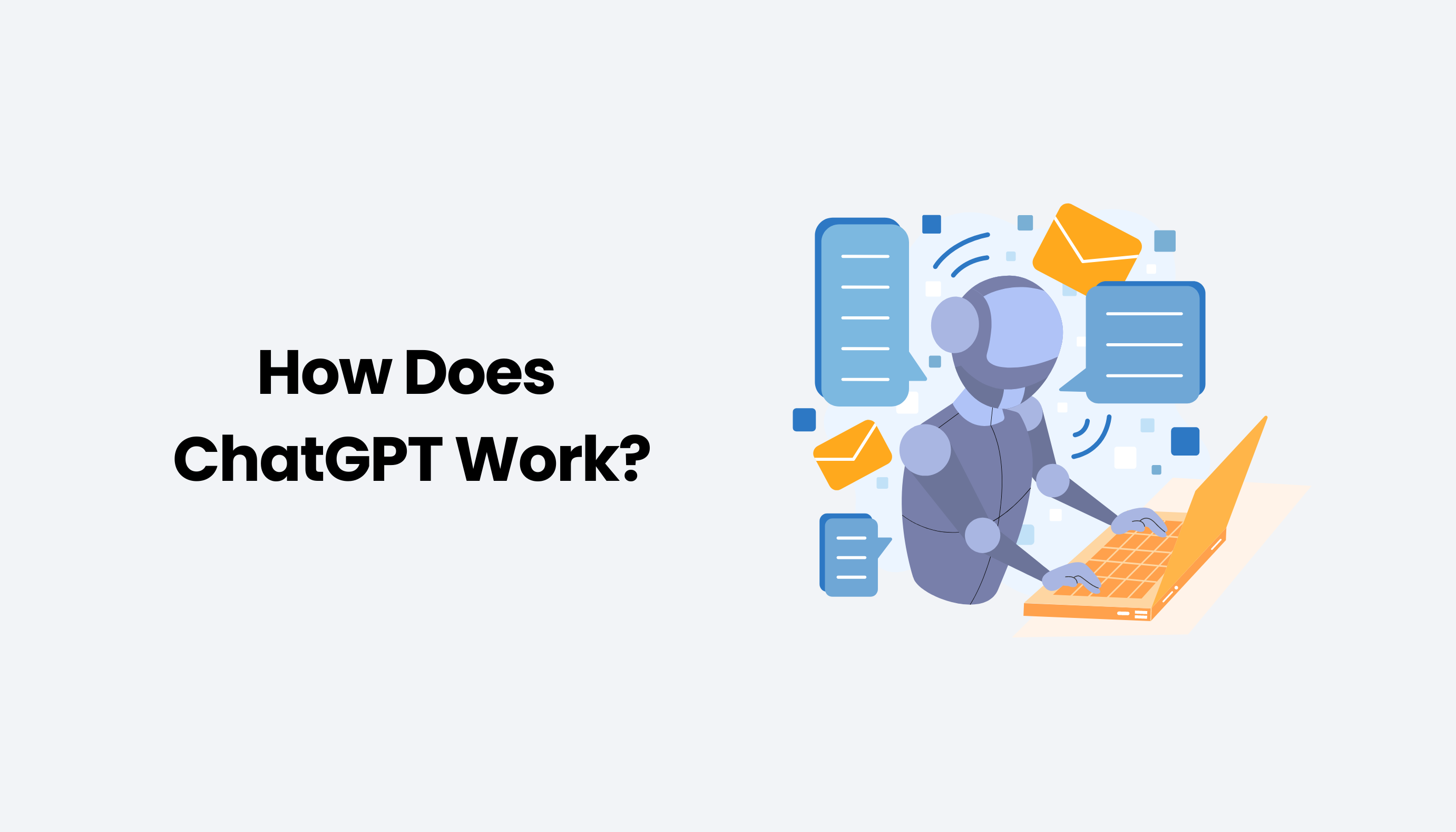
ChatGPT is a new technology that could change how we interact with computers. It lets people have conversations with machines as if they were speaking to another person, something that wasn’t possible before. In this article, we’ll take a closer look at how does ChatGPT works. We'll break down its parts and features, explain the ideas behind it, and show you how it functions.
What is ChatGPT?
ChatGPT, short for "Chat Generative Pre-trained Transformer," is an advanced AI chatbot developed by OpenAI. It's designed to understand and generate human-like text responses based on a vast dataset of text inputs. The core of ChatGPT is built upon the GPT (Generative Pre-trained Transformer) technology, specifically a large language model (LLM) that uses deep learning techniques to produce responses that resemble natural human conversation. ChatGPT is capable of a wide range of tasks, including answering questions, generating creative content, translating languages, providing coding assistance, and more. The AI model aims to simulate human conversation and improve user experience through natural language processing (NLP).
Advantages of ChatGPT
ChatGPT, developed by OpenAI, offers numerous advantages that set it apart from traditional chatbots and other artificial intelligence models. By leveraging advanced techniques in natural language processing (NLP) and machine learning, ChatGPT has become a powerful tool that is transforming various domains, from customer service and content creation to programming and education. Here's a detailed look at the key benefits of using ChatGPT, focusing on its capabilities and unique features.
1. Enhanced Understanding of Context
One of the primary advantages of ChatGPT lies in its advanced ability to understand context. Unlike traditional chatbots that operate on predefined scripts or limited datasets, ChatGPT uses the transformer architecture, a sophisticated neural network model, to capture the context of each conversation dynamically. This allows it to provide more accurate and relevant responses.
Self-Attention Mechanism: ChatGPT employs a self-attention mechanism that enables it to focus on the most relevant parts of the input, regardless of their position in the sentence. This capability allows ChatGPT to maintain context over longer conversations, providing coherent and contextually appropriate responses.
Natural Language Processing (NLP): ChatGPT's use of NLP techniques ensures that it not only understands the meaning behind individual words and phrases but also grasps the overall context of a conversation. This is crucial for generating human-like and meaningful responses that make sense in the flow of dialogue.
2. Versatility Across Applications
ChatGPT is highly versatile and can be applied in numerous fields and use cases, making it a valuable tool for both personal and professional settings. Here are some of the diverse ways in which ChatGPT is being utilized:
Content Creation: ChatGPT can assist in generating high-quality written content, including articles, blog posts, marketing copy, and social media content. Its ability to understand context and maintain tone makes it suitable for a wide range of writing styles.
Customer Support: Many businesses deploy ChatGPT as a virtual assistant to handle customer inquiries. It can provide instant responses to frequently asked questions, troubleshoot issues, and offer personalized recommendations, enhancing customer satisfaction and reducing the need for human intervention.
Educational Support: ChatGPT is being used in educational settings to assist students with homework, explain complex concepts, and even serve as a tutor in various subjects. Its ability to adapt to different language styles and provide detailed explanations makes it an excellent tool for learning.
Programming Assistance: Developers use ChatGPT to debug code, understand programming concepts, and generate code snippets. This is particularly useful for both novice programmers and experienced developers looking for quick solutions to coding challenges.
3. Continuous Learning and Adaptation
ChatGPT is designed to learn continuously and adapt its responses based on new data and feedback. This is made possible by several advanced machine learning techniques:
Reinforcement Learning from Human Feedback (RLHF): ChatGPT undergoes a process called Reinforcement Learning from Human Feedback, where it learns from human evaluators who rate its responses. This helps the model to refine its outputs over time, making it more accurate and reliable.
Fine-Tuning with Supervised Learning: The model is further fine-tuned using supervised learning, where it is trained on specific datasets to improve its performance in certain tasks. This ensures that ChatGPT remains up-to-date with the latest language patterns and user preferences.
Adaptive Learning: ChatGPT adapts to different conversation styles, user preferences, and even cultural nuances. This adaptability allows it to engage in meaningful interactions with users from diverse backgrounds and respond accurately to various prompts.
4. High Scalability and Accessibility
Another significant advantage of ChatGPT is its scalability and accessibility. OpenAI has made ChatGPT widely available, allowing individuals and organizations to integrate it into their workflows with ease.
API Integration: ChatGPT can be integrated into various platforms and applications through APIs, enabling businesses to enhance their customer service, automate tasks, and improve user engagement. This makes it a flexible tool that can be customized to meet specific business needs.
Free and Open Access: Unlike some AI models that require expensive subscriptions or hardware, ChatGPT is available for free to anyone with internet access. This democratization of AI technology allows a wide range of users, from small businesses to individual content creators, to benefit from its capabilities.
Customizability: Organizations can fine-tune ChatGPT to align with their brand voice and specific use cases. This customization ensures that the AI-generated responses are consistent with the organization’s messaging and meet its unique requirements.
5. Efficient Task Automation
ChatGPT excels at automating routine and repetitive tasks, freeing up valuable time for users to focus on more complex and creative endeavors. This is particularly advantageous in professional environments where efficiency is crucial.
Automated Writing and Content Generation: Businesses can use ChatGPT to automate the creation of emails, reports, marketing materials, and more. This not only saves time but also ensures consistency and quality across various types of content.
Workflow Automation: By integrating with tools like Zapier or IFTTT, ChatGPT can be set up to trigger automated workflows. For example, it can generate responses to customer inquiries, schedule social media posts, or summarize meeting notes, streamlining various business processes.
6. Advanced Technical Capabilities
ChatGPT is built on cutting-edge AI technologies that provide several technical advantages:
Transformer Model's Efficiency: The transformer-based architecture allows ChatGPT to process and generate text more efficiently than older AI models. This results in faster response times and the ability to handle more complex queries.
Large Training Dataset: ChatGPT has been trained on a massive dataset, including text from books, articles, websites, and more. This extensive training enables it to provide information on a wide range of topics and understand diverse linguistic nuances.
Multimodal Capabilities: The latest versions of ChatGPT, such as GPT-4o and GPT-4o mini, are multimodal, meaning they can process not only text but also images and audio. This expands the range of possible applications, such as real-time translation, image recognition, and multimedia content creation.
7. Improved User Experience
ChatGPT enhances the user experience by providing more human-like interactions. Its ability to understand natural language and generate coherent responses makes it feel like a genuine conversation partner.
Personalized Interactions: ChatGPT can adapt its tone and style based on user input, offering a more personalized and engaging experience. This is particularly valuable in customer service scenarios, where a customized approach can lead to higher customer satisfaction.
Error Handling and Clarification: Unlike many traditional bots, ChatGPT can handle ambiguous or unclear queries by asking follow-up questions, ensuring that it accurately understands the user's intent before providing a response.
8. Future-Proof and Continuously Evolving
ChatGPT is continuously being updated and improved to keep pace with advancements in AI technology. OpenAI is committed to refining the model's performance and expanding its capabilities.
Ongoing Development: OpenAI regularly releases new versions of ChatGPT, incorporating the latest research in natural language processing and machine learning. This ensures that users have access to state-of-the-art AI technology.
Community and Developer Support: OpenAI fosters a strong community of developers and researchers who contribute to the ongoing development of ChatGPT. This collaborative approach ensures that the model remains at the cutting edge of AI innovation.
How is ChatGPT Unique?
ChatGPT stands out from traditional chatbots and other artificial intelligence models due to its innovative design and advanced functionalities. Developed by OpenAI, ChatGPT utilizes a combination of state-of-the-art machine learning techniques and natural language processing (NLP) to create more accurate, engaging, and context-aware responses. Here’s an in-depth look at what makes ChatGPT unique and how it differs from other AI tools.
1. Advanced Transformer Architecture
At the core of what makes ChatGPT unique is its use of the transformer architecture, a sophisticated deep learning model that revolutionized natural language processing. Unlike traditional AI models that rely on older methods such as recurrent neural networks (RNNs), ChatGPT’s transformer-based architecture allows it to handle and generate text more efficiently.
Self-Attention Mechanism: The transformer model utilizes a self-attention mechanism that enables ChatGPT to focus on different parts of the input text selectively. This means the model can weigh the relevance of each word in a sentence, regardless of its position, allowing it to understand context and meaning with much higher accuracy.
Parallel Processing Capability: Unlike older models that process text sequentially, ChatGPT’s transformer architecture allows for parallel processing of tokens (chunks of text). This ability to analyze all words in a sentence simultaneously enables faster computation and response times, making ChatGPT highly efficient in generating responses.
2. Comprehensive Training on Diverse Data Sources
Another distinctive feature of ChatGPT is its extensive training on a diverse range of text data, making it a more versatile and general-purpose AI tool.
Large-Scale Data Training: ChatGPT was trained on a vast dataset that includes books, articles, websites, and various forms of written content. This large-scale training gives the model a broad understanding of different topics, writing styles, and contexts, enabling it to respond to a wide range of queries with relevant and accurate information.
Training on High-Quality Data: Unlike some AI models that may only use limited or domain-specific datasets, ChatGPT's training data has been curated and filtered to ensure high quality. This helps the model produce coherent and contextually accurate responses, even for complex or ambiguous prompts.
Dynamic and Ongoing Learning: The use of diverse training data ensures that ChatGPT remains up-to-date with language trends, cultural references, and new knowledge. Although it does not learn from individual user interactions in real time, it continuously evolves through updates and retraining on new data sets, keeping it relevant and capable of handling contemporary language use.
3. Reinforcement Learning from Human Feedback (RLHF)
A unique aspect of ChatGPT’s development is the incorporation of Reinforcement Learning from Human Feedback (RLHF). This innovative training method sets it apart from many other AI models and enhances its ability to produce human-like, contextually appropriate responses.
Human-Guided Fine-Tuning: In the RLHF process, human trainers provide feedback on the model's responses, ranking them based on quality and relevance. The model uses this feedback to adjust its outputs, resulting in more refined and effective communication skills.
Iterative Improvement: The RLHF process involves multiple rounds of human feedback and model adjustments, leading to continuous improvements in performance. This iterative refinement ensures that ChatGPT can handle a wide variety of prompts more accurately and engage in more meaningful, nuanced conversations.
4. Multimodal Capabilities
ChatGPT's multimodal capabilities, particularly in its later versions like GPT-4o and GPT-4o mini, further distinguish it from traditional text-based AI models.
Processing Text, Images, and Audio: While earlier versions of ChatGPT were limited to text inputs, newer versions can understand and generate responses based on text, images, and audio. This multimodal approach expands the range of possible applications, such as providing real-time translations, identifying objects in images, or interacting through voice inputs.
Enhanced Context Awareness: By integrating multiple types of input data, ChatGPT can provide richer, more context-aware responses. For instance, it can interpret an image's content and relate it to a textual query, offering more holistic and relevant information.
5. Context Retention and Conversational Continuity
A significant feature that makes ChatGPT unique is its ability to retain context throughout a conversation. This capability enhances the flow of dialogue, making interactions feel more natural and human-like.
Context Memory: ChatGPT can remember previous inputs and refer back to them in subsequent responses, maintaining continuity and coherence in long conversations. This makes it particularly useful in customer support scenarios, where it can recall details from earlier in the conversation to provide more personalized and accurate assistance.
Adaptive Responses: The model adapts its responses based on the context provided by the user, allowing it to engage in more sophisticated conversations. This adaptability makes ChatGPT suitable for a wide range of applications, from casual chat to professional and technical discussions.
6. Flexibility and Customization
ChatGPT is highly flexible and customizable, offering unique advantages over more rigid AI models.
API Integration and Customization: ChatGPT can be integrated into various platforms and services through APIs, allowing businesses and developers to customize its behavior to suit specific needs. For example, companies can fine-tune the model to align with their brand voice or optimize it for specific customer service tasks.
Adjustable Temperature and Response Style: Users and developers can adjust parameters like the "temperature" setting to control the randomness and creativity of ChatGPT’s responses. A lower temperature setting results in more predictable and conservative responses, while a higher setting encourages more diverse and creative outputs. This flexibility allows ChatGPT to be tailored for different applications and audiences.
7. Enhanced Natural Language Understanding and Generation
ChatGPT’s ability to understand and generate human-like text makes it a standout AI model for natural language processing tasks.
Advanced NLP Techniques: ChatGPT employs cutting-edge NLP techniques to analyze and interpret human language, allowing it to generate coherent, context-aware responses. This involves understanding not just the literal meanings of words but also their nuances, idioms, and cultural references.
Tokenization and Semantic Understanding: The model breaks down input text into tokens—smaller units such as words or phrases—allowing it to analyze and generate text with greater accuracy. It uses these tokens to build a semantic understanding of the text, which is critical for providing meaningful and relevant responses.
8. Continuous Updates and Improvements
OpenAI continually updates and improves ChatGPT, ensuring that it remains at the cutting edge of AI technology.
Ongoing Research and Development: OpenAI regularly releases new versions of ChatGPT, incorporating the latest advancements in AI research. This commitment to continuous improvement ensures that the model stays up-to-date with current trends and technologies.
Community Feedback and Collaboration: OpenAI actively engages with the developer community, researchers, and users to gather feedback and improve ChatGPT. This collaborative approach helps refine the model and expand its capabilities, making it more useful and reliable over time.
9. Broad Application Range and Use Cases
ChatGPT is designed to be a general-purpose AI model that can be applied to a wide variety of tasks and industries.
Cross-Disciplinary Use: From generating creative content and providing customer support to assisting with programming and offering educational resources, ChatGPT’s broad application range makes it suitable for numerous industries, including marketing, healthcare, finance, education, and more.
Task Versatility: ChatGPT is capable of performing a wide range of tasks, such as answering questions, drafting emails, writing reports, creating social media content, translating languages, and even coding. This versatility makes it a valuable tool for both individuals and businesses.
10. User-Friendly Interaction
ChatGPT is designed to offer a user-friendly and accessible experience, making it easy for people of all backgrounds to use.
Simple Interface: The ChatGPT interface is straightforward and intuitive, allowing users to engage with the model effortlessly. This simplicity has helped popularize its use across different demographics, from tech-savvy professionals to casual users.
Real-Time Feedback and Adaptation: The model provides real-time feedback and can adjust its responses based on user input, making the interaction feel more interactive and engaging.
How Does ChatGPT Work?
ChatGPT is an advanced AI language model developed by OpenAI, designed to generate human-like text based on the input it receives. It uses sophisticated techniques in machine learning and natural language processing (NLP) to understand and produce coherent and contextually relevant responses. ChatGPT is built upon the GPT (Generative Pre-trained Transformer) architecture, which plays a crucial role in its ability to handle various text-based tasks effectively. To understand how ChatGPT works, it’s important to explore the underlying processes, training methods, and technologies that enable it to function.
1. Foundation: The GPT Architecture
ChatGPT is based on the Generative Pre-trained Transformer (GPT) architecture, which is a type of neural network specifically designed for processing and generating text. The GPT model, currently in its fourth iteration (GPT-4), is known for its ability to learn from vast datasets and generate human-like responses.
Transformer Architecture: The transformer model is central to how ChatGPT functions. Unlike traditional recurrent neural networks (RNNs) that process text sequentially, the transformer model uses a mechanism called self-attention to process all words in a sentence simultaneously. This approach allows the model to consider the context of all words in relation to each other, enhancing its ability to understand and generate coherent text.
Self-Attention Mechanism: The self-attention mechanism enables the model to focus on different parts of the input text to understand relationships between words, regardless of their position in a sentence. For example, in a sentence like “The cat sat on the mat,” the model identifies the connections between "cat" and "sat" or "mat" and "on" to grasp the overall meaning. This makes the model highly effective in generating responses that are contextually appropriate and grammatically accurate.
2. Data Training and Pre-Training Process
ChatGPT's ability to generate accurate and context-aware responses stems from its extensive training on a vast amount of text data. The training process involves two main stages: pre-training and fine-tuning.
Pre-Training Phase: In the pre-training phase, ChatGPT is exposed to a diverse dataset comprising text from books, articles, websites, and other written materials. The model learns the structure, grammar, and patterns of human language by predicting the next word in a sentence based on the context provided by the preceding words. This process helps the model understand how words and phrases are typically used in various contexts.
Tokenization: Before the training begins, the input text is broken down into smaller units called tokens. Tokens can be individual words, subwords, or even single characters. Tokenization enables the model to handle text in a flexible manner, allowing it to understand complex or rare words and manage different languages effectively.
Byte Pair Encoding (BPE): ChatGPT uses Byte Pair Encoding (BPE) for tokenization, which splits words into sub-word units. This method helps the model handle unknown words by breaking them down into smaller, recognizable components, enhancing its ability to understand and generate diverse vocabulary.
Training Data Sources: ChatGPT is trained on a mixture of datasets that include Common Crawl (a large-scale web crawl), WebText2, Books1, Books2, Wikipedia, and specialized datasets like Persona-Chat. These datasets provide a wide range of language styles, topics, and contexts, which helps the model learn how to respond to a variety of prompts.
3. Fine-Tuning with Human Feedback
After pre-training, ChatGPT undergoes a fine-tuning process to enhance its performance and align it more closely with user expectations.
Supervised Fine-Tuning: In the supervised fine-tuning phase, human trainers provide example prompts and desired responses to the model. This allows the model to learn what constitutes an ideal answer for different types of queries, improving its ability to generate accurate and contextually relevant outputs.
Reinforcement Learning from Human Feedback (RLHF): ChatGPT utilizes a unique training method called Reinforcement Learning from Human Feedback (RLHF). During this process, human evaluators assess the model’s responses and provide feedback on their quality. The model then uses this feedback to adjust its parameters, refining its behavior to produce more accurate, coherent, and helpful responses over time. RLHF is crucial for reducing biases, enhancing dialogue quality, and ensuring the model aligns with human values and preferences.
4. Natural Language Processing and Generation
ChatGPT leverages advanced Natural Language Processing (NLP) techniques to understand and generate human language effectively.
Natural Language Understanding (NLU): The first step in generating a response involves Natural Language Understanding (NLU). The model analyzes the input to identify its grammatical structure, meaning, and intent. It breaks down sentences into their constituent parts, such as words and phrases, and determines their relationships. This helps ChatGPT understand the context and the specific information the user is seeking.
Natural Language Generation (NLG): Once the input is understood, ChatGPT uses Natural Language Generation (NLG) to create a response that is both contextually appropriate and linguistically coherent. The model draws on its extensive training data to predict the next word or phrase, generating responses one token at a time. The use of probabilistic modeling ensures that the generated text is relevant and meaningful, aligning with the user's input.
Response Generation Process: The model calculates the likelihood of various possible responses and selects the one with the highest probability. This approach allows ChatGPT to provide diverse and creative outputs, adding variability to its responses while maintaining accuracy and relevance.
5. Context Retention and Conversational Continuity
One of ChatGPT’s standout features is its ability to maintain context and continuity throughout a conversation, which enhances the user experience.
Context Awareness: ChatGPT retains information from earlier parts of a conversation, allowing it to provide consistent and relevant responses. For example, if a user asks a question about a specific topic and follows up with a related query, ChatGPT remembers the context and provides an answer that aligns with the ongoing dialogue.
Adaptive Responses: The model adapts its responses based on the input context. It can handle follow-up questions, clarifications, or even changes in topic, ensuring that the conversation flows naturally and smoothly. This ability to adapt to different conversational scenarios makes ChatGPT highly effective in diverse applications, from customer support to educational assistance.
6. Multimodal Capabilities
Recent versions of ChatGPT, such as GPT-4o and GPT-4o mini, have introduced multimodal capabilities, allowing the model to process and respond to various types of inputs, including text, images, and audio.
Handling Text, Images, and Audio: ChatGPT is now capable of understanding and generating responses based on multiple input types, such as text, images, and audio. This expands the range of possible applications, including real-time translation, image recognition, and voice-based interaction.
Enhanced Real-World Applications: The multimodal functionality makes ChatGPT more versatile and applicable in real-world scenarios. It can be used for tasks like identifying objects in images, converting speech to text, or providing assistance through voice commands.
7. Technical Infrastructure and Scalability
ChatGPT is built on a robust technical infrastructure that allows it to handle large amounts of data and generate responses quickly and efficiently.
Large-Scale Model with Billions of Parameters: ChatGPT is a large language model with billions of parameters. These parameters are the adjustable elements that the model fine-tunes during training to minimize errors and optimize performance. The size of the model enables it to capture subtle language nuances, making it capable of generating more accurate and contextually relevant responses.
Cloud-Based Deployment and API Integration: ChatGPT is deployed on cloud servers and can be integrated into various platforms and applications through APIs. This makes it accessible and scalable, allowing developers and businesses to incorporate ChatGPT into their software, websites, and applications seamlessly.
8. Customizability and Flexibility
ChatGPT’s design allows for extensive customization and flexibility, making it suitable for a wide range of applications across different industries.
API Access for Developers: OpenAI provides API access to ChatGPT, enabling developers to customize its behavior and integrate it into their platforms. This flexibility allows businesses to tailor ChatGPT for specific use cases, such as customer service, content generation, or technical support.
Adjustable Parameters for Output Control: Users and developers can adjust various parameters, such as the “temperature” setting, to control the randomness of the model's responses. A lower temperature value makes responses more deterministic, while a higher value encourages more creativity and variability. This flexibility makes ChatGPT adaptable to different applications and audiences.
9. Continuous Learning and Updates
ChatGPT is continually updated and improved to remain at the cutting edge of AI technology.
Regular Model Updates: OpenAI frequently releases new versions of ChatGPT, incorporating the latest advancements in machine learning and NLP. These updates enhance the model’s performance, improve its understanding of complex language patterns, and expand its knowledge base.
User Feedback and Community Collaboration: OpenAI actively gathers feedback from users and developers to refine ChatGPT’s capabilities. This collaborative approach helps identify areas for improvement, ensuring that the model remains aligned with user needs and expectations.
10. Safety and Ethical AI Deployment
OpenAI is committed to deploying ChatGPT in a safe and ethical manner.
Bias Mitigation: ChatGPT is designed to minimize biases in its responses by employing techniques like RLHF and carefully curating its training data. Efforts are made to ensure that the model provides fair and balanced answers, aligning with ethical AI practices.
Transparency and Accountability: OpenAI maintains transparency about how ChatGPT is developed and used, providing clear documentation and guidelines for responsible usage.
Training the AI
ChatGPT, developed by OpenAI, is a sophisticated AI model designed to understand and generate human-like text. The effectiveness of ChatGPT stems from a meticulous training process that involves large-scale data, complex algorithms, and advanced neural networks. To understand how ChatGPT works, it is essential to delve into the specifics of how it is trained, the data used for training, and the critical process of tokenization.
Training Data
The training of ChatGPT involves two primary stages: pre-training and fine-tuning. These stages are designed to help the model learn from vast amounts of text data and adjust its behavior based on human feedback.
Pre-Training Phase: The pre-training stage is the initial phase where ChatGPT learns language patterns, grammar, facts, and general knowledge from a large dataset. The model is exposed to a wide range of text sources, including books, articles, websites, and other textual data. The objective is for ChatGPT to learn to predict the next word in a sequence, given all the previous words within a certain context. This approach enables it to understand the structure and style of human language.
Learning by Prediction: During pre-training, the model processes billions of words and sentences to recognize patterns in how words and phrases are used. The learning algorithm involves predicting the next word in a sentence or phrase. For example, if given the prompt “The cat sat on the,” ChatGPT learns to predict “mat” by analyzing similar patterns in the training data. This task is performed repeatedly across a massive dataset, allowing the model to learn language constructs effectively.
Fine-Tuning Phase: After pre-training, ChatGPT enters the fine-tuning phase, where it is further refined using a smaller, more specific dataset. This stage involves human trainers who provide feedback on the model's outputs, rating responses based on their relevance and accuracy. This feedback is then used to adjust the model’s parameters, optimizing it for more specific tasks or use cases. The fine-tuning process is crucial for aligning the model with human preferences and ensuring that it generates useful, safe, and contextually appropriate responses.
Reinforcement Learning from Human Feedback (RLHF): A critical component of the fine-tuning phase is the use of Reinforcement Learning from Human Feedback (RLHF). Here, human evaluators rank multiple outputs generated by the model, and the AI is trained to prioritize outputs that receive higher scores. This iterative feedback loop helps improve the model's performance over time, ensuring that it becomes more adept at understanding user intent and generating meaningful responses.
Tokenization
Tokenization is a key step in the training process that involves breaking down text into smaller units called tokens. This process is essential for enabling ChatGPT to understand and generate language effectively.
What is Tokenization? Tokenization is the process of converting raw text into manageable pieces—tokens—that the model can analyze and process. Tokens can be as small as individual characters or as large as whole words, depending on the language and the specific context.
Byte Pair Encoding (BPE) Method: ChatGPT uses a method called Byte Pair Encoding (BPE) for tokenization. BPE splits words into sub-word units or fragments based on the frequency of occurrence in the training data. For example, the word “unhappiness” might be split into the tokens “un-,” “happi-,” and “-ness.” This approach helps the model handle rare or unknown words more effectively, as it can piece together the meaning from smaller, recognizable components.
Handling Rare and Compound Words: Tokenization allows ChatGPT to understand and generate complex and compound words that may not have been explicitly seen during training. By breaking words down into smaller units, the model can generalize from known sub-word tokens to generate novel or less common words.
Efficiency in Data Processing: Tokenization also improves the efficiency of data processing. By converting text into tokens, the model can handle larger datasets and perform computations more quickly. Each token is assigned a unique integer that represents its meaning within the model’s neural network. This representation enables ChatGPT to process text efficiently and generate responses in real time.
Improving Language Understanding: Tokenization is crucial for understanding context and maintaining the integrity of the input text. It enables ChatGPT to analyze the relationships between words, phrases, and sentences, thereby enhancing its ability to produce coherent and contextually appropriate responses. For example, tokenization helps the model distinguish between homonyms or words with multiple meanings by considering the surrounding context.
Neural Network Development
The development of the neural network is fundamental to understanding how ChatGPT works. ChatGPT is built on a sophisticated neural network known as a transformer model. This architecture forms the core of the Generative Pre-trained Transformer (GPT) framework, which powers ChatGPT’s ability to understand and generate human-like text. To understand the intricacies of how ChatGPT actually works, it is essential to explore the neural network's development, its architecture, and the role it plays in processing and generating responses.
1. What is a Neural Network?
A neural network is a complex computational model inspired by the structure and function of the human brain. It is composed of layers of interconnected nodes (or "neurons") that process information by performing calculations on input data. Each connection between neurons has an associated weight that determines the strength of the signal being transmitted. These weights are adjusted during training to minimize errors in predictions and improve the model's performance.
Structure of the Neural Network: The neural network used in ChatGPT is known as a transformer model, which comprises multiple layers of neurons arranged in an encoder-decoder structure. The transformer model is unique because it uses an attention mechanism that enables it to weigh the importance of different parts of the input data, allowing for more accurate and context-aware responses.
Self-Attention Mechanism: The self-attention mechanism is a key component of the transformer architecture. It allows the model to focus on relevant words in a sentence while understanding their relationships to one another, regardless of their position. For instance, in the sentence "The cat sat on the mat," the model can determine that "cat" and "sat" are related and that "mat" is the object of the action. This capability helps the model generate coherent and contextually relevant responses by understanding the meaning and context of words in relation to each other.
2. Training the Neural Network
The development of ChatGPT's neural network involves a training process that consists of several stages designed to optimize the model's ability to generate accurate and relevant text.
Parameter Adjustment: During training, the neural network's parameters (such as weights) are adjusted to minimize the error between its predicted outputs and the actual results. For example, in a text prediction task, the model adjusts its weights based on how accurately it predicts the next word in a sentence given all the preceding words. This process involves millions or even billions of parameters, which are fine-tuned during training to improve performance.
Transformer Layers: ChatGPT's transformer model consists of multiple layers, each responsible for different aspects of processing. These layers include:
Attention Layers: Focus on identifying relevant parts of the input data.
Feedforward Layers: Perform mathematical transformations to learn complex patterns in the data.
Output Layers: Generate the final output based on the computations of the previous layers.
The combination of these layers enables the model to learn from large-scale datasets and understand intricate language patterns.
Scaling the Model: ChatGPT's neural network is extremely large, with billions of parameters. The size of the model allows it to capture subtle nuances in language and understand complex contexts. For instance, GPT-3, one of the models behind ChatGPT, has 175 billion parameters. These parameters represent different weights assigned to connections between neurons, which help the model learn and store knowledge from vast datasets.
Supervised vs. Unsupervised Learning
Training ChatGPT involves both supervised and unsupervised learning techniques. These methodologies are integral to how ChatGPT is trained and developed to generate accurate and context-aware responses. Understanding the difference between these two learning paradigms is crucial to grasping how ChatGPT works.
1. Supervised Learning
Supervised learning is a type of machine learning where the model is trained on a labeled dataset. In this context, the dataset consists of input-output pairs where each input has a corresponding correct output, or "label." The model learns by making predictions and then adjusting its parameters to minimize the error between its predictions and the actual labeled outputs.
Supervised Fine-Tuning: In ChatGPT’s development, supervised learning is used during the fine-tuning phase. Human trainers provide the model with specific prompts and their correct responses. For example, if the input is “Explain how photosynthesis works,” the model is trained on the correct scientific explanation. The model learns from this human-provided data to generate accurate and relevant responses. This process is known as supervised fine-tuning.
Role of Human Feedback: Human evaluators play a critical role in this process by rating the quality of the model's responses. They provide feedback on whether the model's output is correct, relevant, and coherent. This feedback helps the model learn what constitutes a good response, allowing it to refine its understanding and improve its accuracy.
Reinforcement Learning from Human Feedback (RLHF): After supervised fine-tuning, ChatGPT undergoes further refinement using a technique called Reinforcement Learning from Human Feedback (RLHF). In this process, human evaluators rank different responses generated by the model for the same prompt. The model is then trained to generate responses that are more likely to receive higher rankings. This approach helps the model align better with human expectations and produce more reliable outputs.
2. Unsupervised Learning
Unsupervised learning is a type of machine learning where the model is trained on an unlabeled dataset. The model is not given any specific outputs to learn from; instead, it must identify patterns, relationships, and structures within the data on its own.
Pre-Training Phase with Unsupervised Learning: In the context of ChatGPT, unsupervised learning is primarily used during the pre-training phase. The model is exposed to a vast amount of text data from diverse sources (such as books, articles, websites, etc.) without any specific labels. It learns by predicting the next word in a sequence, given all the previous words. For example, if the input is “The sky is,” the model might predict “blue” as the next word based on patterns it has learned from the training data.
Learning Language Patterns: Through this unsupervised learning process, ChatGPT develops a deep understanding of language, grammar, context, and facts about the world. It learns to recognize common phrases, idioms, sentence structures, and the relationships between different concepts. This broad exposure to language enables the model to generate responses that are contextually accurate and human-like.
Generalization Across Topics: Unsupervised learning allows ChatGPT to generalize its understanding across a wide range of topics, styles, and contexts. By learning from diverse datasets, the model becomes capable of responding to a wide variety of queries, from technical questions to casual conversation. This generalization is crucial for making ChatGPT versatile and effective in different applications.
Transformer Architecture and the Algorithm of ChatGPT
Understanding how ChatGPT works requires a deep dive into its underlying architecture and the algorithm that powers it. At the core of ChatGPT is the Transformer Architecture, a breakthrough in natural language processing (NLP) that enables the model to understand context, capture relationships between words, and generate coherent, human-like responses. This section explores how the Transformer Architecture and the specific algorithms used in ChatGPT contribute to its functionality and effectiveness.
1. What is Transformer Architecture?
The Transformer Architecture is a neural network architecture that revolutionized natural language processing by addressing some of the key limitations of previous models, such as Recurrent Neural Networks (RNNs) and Long Short-Term Memory (LSTM) networks. Introduced by researchers at Google in 2017, the transformer model is the backbone of ChatGPT, enabling it to process large amounts of text data efficiently and generate high-quality outputs.
Self-Attention Mechanism: The core innovation in the transformer model is the self-attention mechanism. Unlike traditional models that read text sequentially, self-attention allows the transformer to consider all words in a sentence simultaneously and compute the relevance or “attention” of each word relative to others. This mechanism enables the model to focus on specific words or phrases that are more important for understanding the context of a sentence. For example, in the phrase “The cat sat on the mat,” the model understands that “cat” is related to “sat,” and “mat” is the object, regardless of their position in the sentence.
Parallel Processing: Unlike RNNs that process input sequentially, the transformer architecture processes text in parallel. This parallelism allows the model to handle longer sentences and larger datasets more efficiently, reducing training time and improving performance. The ability to analyze multiple words at once is crucial for capturing the nuances of natural language and generating coherent responses.
Positional Encoding: Since transformers do not process input in a sequential order, they use a technique called positional encoding to keep track of the order of words in a sentence. Positional encoding involves adding a set of fixed values to the input embeddings that provide information about the relative positions of words. This helps the model understand the sequence in which words appear, which is vital for maintaining the structure and meaning of sentences.
2. The Algorithm of ChatGPT: How It Generates Responses
The algorithm used by ChatGPT combines the principles of the transformer architecture with advanced machine learning techniques to generate text. The key steps in this algorithm involve breaking down text into manageable components, analyzing these components to understand context, and producing meaningful responses based on learned patterns.
Tokenization and Input Representation: Before processing, the input text is broken down into smaller units called tokens. Tokens can be whole words, sub-words, or even individual characters, depending on the context. ChatGPT uses a method called Byte Pair Encoding (BPE) to tokenize text, which allows it to handle complex words and rare vocabulary more effectively by breaking them into sub-components. Each token is then converted into a numerical representation or embedding that the model can process.
Multi-Head Attention: The self-attention mechanism is further enhanced through a technique called multi-head attention. This method allows the model to focus on different parts of the input simultaneously, providing multiple perspectives on the context. For instance, in a sentence, one attention head might focus on the subject, while another focuses on the verb or object, capturing different syntactic and semantic relationships. Multi-head attention improves the model’s ability to generate nuanced and context-aware responses.
Feedforward Neural Network Layers: After the attention layers, the tokens pass through several feedforward neural network layers. These layers consist of multiple linear transformations followed by non-linear activation functions that help the model learn complex patterns in the data. The output from these layers is combined with the attention results to produce a representation that encapsulates both contextual and syntactic information about the input text.
Layer Normalization and Residual Connections: To stabilize training and improve performance, the transformer architecture incorporates layer normalization and residual connections. Layer normalization ensures that the input to each layer has a stable distribution, which speeds up training and improves generalization. Residual connections allow information to bypass certain layers, reducing the risk of vanishing gradients during backpropagation and allowing the model to learn more effectively from deeper layers.
Output Generation Using Decoding Layers: After processing the input through attention and feedforward layers, the model generates the output token by token using decoding layers. The output is produced based on a probability distribution over the vocabulary, where the model predicts the most likely next word given the context. This process is repeated iteratively until a complete response is generated.
Temperature and Sampling: ChatGPT uses a parameter called temperature to control the randomness of the output. A lower temperature value results in more deterministic and conservative responses, while a higher temperature encourages more diverse and creative outputs. Sampling is another technique used to add variability to the responses by selecting words based on their probability distribution rather than always choosing the most likely word.
3. Training the Transformer Model: Learning from Data
The development of ChatGPT involves a complex training process where the transformer model learns from vast amounts of text data. This process includes both unsupervised learning during the pre-training phase and supervised fine-tuning to refine the model’s outputs.
Pre-Training with Unsupervised Learning: In the pre-training phase, ChatGPT is trained on a massive corpus of text data from books, articles, websites, and other sources. This phase uses unsupervised learning, where the model learns to predict the next word in a sequence based on the context provided by preceding words. For example, given the input "The sun rises in the," the model learns to predict "east" based on patterns observed in the data. This approach allows the model to learn language patterns, grammar, and factual information without any explicit labeling of the data.
Fine-Tuning with Supervised Learning: After pre-training, the model undergoes a fine-tuning phase using supervised learning. Here, human trainers provide specific prompts and ideal responses to guide the model’s behavior. The model learns from these examples to generate more accurate, contextually relevant responses. Additionally, Reinforcement Learning from Human Feedback (RLHF) is used to further refine the model’s outputs based on human feedback, where responses are ranked, and the model is trained to favor higher-ranked outputs.
4. Advantages of the Transformer Architecture in ChatGPT
The transformer architecture offers several advantages that make ChatGPT highly effective for natural language processing tasks:
Efficiency and Scalability: The transformer model’s ability to process input in parallel makes it more efficient and scalable than traditional RNNs or LSTMs. This efficiency is particularly important for training on large datasets and generating responses quickly in real-time applications.
Context Awareness and Coherence: The self-attention mechanism and multi-head attention in the transformer architecture enable ChatGPT to maintain context and coherence across long passages of text. This makes it capable of understanding complex queries, handling follow-up questions, and generating nuanced responses.
Flexibility and Adaptability: The transformer architecture is highly flexible, allowing it to adapt to various tasks, such as text summarization, translation, and question-answering. This versatility makes ChatGPT suitable for a wide range of applications, from customer support to creative writing.
Improved Generalization: The use of large-scale data during pre-training, combined with fine-tuning, enables the transformer model to generalize well across different topics, styles, and contexts. This broad understanding allows ChatGPT to respond accurately to a wide variety of queries, enhancing its utility and effectiveness.
Reinforcement Learning from Human Feedback (RLHF)
Reinforcement Learning from Human Feedback (RLHF) is a key component in the development of ChatGPT that helps align the AI model’s behavior with human values and preferences. RLHF is a unique method that uses human evaluators to guide the model in producing more accurate, contextually appropriate, and useful responses.
What is RLHF?
Reinforcement Learning from Human Feedback (RLHF) is a technique where the model is fine-tuned using feedback provided by human evaluators. After the initial training phase, where ChatGPT is exposed to vast amounts of text data, RLHF helps refine the model further by incorporating human judgment into its learning process.
Human Evaluators' Role: In RLHF, human evaluators interact with the AI, assess its responses, and provide feedback on their quality. They rank the outputs based on several criteria, such as relevance, accuracy, coherence, and appropriateness. This feedback is then used to train a Reward Model, which guides the AI in selecting the best possible responses during future interactions.
Iterative Improvement: RLHF involves multiple rounds of interaction between the model and human evaluators. Each round provides new feedback, allowing the model to continuously improve and align more closely with human expectations. This iterative process is essential for reducing biases, enhancing the quality of the responses, and ensuring that the AI behaves in a way that aligns with ethical and practical standards.
Supervised Fine-Tuning (SFT)
Supervised Fine-Tuning (SFT) is another critical step in training ChatGPT. It involves refining the model's behavior by exposing it to specific examples of inputs and outputs, which are curated by human trainers.
How Supervised Fine-Tuning Works: During the SFT phase, human trainers create a dataset of prompts and corresponding ideal responses. These prompts can cover a wide range of topics, questions, and conversational scenarios. The model is then trained to replicate these responses, learning from the examples provided by the human trainers. This step ensures that the model produces answers that are not only accurate but also contextually appropriate.
Role in Aligning with Human Intent: Supervised fine-tuning plays a crucial role in teaching the model how to respond correctly to different types of queries. It helps the model understand the nuances of human language, such as tone, style, and context. For instance, a prompt asking for a formal response versus a casual one would be handled differently after supervised fine-tuning. This process ensures that ChatGPT can handle a wide variety of requests in a manner that aligns with human expectations.
Creating the Initial Dataset: In the initial phase of SFT, human contractors generate a substantial number of input-output pairs. For example, OpenAI used about 13,000 pairs to kickstart the fine-tuning of GPT-3. These pairs include diverse examples that cover different conversational styles, queries, and domains. The dataset is then fed into the model, enabling it to learn from these high-quality examples and improve its performance.
Reward Model
The Reward Model is a key mechanism used in the RLHF process to evaluate and improve the quality of ChatGPT's responses. It is developed based on feedback from human evaluators, which helps the model prioritize responses that are more likely to be useful, accurate, and contextually appropriate.
How the Reward Model Works: In practice, multiple responses generated by ChatGPT for the same input prompt are presented to human evaluators. The evaluators rank these responses from the most to the least effective based on criteria like relevance, clarity, and correctness. The ranked responses are then used to train a reward model that assigns a score or "reward" to each output.
Training the Model: The reward model is trained using a large number of human-generated rankings, which creates a dataset that represents what constitutes a good response. This model then guides ChatGPT during future interactions, helping it to generate responses that are more likely to align with human preferences.
Purpose of the Reward Model: The reward model is designed to maximize the probability of generating high-quality responses. By using feedback to optimize its outputs, ChatGPT becomes more reliable and effective in handling diverse prompts. This mechanism ensures that the AI continuously learns from human feedback, adapting to different contexts and improving its performance over time.
Reinforcement Learning
Reinforcement Learning is a critical aspect of training ChatGPT that involves using the reward model to optimize the AI’s behavior. It builds upon the foundations set by supervised fine-tuning and the reward model to create a more dynamic and adaptable AI system.
How Reinforcement Learning Works in ChatGPT: In the context of ChatGPT, reinforcement learning involves taking a prompt, generating a response, and then receiving a "reward" based on the feedback from the reward model. This feedback is used to adjust the AI's parameters, refining its responses over time. The goal is to maximize the cumulative reward, which translates into generating more accurate and contextually appropriate outputs.
Proximal Policy Optimization (PPO): To implement reinforcement learning effectively, OpenAI uses a specific algorithm called Proximal Policy Optimization (PPO). PPO is a type of reinforcement learning technique that helps strike a balance between exploration (trying out new responses) and exploitation (using the best-known responses). It adjusts the AI’s policy—its strategy for generating responses—based on the feedback from the reward model, ensuring that the AI does not become overly reliant on any single type of response.
Optimization and Overfitting Prevention: PPO prevents over-optimization by using regularization techniques, which avoid the AI model from becoming too specialized or narrow in its responses. This ensures that ChatGPT remains flexible and capable of generating diverse outputs across different domains and types of queries. The use of reinforcement learning, therefore, enhances the model’s ability to generalize from its training data, making it more robust and versatile.
Iterative Learning Process: Reinforcement learning is an iterative process that involves multiple rounds of generating responses, receiving feedback, and adjusting the model’s parameters. Each iteration improves the model’s ability to understand human intent and produce relevant responses. This continuous learning loop is key to developing an AI that can interact naturally and effectively in diverse situations.
Natural Language Processing (NLP)
Natural Language Processing (NLP) is at the heart of how ChatGPT works. It is a branch of artificial intelligence that focuses on enabling computers to understand, interpret, and generate human language in a way that is both meaningful and useful. By leveraging advanced NLP techniques, ChatGPT can process vast amounts of text, understand context, and produce coherent and contextually relevant responses. Here, we explore how NLP powers ChatGPT, breaking down the specific components and methodologies that make this AI model so effective.
1. What is Natural Language Processing (NLP)?
Natural Language Processing (NLP) refers to a set of computational techniques used to analyze and generate human language. NLP enables machines to interpret text or spoken language, understand context, and perform tasks such as translation, sentiment analysis, and dialogue generation. In the case of ChatGPT, NLP is critical for converting raw text inputs into structured data that the model can process, and for transforming the model’s output into fluent and human-like responses.
Understanding Human Language: At its core, NLP involves understanding the structure and meaning of human language. This includes recognizing the grammar and syntax of sentences, identifying the meaning of words based on context, and understanding the sentiment or intent behind a particular phrase. NLP allows ChatGPT to parse user input, break down sentences into their constituent parts, and identify key entities, topics, and relationships.
Generating Human-Like Responses: Beyond understanding, NLP also enables the generation of text that is grammatically correct and contextually appropriate. This is achieved through a combination of machine learning algorithms, statistical models, and linguistic rules that guide the AI in producing coherent and relevant responses.
2. How Does ChatGPT Use NLP to Generate Responses?
ChatGPT utilizes a range of NLP techniques to analyze and generate text. The model processes input in several stages, each leveraging different aspects of NLP to ensure that the output is accurate, relevant, and contextually appropriate.
Tokenization: The first step in the NLP process is tokenization, where the input text is broken down into smaller units called tokens. Tokens can be words, parts of words, or even individual characters. ChatGPT uses Byte Pair Encoding (BPE) to split words into sub-word units, allowing it to handle complex or rare vocabulary more effectively. Tokenization enables the model to analyze the input text at a granular level, helping it understand the meaning of each token in context.
Contextual Understanding: One of the key strengths of ChatGPT lies in its ability to maintain context awareness across long conversations. The model uses NLP techniques to retain information from earlier parts of a conversation and apply that context to generate more relevant responses later. For instance, if a user asks a follow-up question, ChatGPT can recall the context of the initial query and provide a coherent answer that aligns with the previous interaction.
Semantic Analysis: ChatGPT uses semantic analysis to understand the meaning behind words and phrases. This involves interpreting the relationships between words and recognizing their meanings based on the surrounding context. For example, in the sentence "The bank was flooded after the heavy rain," ChatGPT understands that "bank" refers to the edge of a river, not a financial institution, based on the context provided by the rest of the sentence.
Syntactic Parsing: Another critical NLP technique used by ChatGPT is syntactic parsing. This process involves breaking down sentences into their grammatical components, such as subjects, verbs, objects, and modifiers. Syntactic parsing helps ChatGPT understand the structure of a sentence, which is essential for generating grammatically correct responses and identifying the relationships between different parts of a sentence.
3. The Role of NLP in Understanding User Intent
A significant aspect of how ChatGPT works is its ability to discern user intent, which is vital for generating meaningful responses.
Intent Recognition: ChatGPT employs various NLP models to identify the user’s intent behind a given query. Intent recognition involves understanding the underlying purpose of a sentence, such as whether the user is asking a question, making a request, or expressing an opinion. For example, the input "Can you explain how photosynthesis works?" is recognized as a request for information, prompting the model to generate an educational response.
Handling Ambiguity: Human language is often ambiguous, with words having multiple meanings depending on the context. NLP allows ChatGPT to handle such ambiguities by analyzing the surrounding text to determine the most likely interpretation. For example, the word "bat" could refer to an animal or a piece of sports equipment; NLP helps the model infer the correct meaning based on context.
Sentiment Analysis: ChatGPT uses sentiment analysis to gauge the emotional tone of user input. This can help the model adjust its responses to match the sentiment expressed by the user. For example, if the input conveys frustration or anger, ChatGPT might respond in a more empathetic and supportive manner.
4. Techniques That Enhance ChatGPT's NLP Capabilities
ChatGPT's NLP capabilities are enhanced by several advanced techniques, allowing it to generate high-quality, human-like text.
Transformer Architecture: At the core of ChatGPT’s NLP capabilities is the Transformer Architecture, which utilizes a self-attention mechanism to analyze and generate text. The self-attention mechanism allows the model to focus on different parts of the input text simultaneously, enabling it to understand context more accurately. This capability is critical for maintaining the coherence and relevance of responses, even in complex conversations.
Multi-Head Attention: ChatGPT also uses multi-head attention, an extension of the self-attention mechanism, which enables the model to focus on different parts of the input simultaneously and interpret them from multiple perspectives. Each "head" in the multi-head attention mechanism processes a different aspect of the input, such as syntax, semantics, or specific entities, which improves the model’s ability to understand nuanced queries.
Transfer Learning: ChatGPT leverages transfer learning to enhance its NLP capabilities. During the pre-training phase, the model learns from a vast amount of text data across various domains. This knowledge is then transferred to more specific tasks during the fine-tuning phase. Transfer learning enables ChatGPT to generalize its understanding of language and apply it to different contexts, making it highly versatile and adaptable.
Reinforcement Learning from Human Feedback (RLHF): RLHF is another technique that helps improve ChatGPT's NLP performance. By learning from human-provided feedback, the model refines its responses to align more closely with human expectations. This iterative learning process ensures that the model continually improves its ability to understand and generate text that is both accurate and contextually appropriate.
5. Applications of NLP in ChatGPT
The NLP capabilities of ChatGPT make it a powerful tool for a wide range of applications:
Customer Support: ChatGPT can handle customer inquiries by understanding the intent behind questions and providing accurate, contextually relevant responses. Its ability to maintain context across multiple exchanges makes it ideal for extended customer service interactions.
Content Creation: With its NLP-driven understanding of language, ChatGPT can assist in generating content such as articles, blog posts, social media updates, and more. It can adapt to different writing styles and tones, making it versatile for various content needs.
Education and Training: ChatGPT can serve as a virtual tutor, helping students understand complex topics by generating explanatory content in simple terms. Its ability to adjust explanations based on user input makes it an effective educational tool.
Programming Assistance: Developers use ChatGPT to understand programming concepts, debug code, and generate code snippets. The model's NLP capabilities allow it to interpret programming-related queries accurately and provide detailed explanations or solutions.
6. Future Directions for NLP in ChatGPT
The future of NLP in ChatGPT involves continuous improvements to its understanding and generation capabilities:
Enhanced Context Management: Future versions of ChatGPT are likely to improve in retaining and managing context over longer conversations, ensuring that responses remain relevant and coherent even after multiple exchanges.
Multimodal NLP: As ChatGPT evolves, it is expected to handle multimodal inputs, such as text, images, and audio, more effectively. This will enhance its ability to interpret and respond to more complex queries that involve multiple types of data.
Greater Cultural and Linguistic Sensitivity: By integrating more diverse datasets and refining its NLP algorithms, ChatGPT will become more adept at understanding cultural nuances, dialects, and languages, broadening its applicability across different regions and contexts.
What Can ChatGPT Do?
ChatGPT, developed by OpenAI, is a versatile AI tool capable of performing a wide range of tasks that involve understanding and generating human language. Built on the advanced GPT (Generative Pre-trained Transformer) architecture, ChatGPT leverages sophisticated Natural Language Processing (NLP) techniques to produce accurate, context-aware responses across various applications. Whether it's answering complex questions, generating tailored messages and emails, or engaging in dynamic conversations, ChatGPT demonstrates a broad utility that makes it a valuable tool for individuals and businesses alike.
Answer Questions
One of the primary capabilities of ChatGPT is its ability to answer questions accurately and contextually. By understanding the nuances of human language and interpreting context, ChatGPT can provide detailed and informative answers to a wide range of questions, making it a powerful tool for both casual users and professionals.
Understanding Context and Relevance: ChatGPT is trained on a diverse range of text data, which allows it to understand the context behind questions. When a user poses a query, ChatGPT processes the input using its NLP algorithms to determine the intent, extract relevant information, and provide a coherent response. For example, if asked, "What are the benefits of renewable energy?" ChatGPT will analyze the keywords and context to generate a comprehensive answer outlining the advantages of renewable energy sources.
Handling Complex Queries: ChatGPT is not limited to simple questions; it can handle complex, multi-part queries that require a deep understanding of various subjects. For instance, when faced with a question like "Explain the differences between classical and quantum physics," ChatGPT draws from its extensive training data to provide a detailed comparison, highlighting key concepts and distinctions between the two fields. This ability makes it useful for educational purposes, research, and technical support.
Providing Real-Time Information: ChatGPT can provide up-to-date information on a wide range of topics. Although the model itself is static and does not learn from user inputs in real time, it can be integrated with external data sources or APIs to offer real-time data, such as weather forecasts, news updates, or stock market trends. This feature is particularly useful for businesses that require immediate and accurate information to make decisions.
Multilingual Support: ChatGPT’s ability to understand and respond in multiple languages makes it a versatile tool for users across the globe. It can translate text, answer questions in different languages, and provide explanations or clarifications in a language of the user's choice. This multilingual capability expands its reach and usability, catering to diverse linguistic needs.
Adapting to User Feedback: Through Reinforcement Learning from Human Feedback (RLHF), ChatGPT continuously improves its ability to understand and respond to questions accurately. By learning from human feedback, the model adapts to generate more relevant and contextually appropriate answers, enhancing the overall user experience.
Write Tailored Messages and Emails
ChatGPT is also highly effective in generating customized messages and emails. It can draft a wide range of written content, from professional correspondence to personalized messages, adapting its tone, style, and content to suit different contexts and audiences.
Generating Personalized Emails: One of the most practical uses of ChatGPT is to draft emails tailored to specific recipients or situations. For example, if a user needs to send a follow-up email after a business meeting, ChatGPT can generate a concise and professional message that captures key discussion points and next steps. The model can adapt to different tones—whether formal or casual—depending on the context, ensuring that the email resonates with its intended audience.
Creating Engaging Marketing Content: ChatGPT can be used to write compelling marketing emails designed to capture the reader's attention and drive action. By understanding the principles of persuasive writing and user intent, ChatGPT can generate email copy that promotes products, services, or events effectively. It can incorporate calls to action, emphasize key benefits, and adapt its style to match the brand's voice.
Customizing Messages for Different Scenarios: Whether it's writing a thank-you note, a condolence message, or an invitation, ChatGPT can generate tailored messages for a wide range of personal and professional scenarios. It uses its understanding of language and context to ensure that the message is appropriate for the situation, capturing the right tone and sentiment.
Streamlining Workflow and Saving Time: By automating the process of drafting messages and emails, ChatGPT helps users save time and streamline their workflow. This is especially valuable for professionals who need to handle large volumes of correspondence daily. Instead of spending time crafting each message from scratch, users can rely on ChatGPT to generate drafts that can be quickly reviewed and sent.
Enhancing Creativity and Overcoming Writer’s Block: For individuals who struggle with writer’s block or need inspiration, ChatGPT can provide creative suggestions and ideas for writing. It can help brainstorm topics, suggest alternative phrases, or even rewrite content in different styles. This makes it a valuable tool for writers, marketers, and content creators looking for fresh ideas and perspectives.
Check Code for Errors
One of the key capabilities of ChatGPT is its ability to check code for errors. This function is particularly valuable for developers and programmers who seek to quickly identify and resolve issues in their code. Using advanced Natural Language Processing (NLP) and machine learning techniques, ChatGPT can analyze code snippets, detect potential bugs or errors, and suggest corrections. This feature demonstrates how ChatGPT works technically to support coding tasks and enhance programming efficiency.
How ChatGPT Checks Code for Errors
Understanding Programming Languages: ChatGPT is trained on a vast dataset that includes a wide range of programming languages, such as Python, JavaScript, C++, Java, HTML, and SQL. This training allows the model to understand syntax, common functions, libraries, and coding conventions specific to each language. When a user submits a code snippet, ChatGPT uses its understanding of these languages to analyze the structure, identify potential issues, and generate recommendations for correction.
Error Detection: When checking for errors, ChatGPT examines the syntax and logic of the code. It looks for common programming mistakes, such as missing semicolons, incorrect function calls, unmatched brackets, or improper indentation. For example, if a user submits a Python code snippet with a missing colon in a conditional statement (if x == 5), ChatGPT can detect the missing colon (:) and suggest adding it (if x == 5:).
Debugging and Optimization: Beyond syntax errors, ChatGPT can also assist with debugging by identifying logical errors that might not immediately trigger syntax warnings but could cause the program to behave unexpectedly. For instance, if a loop is set up to iterate incorrectly or a variable is used before initialization, ChatGPT can highlight these issues and suggest corrections. Additionally, it can offer optimization tips to improve code performance, such as suggesting more efficient algorithms or refactoring redundant code.
Code Explanation and Clarification: In addition to detecting errors, ChatGPT can provide explanations for why a certain part of the code may be incorrect and how to fix it. This makes it an excellent tool for learning and understanding programming concepts, especially for beginners. For example, if a user is unsure why their function is returning an unexpected result, ChatGPT can analyze the function and explain which part of the logic might be causing the problem.
Integration with Development Environments: ChatGPT can be integrated with development environments and coding platforms through APIs, providing real-time code review and error detection. This integration allows developers to catch mistakes early in the development process, reducing debugging time and improving code quality.
Examples of Code Checking with ChatGPT
Syntax Checking: If a user submits a JavaScript code snippet with a missing curly brace, ChatGPT can quickly identify the syntax error and suggest adding the necessary curly brace to correct the code.
Logical Errors: In a Python program, if a user has written a loop that runs indefinitely due to a faulty condition, ChatGPT can detect the logical error and provide suggestions on how to adjust the loop condition to prevent infinite execution.
Optimization Suggestions: If a user provides a SQL query that is functional but not optimized, ChatGPT can recommend more efficient ways to write the query, such as using indexed columns or avoiding unnecessary subqueries.
Multimodality in ChatGPT: Images, Audio, and More
Multimodality in ChatGPT refers to its capability to process and generate responses based on multiple types of inputs, such as text, images, audio, and potentially even video. This represents a significant advancement in how ChatGPT works, broadening its applications beyond traditional text-based interactions to include diverse types of data and sensory inputs.
1. What is Multimodality?
Multimodality refers to the ability of an AI model to understand and respond to different forms of input data—such as text, images, and audio—simultaneously or interchangeably. This capability allows the AI to provide richer, more context-aware responses and perform tasks that go beyond text comprehension. In the case of ChatGPT, multimodal capabilities enable it to handle a broader range of applications, enhancing its usefulness in various domains.
Text Processing: At its core, ChatGPT is optimized for processing and generating text. It uses NLP techniques to analyze input text, understand the context, and produce coherent responses. The addition of multimodality enhances its text-based capabilities by incorporating other types of data.
Image Recognition and Analysis: With multimodal capabilities, ChatGPT can process and interpret visual data. For example, it can analyze images, recognize objects, identify scenes, or even describe the contents of a picture. This capability makes ChatGPT useful for applications such as image-based customer support, where a user might upload a photo to describe a product issue, or in educational settings, where it can be used to analyze diagrams or visual content.
Audio Interpretation: ChatGPT’s multimodal capabilities also extend to audio data. It can interpret spoken language, convert speech to text, and generate responses based on the audio input. This functionality is valuable for creating voice-activated assistants, real-time translation services, or accessibility tools for visually impaired users. For example, in a customer service scenario, ChatGPT could listen to a user's query via audio input, convert it to text, analyze the context, and provide an appropriate response.
2. How Does Multimodality Work in ChatGPT?
Integration of Multiple Data Types: Multimodality in ChatGPT involves the integration of various data types into a unified model that can handle them concurrently. This integration is achieved through advanced machine learning techniques, such as deep neural networks and transformer models, which are capable of processing different types of data in parallel.
Cross-Modal Learning: ChatGPT utilizes cross-modal learning, where the model learns to correlate information from different modalities. For instance, if an input consists of both an image and a text description, ChatGPT can use information from both sources to provide a more comprehensive response. This capability enhances its ability to understand complex queries that involve multiple types of data.
Enhanced Context Awareness: By combining different modalities, ChatGPT can provide richer and more accurate responses. For example, in medical applications, ChatGPT could analyze an X-ray image while simultaneously reading a doctor’s notes to provide a more informed diagnosis or recommendation.
3. Applications of Multimodality in ChatGPT
The multimodal capabilities of ChatGPT open up new possibilities for its use in various fields:
Customer Service: Multimodal ChatGPT can enhance customer support by allowing users to upload images of products, parts, or issues they are experiencing, alongside a text description. The AI can then analyze both inputs to provide more accurate troubleshooting or assistance.
Education and Training: In educational settings, ChatGPT can help interpret visual aids such as charts, graphs, or diagrams while also explaining complex concepts in text. This capability makes it a valuable tool for interactive learning.
Healthcare: In healthcare, ChatGPT's multimodal capabilities can assist doctors and medical professionals by analyzing patient images (like X-rays or MRIs) and synthesizing the information with patient records or medical literature to support diagnostic decisions.
Accessibility Tools: ChatGPT can serve as a robust accessibility tool by converting text to speech or interpreting images for visually impaired users. It can also transcribe spoken content for those with hearing difficulties, bridging communication gaps.
4. Future Directions for Multimodality in ChatGPT
As ChatGPT continues to evolve, its multimodal capabilities are expected to expand further:
Improved Visual Recognition: Future iterations of ChatGPT may include enhanced visual recognition capabilities, allowing it to handle more complex image analysis tasks, such as identifying emotions from facial expressions or recognizing objects in cluttered scenes.
Advanced Audio Processing: ChatGPT's audio capabilities may also be refined to support real-time translation, sentiment analysis from voice tone, and even more sophisticated voice-to-text conversions.
Integration with Virtual and Augmented Reality (VR/AR): ChatGPT could potentially integrate with VR and AR platforms, providing context-aware assistance in immersive environments. This could be useful in gaming, training simulations, or virtual customer support.
Applications of ChatGPT
ChatGPT, powered by OpenAI's Generative Pre-trained Transformer (GPT) technology, has rapidly become a versatile tool across various sectors, demonstrating its ability to revolutionize both professional and personal tasks. By understanding how ChatGPT works, including its underlying architecture and training processes, we can better appreciate its wide range of applications. Below is a detailed exploration of how ChatGPT is being used across different fields.
1. Content Creation and Writing Assistance
ChatGPT is extensively used in content creation, providing significant value to both amateur writers and professional content creators. It can generate creative writing prompts, draft articles, compose engaging social media content, and even craft emails. By leveraging its deep learning capabilities, ChatGPT can produce text that mimics human language patterns, making it an ideal tool for writing assistance. Its ability to understand context and generate coherent, relevant content has made it indispensable for bloggers, journalists, and digital marketers looking to streamline their content creation processes.
2. Programming and Technical Support
In the realm of programming, ChatGPT is a valuable resource for developers. It assists in understanding complex programming concepts, debugging code, and finding solutions to coding problems. Its natural language processing (NLP) capabilities enable it to explain code, suggest improvements, and even generate code snippets based on user prompts. This application is particularly beneficial for both novice programmers and seasoned developers who need quick, reliable technical support.
3. Educational Support
ChatGPT is also a powerful educational tool. It helps students and educators by providing explanations on complex topics, assisting with homework, and even generating study materials. The AI's ability to break down complicated subjects into simpler, more understandable parts makes it an excellent tutor for a wide range of subjects, from mathematics and science to literature and history. Furthermore, its interactive nature allows students to engage in a dialogue, asking follow-up questions and receiving tailored responses that cater to their specific learning needs.
4. Professional Development
For individuals seeking to advance their careers, ChatGPT offers a range of professional development tools. It can assist with resume writing, draft cover letters, and even provide mock interview questions. By simulating real-world scenarios, ChatGPT helps users prepare for job interviews, offering feedback on their answers and suggesting improvements. This application extends to various professional tasks, such as writing business emails, creating presentations, and drafting reports, making it a comprehensive tool for career advancement.
5. Customer Service and Virtual Assistance
Many businesses are integrating ChatGPT into their customer service systems. Its ability to understand and respond to customer inquiries in real-time makes it an effective virtual assistant. Unlike traditional chatbots that rely on predefined scripts, ChatGPT can engage in meaningful conversations, providing personalized responses based on the context of the query. This adaptability improves customer satisfaction and streamlines support processes, making ChatGPT a valuable asset for businesses looking to enhance their customer service offerings.
6. Entertainment and Leisure
Beyond its professional applications, ChatGPT serves as a source of entertainment. Users can engage with the AI for casual conversations, generate jokes, create stories, or even play text-based games. Its ability to simulate human-like interactions makes it an enjoyable tool for those looking to unwind or explore creative ideas. Additionally, its capabilities in generating creative content, such as poems or scripts, provide users with endless possibilities for leisure activities.
7. Healthcare and Medical Support
In the healthcare sector, ChatGPT is being explored for its potential to assist with medical diagnoses, patient education, and mental health support. While it is not a substitute for professional medical advice, ChatGPT can provide preliminary information on symptoms, suggest possible conditions, and offer advice on when to seek medical attention. For mental health, it can engage in conversations that help users articulate their feelings and provide general advice on managing stress and anxiety, acting as a preliminary support tool before professional intervention.
8. Research and Knowledge Expansion
Researchers are leveraging ChatGPT to explore new ideas, summarize research papers, and generate hypotheses. Its ability to process vast amounts of information and generate coherent summaries makes it an invaluable tool for academic and scientific research. By automating the synthesis of information, ChatGPT helps researchers focus on analysis and experimentation, thus accelerating the pace of discovery and innovation.
9. Legal and Compliance Assistance
Legal professionals are beginning to use ChatGPT for drafting documents, reviewing contracts, and ensuring compliance with regulations. The AI's ability to understand legal language and generate text that aligns with specific legal requirements makes it a useful tool for lawyers and compliance officers. While it cannot replace the nuanced understanding of a human legal expert, ChatGPT can assist in routine tasks, thus freeing up time for more complex legal work.
10. Marketing and Sales
In marketing, ChatGPT is utilized to create personalized marketing campaigns, draft product descriptions, and generate sales emails. Its ability to analyze customer data and generate targeted content helps businesses engage with their audience more effectively. In sales, ChatGPT can assist in lead generation by responding to inquiries and providing information on products and services, thereby increasing the efficiency of sales teams.
What’s the Difference Between ChatGPT and a Search Engine?
Understanding the difference between ChatGPT and a search engine is crucial, as these technologies serve distinct purposes and operate based on different underlying mechanisms. Although both can provide information in response to user queries, their functionalities, methods of operation, and ideal use cases diverge significantly.
1. Purpose and Functionality
Search Engines: Search engines like Google, Bing, and Yahoo are designed to retrieve and present relevant web pages from an indexed database of the internet based on a user's query. They primarily function as tools for information retrieval, pulling from a vast repository of web pages, documents, images, and videos. When you enter a query, the search engine analyzes it, searches its index, and presents a list of links that are most relevant to the keywords in your query. The results are typically ranked by relevance, popularity, and other algorithmic factors, providing users with a wide array of options to choose from.
ChatGPT: On the other hand, ChatGPT, developed by OpenAI, is a conversational AI chatbot that generates human-like text responses to user prompts based on a large language model. Instead of retrieving existing content from the web, ChatGPT generates text in real-time by predicting the most appropriate response to a given input. It’s designed to simulate conversation, answer questions, generate content, and assist with tasks like coding or writing. ChatGPT does not simply retrieve and display information but creates new text that aligns with the context and specifics of the user's query.
2. How They Work: Data Sources and Processing
Search Engines: Search engines use a process called crawling and indexing to collect data from the web. This involves automated bots called spiders that scour the internet, following links, and indexing the content of web pages. Once this data is indexed, search engines apply complex algorithms to rank these pages based on relevance to the user's query. The results are drawn from this indexed data, which is continually updated to include new or modified web pages.
ChatGPT: ChatGPT, on the other hand, operates differently. It is based on the Generative Pre-trained Transformer (GPT) architecture, a type of machine learning model. ChatGPT was trained on a massive dataset comprising text from books, articles, websites, and other written content, allowing it to understand and generate human-like responses. Unlike search engines, ChatGPT doesn’t retrieve data from a database at the moment of query. Instead, it uses its training to generate responses based on patterns and relationships it has learned from the data it was trained on. This means that while ChatGPT can provide information, it does so by generating new text rather than pulling direct answers from a source.
3. Response Generation and Output
Search Engines: When you input a query into a search engine, the result is a list of web pages that match your query. The engine provides a summary (often called a snippet) for each link, helping users determine which link might be most relevant. These summaries are directly pulled from the web pages themselves, and users must click through to read the full content.
ChatGPT: In contrast, when you input a query into ChatGPT, the response is generated in full within the chat interface. The AI processes the input, uses its understanding from the training data, and generates a coherent, contextually appropriate response. ChatGPT’s responses are not direct quotations or references to specific documents but are newly synthesized text that aims to fulfill the user’s request in a conversational manner.
4. Contextual Understanding and Interaction
Search Engines: Search engines are primarily transactional—they take a query, provide results, and the interaction ends unless a new search is initiated. The interaction is query-driven and doesn’t retain context from previous searches. Each query is treated independently, and search engines do not adapt based on previous interactions within the same session.
ChatGPT: ChatGPT, however, is designed for ongoing conversation and can maintain context across multiple interactions within a session. For example, if you ask ChatGPT about a specific topic and then ask a follow-up question, it will remember the context of the initial conversation. This allows for a more fluid and natural interaction, making it possible to have extended, nuanced conversations where the AI adapts to the flow of dialogue.
5. Use Cases and Applications
Search Engines: The primary use case for search engines is information retrieval. Whether you need to find the latest news, research a topic, locate a business, or discover new websites, search engines excel at bringing together resources from across the web. They are particularly effective when users need to browse multiple sources of information or seek out content from specific sites.
ChatGPT: ChatGPT is best suited for tasks that require generating new content or engaging in a back-and-forth conversation. It can assist with writing essays, drafting emails, creating dialogue, or brainstorming ideas. Its ability to maintain conversation context makes it ideal for customer service, tutoring, and other scenarios where ongoing dialogue is needed. ChatGPT is also useful for creative endeavors, such as generating stories, poems, or scripts, where it can simulate human-like creativity and coherence.
6. Accuracy and Reliability
Search Engines: Since search engines direct users to existing web pages, the accuracy of the information depends on the credibility of the source. Users can cross-reference multiple sources, but search engines do not generate the content themselves—they merely provide access to it. This means that the reliability of information largely depends on the user’s ability to discern credible sources.
ChatGPT: While ChatGPT generates responses based on its training data, it can sometimes produce inaccurate or misleading information because it doesn’t have real-time access to databases or the internet. Its responses are probabilistic, meaning it generates text that it predicts to be appropriate rather than verifying facts. Users should be cautious and cross-check information provided by ChatGPT, especially when accuracy is critical.
What is the ChatGPT API?
The ChatGPT API, developed by OpenAI, is a powerful tool that allows developers to integrate the capabilities of ChatGPT into various applications, platforms, and services. The API essentially provides access to the underlying GPT (Generative Pre-trained Transformer) models, enabling a wide range of functionalities that can be customized and extended to meet specific needs.
The ChatGPT API is built on the same GPT architecture that powers the ChatGPT web interface. It allows developers to harness the deep learning capabilities of GPT models, which are trained on vast datasets comprising text from books, articles, and websites. This API enables applications to generate human-like text responses, automate content creation, and engage in natural language conversations.
By utilizing the ChatGPT API, developers can create applications that interact with users in a conversational manner. This can include anything from customer service bots to virtual assistants that can understand and respond to complex queries. The API is designed to be flexible and scalable, making it suitable for a wide range of use cases across different industries.
The ChatGPT API functions by receiving input text (prompts) from the user, processing it through the GPT model, and generating a text-based response. The API handles all the complexity of running the model and allows developers to focus on building their applications.
When a user sends a request to the API, the input text is tokenized—broken down into smaller units called tokens. The GPT model then analyzes these tokens, using its vast network of parameters to predict and generate the most relevant response based on the input. The generated response is then sent back to the user through the API, allowing for seamless interaction.
The API is also equipped with advanced features like fine-tuning, where developers can customize the model’s behavior by training it on specific datasets. This is particularly useful for creating specialized applications that require domain-specific knowledge or a particular style of communication.
Start a Conversation with ChatGPT in Slack
Integrating ChatGPT into Slack can significantly enhance the way teams communicate and collaborate. By embedding the ChatGPT API into Slack, users can start conversations with the AI directly within their Slack channels, enabling real-time assistance and support.
1. How to Integrate ChatGPT with Slack
To start a conversation with ChatGPT in Slack, developers need to use the ChatGPT API to create a custom Slack bot. This bot can be configured to respond to specific triggers or commands within a Slack workspace. For example, team members can ask the bot questions, request summaries of long threads, or even get assistance with drafting messages.
The integration process involves creating a Slack app, configuring it to connect with the ChatGPT API, and setting up the necessary authentication. Once integrated, the bot can listen to user inputs in Slack, send them to the ChatGPT API for processing, and return the AI-generated responses in the same channel.
2. Benefits of ChatGPT in Slack
Instant Responses: ChatGPT can provide instant responses to queries, helping teams find answers quickly without leaving the Slack interface.
Automated Support: It can serve as a first line of support, handling common questions and tasks, freeing up human team members to focus on more complex issues.
Enhanced Collaboration: By summarizing long discussions or extracting key points, ChatGPT helps keep everyone on the same page, improving team collaboration and productivity.
Create Email Copy with ChatGPT and Save as Drafts in Gmail
Using ChatGPT to draft email content can save time and improve the quality of communication, especially in professional settings. Integrating ChatGPT with Gmail allows users to automatically generate email drafts based on input prompts and save them directly in their Gmail account.
1. How the Integration Works
By leveraging the ChatGPT API, developers can create a system where users input a brief description of what they need in an email, and ChatGPT generates the full email text. This text can then be automatically saved as a draft in Gmail, ready for review and sending.
This integration typically involves setting up a workflow where user inputs are sent to the ChatGPT API. The API processes the input, generates the email content, and then uses Gmail’s API to save the generated text as a draft in the user’s account.
2. Use Cases for Email Generation
Sales and Marketing: Sales teams can use this integration to quickly generate personalized outreach emails, saving time and ensuring consistency in messaging.
Customer Support: Support teams can generate templated responses that are tailored to specific customer queries, improving efficiency and response times.
Internal Communication: Team members can use ChatGPT to draft internal memos, meeting summaries, or follow-up emails, ensuring clear and professional communication.
Generate Conversations in ChatGPT with New Emails in Gmail
A more advanced use of the ChatGPT API involves creating conversations based on new emails received in Gmail. This can be particularly useful for automating responses or generating insights from ongoing email threads.
1. Automating Conversations with Incoming Emails
When a new email is received in Gmail, an integrated system can trigger ChatGPT to analyze the content of the email and generate a response or summary. This response can either be sent automatically or saved as a draft for human review.
The integration typically works by using Gmail’s API to detect new emails, extracting the relevant content, and sending it to ChatGPT through the API. ChatGPT processes the email content, generates a response or summary, and then sends it back to Gmail, where it can be handled according to predefined rules.
2. Benefits of Email-Based Conversations with ChatGPT
Efficiency: Automating the generation of responses to emails can significantly reduce the time spent on routine communication tasks.
Consistency: ChatGPT can help maintain a consistent tone and style in responses, which is important for brand communication and customer relations.
Insight Generation: By analyzing email threads, ChatGPT can generate insights or key takeaways, helping users stay on top of ongoing conversations without manually reading through every message.
Future of ChatGPT and AI Automation
The future of ChatGPT and AI automation is set to be transformative, as advancements in artificial intelligence technology continue to accelerate. ChatGPT, developed by OpenAI, represents a powerful example of how AI can be used to understand and generate human-like text. The evolution of ChatGPT and its underlying technologies will pave the way for more sophisticated AI applications that can handle complex tasks across multiple domains, from customer service and content creation to healthcare and finance. This section explores the future of ChatGPT and AI automation, highlighting key areas of development and potential impacts.
1. Enhanced Natural Language Understanding and Generation
As AI technology continues to advance, future iterations of ChatGPT are expected to exhibit even more sophisticated natural language understanding and generation capabilities. This improvement will enhance the model’s ability to handle more nuanced and complex queries, making interactions more seamless and human-like.
Greater Context Awareness: Future versions of ChatGPT will likely have enhanced context management, enabling the model to maintain the context of conversations over longer exchanges. This will allow it to engage in more meaningful and coherent dialogues, understand complex instructions, and provide more accurate responses tailored to the specific needs of users.
Improved Language Fluency and Accuracy: By leveraging larger and more diverse datasets, ChatGPT can continue to improve its fluency and grammatical accuracy across multiple languages. This will help it better understand idiomatic expressions, cultural nuances, and diverse dialects, making it a more effective tool for global communication.
More Personalized Interactions: Future developments could enable ChatGPT to offer more personalized user experiences by learning from individual preferences and adapting its responses accordingly. While privacy and data security will remain top priorities, AI models may be designed to remember user preferences for specific tasks, such as preferred writing styles, tone, or even particular formatting, thereby making interactions more efficient and tailored.
2. Expansion of Multimodal Capabilities
One of the most exciting areas of growth for ChatGPT lies in the expansion of its multimodal capabilities. Future versions of ChatGPT are expected to handle not just text but also a wider range of inputs, such as images, audio, and even video, making the AI model more versatile and powerful.
Advanced Visual Understanding: Future iterations of ChatGPT could include more sophisticated visual recognition and interpretation capabilities, allowing it to analyze complex images, recognize objects, and provide detailed descriptions. For example, it could assist in medical imaging by analyzing X-rays or MRIs or help identify objects in photos for retail or security applications.
Enhanced Audio Processing: In the future, ChatGPT may be able to handle more advanced audio inputs, including real-time voice recognition and speech synthesis. This capability could support applications like voice-activated virtual assistants, real-time translation services, and transcription tools that can capture not only the text of a conversation but also the speaker’s tone, mood, and intent.
Integration with AR/VR Platforms: As augmented reality (AR) and virtual reality (VR) technologies continue to develop, ChatGPT could be integrated with AR/VR platforms to provide immersive, context-aware assistance. For example, in a VR training simulation, ChatGPT could act as an interactive tutor, providing real-time feedback and guidance.
3. Greater Automation Across Industries
The future of ChatGPT and AI automation is also closely tied to its ability to automate tasks across various industries, leading to increased efficiency, reduced costs, and enhanced productivity.
Customer Service and Support: ChatGPT’s ability to understand and generate natural language responses will make it a critical tool in customer service automation. Future versions of ChatGPT could handle more complex customer queries, provide personalized recommendations, and even predict customer needs based on historical interactions, reducing the need for human intervention and improving customer satisfaction.
Healthcare and Diagnostics: In healthcare, AI models like ChatGPT could assist medical professionals by automating routine tasks such as patient triage, medical record analysis, and appointment scheduling. With more advanced multimodal capabilities, ChatGPT could analyze medical images, interpret patient symptoms, and provide preliminary diagnoses, enabling faster and more accurate patient care.
Financial Services and Risk Management: In the financial sector, ChatGPT could be used to automate tasks such as customer onboarding, transaction monitoring, fraud detection, and risk assessment. Its ability to analyze large datasets and provide real-time insights could help financial institutions make more informed decisions and respond more quickly to market changes.
Content Creation and Marketing: As ChatGPT’s language generation capabilities improve, it could be increasingly used for content creation, including generating blog posts, social media updates, and marketing materials. By understanding audience preferences and brand voice, future versions of ChatGPT could produce more engaging and relevant content at scale, reducing the workload for marketing teams.
4. Integration with IoT and Smart Devices
The integration of ChatGPT with the Internet of Things (IoT) and smart devices is another promising area for future development. By combining NLP with IoT capabilities, ChatGPT could serve as a central hub for controlling and interacting with various smart devices.
Smart Home Automation: ChatGPT could be integrated with smart home systems to provide more intuitive control over appliances, lighting, heating, and security systems. For example, a user could issue complex voice commands like "Set the living room lights to 50% brightness and play my favorite playlist," and ChatGPT would be able to parse the command and execute it using connected devices.
Wearable Technology: ChatGPT could be embedded in wearable devices such as smartwatches or fitness trackers to provide real-time coaching, health monitoring, and personalized advice. This could enhance the user experience by providing seamless, context-aware assistance.
5. Ethical AI and Responsible Deployment
As ChatGPT and AI automation continue to evolve, it is critical to address ethical considerations and ensure responsible deployment. The future development of ChatGPT will involve continuous efforts to make the model safer, more transparent, and fairer.
Bias Mitigation: Future versions of ChatGPT will incorporate more robust bias mitigation techniques to ensure that the AI provides fair and unbiased responses. This will involve refining training data, improving the reward models, and increasing transparency around how the AI makes decisions.
Privacy and Data Security: As AI models become more integrated into everyday life, protecting user data will be of paramount importance. Future developments will likely focus on creating secure, privacy-preserving methods for data handling, ensuring that AI systems like ChatGPT do not inadvertently compromise personal information.
Human-AI Collaboration: The future of AI automation will also emphasize human-AI collaboration rather than replacement. ChatGPT will likely be designed to complement human skills, acting as a tool that enhances human capabilities rather than replacing them. This will create new opportunities for learning, innovation, and productivity across various sectors.
6. Continuous Learning and Adaptability
Looking forward, the ability of ChatGPT to continuously learn and adapt will be critical to its evolution and effectiveness. Future versions of the model may be designed to learn from real-time interactions while still respecting user privacy and ethical guidelines.
Adaptive Learning Models: Future iterations could incorporate mechanisms that allow ChatGPT to adapt its responses based on user feedback and interaction history, making the AI more responsive and attuned to specific user needs. This would enable more dynamic and personalized interactions.
Improved Learning from Human Feedback: Enhanced methods for Reinforcement Learning from Human Feedback (RLHF) will allow ChatGPT to refine its outputs more effectively. This continuous improvement process will help the model better align with human values and expectations, resulting in more accurate and useful outputs.
Conclusion
ChatGPT stands as a transformative force in the realm of AI, redefining how machines understand and interact with human language. Its ability to generate accurate, contextually relevant, and human-like responses makes it a powerful tool for automation and innovation. As it continues to develop and integrate with other technologies, ChatGPT will undoubtedly play an increasingly important role in both professional and personal contexts, driving efficiency, creativity, and productivity across various sectors.
Frequently Asked Questions (FAQs)
How does ChatGPT handle sensitive or inappropriate content?
ChatGPT uses content filtering and moderation tools to detect and minimize sensitive or inappropriate content, aiming to provide safe and ethical responses aligned with OpenAI's use guidelines.
Is ChatGPT capable of learning from individual user interactions?
No, ChatGPT does not learn from individual user interactions in real time; it relies on periodic updates and retraining on new data sets to enhance its capabilities.
What distinguishes GPT-4 from earlier versions like GPT-3?
GPT-4 offers enhanced multimodal capabilities, greater accuracy, and improved context retention over GPT-3, making it better suited for diverse and complex applications.