AI Guardrails: Ensuring Safe & Responsible Use of Generative AI
Written By
Published On
Read Time
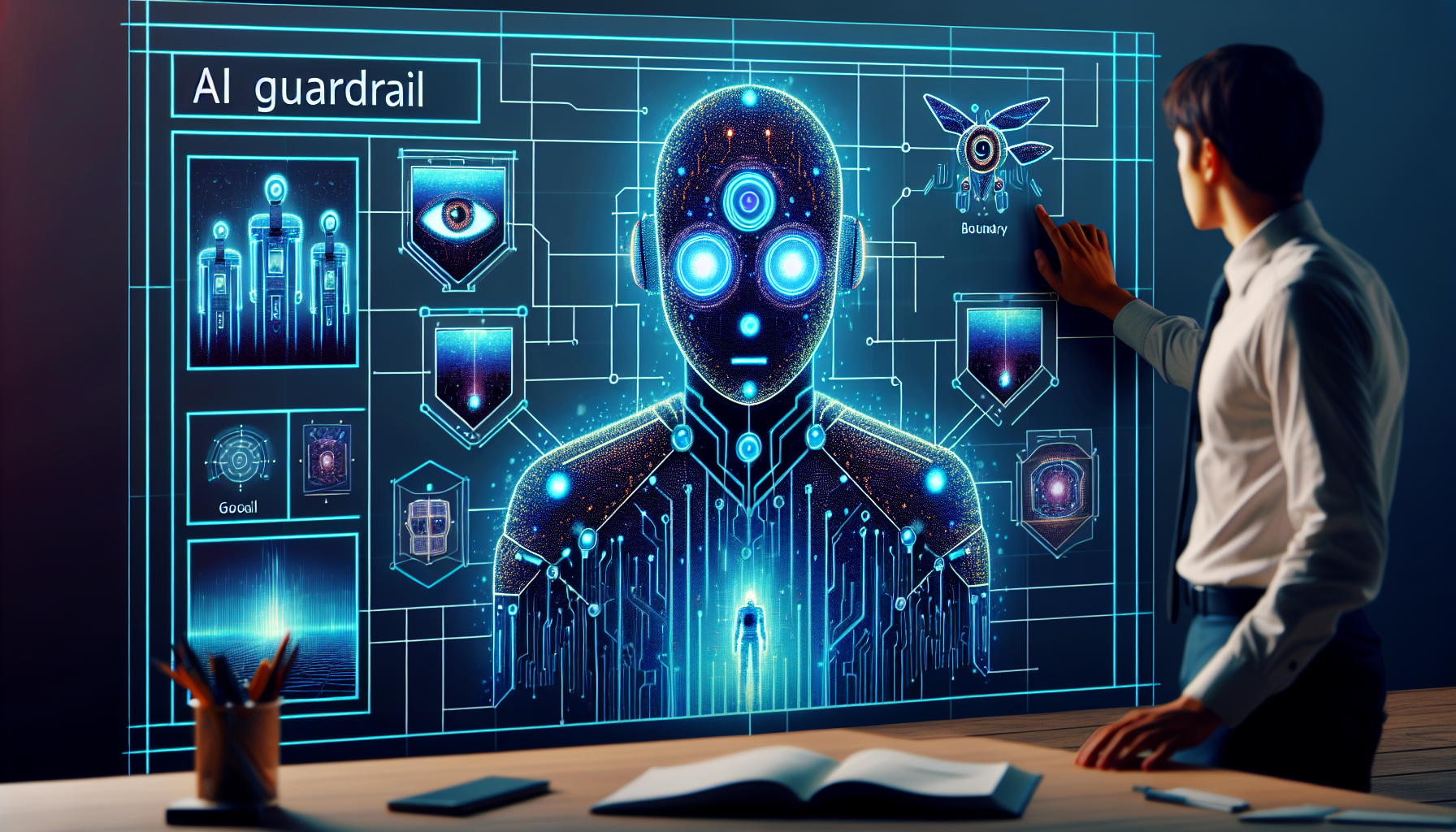
The phrase "guardrails" usually means the methods, rules, and habits set up to make sure artificial intelligence (AI) systems work in a safe, ethical way, staying within set limits. This is especially true for generative AI models. These guardrails are crucial for using AI responsibly and for reducing risks and unexpected results. For companies thinking about using generative AI, guardrails are what protect them from giving their customers a bad experience. This article will explore why AI guardrails are necessary, the different kinds of guardrails that exist, and how using guardrails on generative AI affects marketing.
What is an AI Guardrail?
AI guardrails are essential safeguards in the realm of artificial intelligence, designed to prevent AI systems from causing harm and ensuring their operation within ethical and legal boundaries. These guardrails are akin to highway guardrails, both aiming to promote safety and guide positive outcomes. As AI continues to evolve, the significance of guardrails escalates, especially for maintaining public trust and ensuring safe AI-enabled technology operations.

Source: Forbes
Why Do We Need AI Guardrails?
The advent of advanced AI technologies, particularly generative AI and Large Language Models (LLMs), has ushered in a new era of innovation and potential. However, with great power comes great responsibility, and this is where AI guardrails become indispensable. AI guardrails are essential mechanisms designed to ensure that AI systems, especially generative AI models, operate within safe, ethical, and legal boundaries. They are akin to safety barriers on highways, guiding AI towards beneficial outcomes while preventing potential harm.
Ensuring Ethical and Safe AI Deployment
AI guardrails are vital in maintaining the ethical integrity and safety of AI applications. As AI systems, including LLMs like GPT-3 and BERT, become more integrated into our daily lives and business operations, the risks associated with their misuse or malfunction cannot be overlooked. AI guardrails help in mitigating these risks by setting predefined rules, limitations, and operational protocols. This includes preventing AI from generating misleading, inappropriate, or harmful content, and safeguarding against security vulnerabilities.
Maintaining Public Trust and Legal Compliance
Public trust in AI is a fragile commodity. Instances of AI perpetuating biases, infringing on privacy, or making unethical decisions can lead to widespread mistrust. AI guardrails play a crucial role in building and maintaining this trust by ensuring that AI systems operate transparently and accountably. Additionally, they help in aligning AI operations with legal standards, particularly crucial in sectors like healthcare, finance, and legal services, where regulatory compliance is non-negotiable.
Balancing Innovation with Societal Norms
The rapid pace of AI development poses a challenge in balancing technological innovation with societal norms and values. AI guardrails provide a framework within which AI can evolve without overstepping ethical boundaries or societal expectations. This balance is crucial for sustainable and socially responsible AI advancement.
Examples and Implementation in Real-World Scenarios
In practical terms, AI guardrails manifest in various forms. For instance, Nvidia's NeMo tool helps developers build rules to limit LLMs' functionalities, such as restricting certain topics or detecting misinformation. In customer service applications, guardrails ensure that chatbots trained on customer interactions do not pass through personal identifiable information (PII) to the model, thereby protecting user privacy.

Three Pillars of AI Guardrails
With the advent of generative AI and Large Language Models (LLMs), the implementation of AI guardrails has become a critical factor in ensuring safe, ethical, and responsible AI deployment. These guardrails are built on three foundational pillars, each playing a vital role in guiding AI systems toward beneficial and trustworthy outcomes.
1. Policy Enforcement
Policy enforcement is a cornerstone of AI guardrails, ensuring that AI systems, including generative AI and LLMs, operate within defined ethical and legal boundaries. This involves setting and adhering to policies that align with a company's ethical guidelines and legal requirements. In practice, this means ensuring that the responses and actions of AI systems, such as Chat-GPT or DALL-E, stay within acceptable limits defined by the enterprise. For example, Nvidia's NeMo tool represents a practical implementation of this pillar, allowing developers to build rules that limit LLM functionalities, such as topic restrictions and misinformation detection.
Policy enforcement in AI guardrails serves multiple purposes:
Legal Compliance: Ensuring AI systems comply with relevant laws and regulations, particularly in sensitive sectors like healthcare and finance.
Ethical Alignment: Aligning AI operations with societal norms and ethical standards to maintain public trust and avoid reputational risks.
Operational Integrity: Maintaining the integrity of AI systems by preventing misuse and ensuring they function as intended.
2. Contextual Understanding
Enhancing the contextual understanding of AI systems is another critical pillar of AI guardrails. Generative AI often lacks nuanced comprehension of context, leading to responses that can be off-mark or potentially harmful. Improving this aspect enhances the AI model's ability to interact effectively and safely. This involves training AI systems to better understand the subtleties and complexities of human language and context, thereby reducing the risk of generating inappropriate or misleading content.
Key aspects of contextual understanding in AI guardrails include:
Relevance and Appropriateness: Ensuring AI-generated content is relevant and appropriate for the given context, avoiding outputs that may be harmless in one setting but inappropriate in another.
Accuracy and Reliability: Enhancing the accuracy of AI responses, particularly in critical applications like medical or legal advice.
Cultural and Social Sensitivity: Incorporating cultural and social awareness into AI systems to prevent biases and promote inclusivity.
3. Continuous Adaptability
The third pillar, continuous adaptability, acknowledges the dynamic nature of both the AI field and the broader societal and business landscapes. AI guardrails must be flexible and adaptable, allowing for updates and refinements in alignment with changing organizational needs, technological advancements, and societal norms. This adaptability ensures that AI systems remain relevant, safe, and effective over time.
Continuous adaptability in AI guardrails involves:
Regular Updates and Refinements: Continuously updating AI models and their guardrails to reflect new data, emerging trends, and evolving ethical standards.
Feedback Mechanisms: Implementing mechanisms for user feedback and real-time monitoring to identify and rectify issues promptly.
Future-Proofing: Anticipating future developments and challenges in AI to proactively adjust guardrails accordingly.
Types of AI Guardrails
AI guardrails can be broadly categorized into three types: Technical Controls, Policy-Based Guardrails, and Legal Guardrails. Each type plays a unique role in shaping the way AI systems are developed and utilized, ensuring they align with societal norms, ethical standards, and legal requirements.
Technical Controls
Technical controls in AI guardrails are embedded directly within the AI systems and workflows. They are operational processes that become an integral part of how the AI functions on a day-to-day basis. These controls are designed to ensure that AI systems, including generative AI and LLMs, operate within predefined safety and ethical parameters.
Key aspects of technical controls include:
Validation Tests: These are designed to verify that complex AI systems behave as intended, ensuring reliability and accuracy in their outputs.
Feedback Mechanisms: Allowing users to report errors or issues, feedback mechanisms are crucial for continuous improvement and adaptation of AI systems.
Security Protocols: Protecting AI systems from cyberattacks and misuse, security protocols are essential for maintaining the integrity and trustworthiness of AI applications.
Watermarks for AI-Generated Content: This helps in distinguishing AI-generated outputs from human-generated content, maintaining transparency and authenticity.
Policy-Based Guardrails
Policy-based guardrails are guidelines and frameworks that influence the design and management of AI workflows. Unlike technical controls, they are not embedded into the AI systems but rather guide the overall approach to AI development and deployment within an organization or industry.
Elements of policy-based guardrails include:
Data Management Policies: Guidelines on how training data should be collected, stored, and shared, ensuring privacy and ethical use of data.
Ethical AI Frameworks: Best practices and frameworks addressing concerns like fairness, accountability, and transparency in AI systems.
Industry-Specific Regulations: Policies tailored to specific industries, ensuring AI applications comply with sector-specific standards and practices.
Intellectual Property Policies: Governing the rights and usage of AI-generated content, these policies address the legal aspects of AI outputs.
Legal Guardrails
Legal guardrails consist of laws, regulations, and formal standards that govern the development and deployment of AI systems. They are enforceable and have a significant influence on both technical and policy-based guardrails.
Key components of legal guardrails include:
Legislation: Laws passed by governments addressing various aspects of AI, such as liability, privacy, and usage rights.
Regulatory Compliance: Regulations ensuring AI systems comply with existing legal frameworks, particularly in areas like data protection and user privacy.
Standards for Compliance Assessment: Formal standards used to assess AI systems' compliance with laws and regulations, ensuring legal accountability.
Setting Guardrails Throughout AI Design
In the dynamic field of artificial intelligence, particularly with the rise of generative AI and Large Language Models (LLMs), the concept of setting AI guardrails throughout the design process has become increasingly crucial. These guardrails are essential for ensuring that AI systems are developed and deployed in a manner that is safe, ethical, and aligned with societal norms. The process of setting these guardrails involves a comprehensive approach, encompassing various stages of AI design and development.
Integrating Guardrails in the Initial Design Phase
The initial design phase of AI, especially for generative AI and LLMs, is critical for laying the foundation of ethical and safe AI applications. At this stage, it's essential to establish clear objectives for what the AI system should achieve and the ethical boundaries it must adhere to. This involves:
Defining Ethical Principles: Establishing core ethical principles that the AI system will adhere to, such as fairness, transparency, and accountability.
Risk Assessment: Conducting thorough risk assessments to identify potential ethical and safety issues that might arise from the AI system's deployment.
Designing for Transparency: Ensuring the AI system is designed in a way that its decisions and processes can be understood and explained, fostering trust among users.
Guardrails During AI Development
As the AI system moves into the development phase, integrating guardrails becomes a matter of embedding ethical and safety considerations into the AI's architecture. This includes:
Data Governance: Implementing strict data governance policies to ensure the ethical sourcing and use of data, which is particularly crucial for training generative AI models.
Bias Mitigation: Incorporating mechanisms to detect and mitigate biases in AI algorithms, ensuring that the AI system does not perpetuate or amplify existing societal biases.
Security Measures: Embedding robust security measures to protect the AI system from potential threats and misuse.
Post-Development: Testing and Refinement
After the AI system is developed, it enters a critical phase of testing and refinement, where guardrails are crucial for ensuring the system's readiness for deployment. This stage involves:
Validation and Testing: Rigorous testing of the AI system to validate its performance against the set ethical and safety standards.
Feedback Loops: Establishing feedback mechanisms to gather insights from users and stakeholders, which can be used to continuously improve the AI system.
Compliance Checks: Ensuring the AI system complies with all relevant legal and regulatory requirements, particularly important for applications in regulated industries.
Deployment and Continuous Monitoring
Even after deployment, AI guardrails play a vital role in ensuring the ongoing ethical and safe operation of the AI system. This includes:
Real-Time Monitoring: Continuously monitoring the AI system's performance to quickly identify and address any ethical or safety issues that arise.
Adaptive Learning: Allowing the AI system to adapt and evolve over time, while ensuring that these changes adhere to the established guardrails.
User Education: Educating users about the capabilities and limitations of the AI system, ensuring they have realistic expectations and understand how to interact with the system responsibly.
Who Is Responsible For Creating AI Guardrails?
In the rapidly advancing world of artificial intelligence, particularly with the emergence of generative AI and Large Language Models (LLMs), the question of responsibility for creating AI guardrails is increasingly significant. AI guardrails are essential frameworks that ensure AI systems operate within safe, ethical, and legal boundaries. The responsibility for creating these guardrails is a collaborative and multi-faceted endeavor, involving various stakeholders from different sectors.
Collaboration Among Diverse Stakeholders
The development of effective AI guardrails is not the sole responsibility of any single entity but rather a collective effort involving a diverse group of stakeholders. Each group plays a unique role in shaping these guardrails, bringing different perspectives and expertise to the table.
Tech Companies and AI Developers: These are the primary creators of AI technologies, including generative AI and LLMs. They are responsible for embedding ethical considerations and safety features directly into AI systems from the ground up.
AI Researchers and Academics: Researchers contribute by exploring the ethical, social, and technical aspects of AI, providing insights and guidelines for responsible AI development.
Government Agencies and Regulatory Bodies: These entities are responsible for creating laws and regulations that define the legal framework within which AI must operate. They ensure that AI guardrails comply with national and international standards.
Industry Experts and Professional Organizations: Professionals from various industries contribute by developing industry-specific guidelines and best practices for AI deployment, ensuring that AI solutions are tailored to meet the unique needs and challenges of different sectors.
Ethicists and Civic Organizations: These groups advocate for the ethical use of AI, ensuring that guardrails address broader societal concerns such as privacy, fairness, and transparency.
End-Users and the General Public: The feedback and concerns of end-users and the general public are crucial in shaping AI guardrails. They ensure that AI systems are user-friendly, transparent, and aligned with public expectations.
The Interdisciplinary Nature of AI Guardrails
Creating AI guardrails is an interdisciplinary task that requires a blend of technical expertise, ethical considerations, legal knowledge, and societal understanding. It involves:
Technical Design and Implementation: Engineers and developers work on the technical aspects, designing AI systems with built-in safeguards and ethical algorithms.
Legal and Regulatory Frameworks: Legal experts and policymakers develop regulations and standards that guide the ethical and lawful use of AI.
Ethical Guidelines and Social Considerations: Ethicists and social scientists ensure that AI guardrails respect human values, rights, and societal norms.
Continuous Collaboration and Adaptation
The responsibility for creating AI guardrails is an ongoing process, requiring continuous collaboration and adaptation to new developments in AI technology and changes in societal values and legal landscapes. This dynamic process ensures that AI guardrails remain relevant, effective, and aligned with the evolving nature of AI and its impact on society.
Implementing Guardrails in Various Stages of AI Development
In the development of artificial intelligence, particularly in the context of generative AI and Large Language Models (LLMs), implementing AI guardrails at various stages is crucial for ensuring the safety, ethics, and effectiveness of these technologies. AI guardrails serve as protective measures, guiding AI systems to operate within desired parameters and preventing unintended consequences. Let's explore how these guardrails are implemented during different stages of AI development.
Guardrails During Training
The training stage is critical in AI development, especially for generative AI and LLMs, as it sets the foundation for how the AI system will behave.
Data Selection and Preparation: Implementing guardrails at this stage involves careful selection and preparation of training data to avoid biases and ensure diversity and representativeness.
Ethical Considerations: Ethical guardrails are put in place to prevent the AI from learning harmful or discriminatory patterns. This includes filtering out inappropriate content and ensuring that the data aligns with ethical standards.
Security Measures: To protect the integrity of the AI model, guardrails for data security and privacy are essential. This includes measures to anonymize data and protect sensitive information.
Guardrails For Prompts and Inputs
As AI systems, particularly LLMs, often rely on prompts and inputs to generate outputs, setting guardrails in this area is crucial for controlling the behavior of the AI.
Input Validation: Guardrails here involve validating the inputs to ensure they are appropriate and within the expected domain. This prevents the AI system from processing harmful or irrelevant prompts.
Prompt Design: Designing prompts in a way that guides the AI towards desired responses and away from unethical or biased outputs is a key guardrail strategy.
Handling Ambiguity: Implementing mechanisms to handle ambiguous or unclear inputs can prevent the AI from making incorrect or harmful assumptions.
Guardrails For Outputs
The output stage is where the AI interacts with the world, making it crucial to have strong guardrails to ensure that these interactions are safe, ethical, and aligned with user expectations.
Content Moderation: This involves filtering the AI's outputs to remove or flag inappropriate, biased, or harmful content.
Accuracy and Reliability Checks: Ensuring the outputs are accurate and reliable, particularly in critical applications like medical or legal advice, is a key guardrail.
Feedback Loops: Implementing feedback mechanisms allows for the continuous improvement of the AI system, ensuring that outputs remain aligned with ethical and safety standards.
Practical Implementation of Guardrails in LLMs
In the rapidly evolving landscape of Large Language Models (LLMs) and generative AI, the practical implementation of AI guardrails is crucial for ensuring these technologies are used safely, ethically, and effectively. Guardrails in LLMs involve a series of measures and strategies that guide the development and usage of AI systems, ensuring they align with ethical standards and societal expectations. Let's delve into the key aspects of implementing these guardrails.
Transparency and Accountability
Transparency and accountability are fundamental in the implementation of AI guardrails, especially in LLMs and generative AI systems.
Clear Documentation: This involves providing detailed documentation of the AI system's development process, including data sources, training methodologies, and limitations. This transparency helps users understand how the model makes decisions.
Audit Trails and Evaluations: Implementing audit trails and encouraging third-party evaluations can enhance accountability. These practices ensure that AI applications are designed with ethical considerations in mind and adhere to established standards.
Open Communication: Maintaining open communication channels about the capabilities and limitations of AI systems fosters trust and understanding among users and stakeholders.
User Education and Guidelines
Educating users about the capabilities and limitations of LLMs is essential for mitigating risks associated with misuse or misunderstanding of AI technologies.
Developing User Guidelines: Providing clear guidelines and FAQs can help set the right expectations and prevent unintended or harmful outcomes.
Training Programs: Implementing training programs for users to understand how to interact with AI systems responsibly and effectively can significantly reduce misuse and enhance the user experience.
Awareness Campaigns: Conducting awareness campaigns about the potential and limitations of AI can help demystify AI technologies and promote informed usage.
Real-Time Monitoring and Control
Continuous oversight is crucial for the ongoing safe operation of LLMs and generative AI systems.
Monitoring Tools: Integrating real-time monitoring tools allows for the continuous oversight of AI systems, enabling quick interventions if the model generates harmful or misleading information.
Human-in-the-Loop Systems: Designing AI systems with human-in-the-loop mechanisms ensures that critical decisions are reviewed and validated by human experts, adding an additional layer of safety and reliability.
Adaptive Response Mechanisms: Implementing systems that can adapt and respond to unexpected situations or outputs in real-time is crucial for maintaining the integrity and safety of AI applications.
Feedback Loops
In the context of AI guardrails, particularly for generative AI and Large Language Models (LLMs), feedback loops play a crucial role in ensuring continuous improvement and adaptation of AI systems. These loops are mechanisms through which AI systems can be refined and optimized based on user interactions and outcomes.
User Feedback Integration: Incorporating user feedback into the AI system is essential for identifying areas of improvement. This feedback can come from various sources, including direct user reports, behavior analysis, and interaction patterns.
Iterative Improvement Process: AI systems, especially LLMs, benefit from an iterative process where they are continuously updated based on the feedback received. This process helps in fine-tuning the AI's responses and functionalities, ensuring they remain relevant and effective.
Error Reporting and Resolution: Implementing a robust system for error reporting and resolution is a key aspect of feedback loops. Users should be able to easily report any issues or inaccuracies in the AI's output, which can then be used to improve the model.
Legal and Ethical Framework
The implementation of AI guardrails must be grounded in a strong legal and ethical framework to ensure that AI technologies, including generative AI and LLMs, are developed and used responsibly.
Compliance with Laws and Regulations: AI systems must adhere to existing legal frameworks, including data protection laws, privacy regulations, and intellectual property rights. This compliance ensures that AI technologies operate within the legal boundaries.
Ethical Standards Alignment: Aligning AI systems with ethical standards involves ensuring fairness, transparency, and accountability in AI operations. This includes measures to prevent bias, protect user privacy, and ensure that AI decisions are explainable and justifiable.
Development of New Legal Frameworks: Given the rapid advancement in AI, there is often a need for new legal frameworks that specifically address the unique challenges posed by AI technologies. Collaboration between technologists, legal experts, and policymakers is crucial in this regard.
Safety
Safety is a paramount consideration in the implementation of AI guardrails, particularly for generative AI and LLMs, to prevent misuse and harmful consequences.
Risk Assessment and Management: Conducting thorough risk assessments to identify potential safety issues and implementing strategies to manage these risks is crucial. This includes evaluating the AI system for vulnerabilities that could be exploited for malicious purposes.
Safe Deployment Practices: Ensuring safe deployment practices involves rigorous testing of AI systems before they are released into real-world environments. This testing should cover various scenarios to ensure the AI behaves safely under different conditions.
Proactive Safety Measures: Implementing proactive safety measures, such as real-time monitoring for harmful or inappropriate content generation and setting up mechanisms to prevent the AI from being used for unethical purposes, is essential.
Red Teaming
In the context of AI guardrails, particularly for generative AI and Large Language Models (LLMs), Red Teaming plays a crucial role in enhancing system security and reliability. Red Teaming involves a group of experts simulating potential attacks or challenging situations to test the resilience and response capabilities of AI systems.
Identifying Vulnerabilities: Red Teams rigorously test AI systems, including LLMs, to identify vulnerabilities that could be exploited by malicious actors. This proactive approach helps in uncovering potential weaknesses before they can be exploited in real-world scenarios.
Simulating Adversarial Attacks: By simulating adversarial attacks, Red Teams provide valuable insights into how AI systems might respond to malicious inputs or manipulation attempts, ensuring robustness against such threats.
Enhancing Security Measures: The insights gained from Red Teaming are used to enhance the AI system's security measures, making them more resilient to attacks and misuse. This is particularly important for AI systems that handle sensitive data or are used in critical applications.
Enterprise-Specific LLMs
Enterprise-specific LLMs refer to customized Large Language Models that are tailored to meet the specific needs and requirements of an organization. These models offer several advantages in terms of alignment with enterprise-specific goals and compliance requirements.
Customization for Specific Needs: Enterprise-specific LLMs are designed to align closely with the unique operational, ethical, and data privacy requirements of an organization, offering more targeted and relevant functionalities.
Control Over Data and Training: Organizations have greater control over the data used for training these models, ensuring that the outputs are highly relevant and compliant with internal policies and standards.
Enhanced Privacy and Security: By using enterprise-specific LLMs, organizations can ensure that their data remains within their control, reducing the risk of data breaches and enhancing overall data security.
Optimized LLMs
Optimized LLMs involve the use of advanced techniques to improve the performance, accuracy, and efficiency of Large Language Models. This optimization is crucial for ensuring that LLMs deliver the best possible outcomes in various applications.
Advanced Training Techniques: Utilizing advanced training techniques such as reinforcement learning and fine-tuning based on specific use cases can significantly enhance the performance of LLMs.
Efficiency Improvements: Optimization also focuses on making LLMs more efficient in terms of processing speed and resource utilization, which is crucial for scaling AI applications.
Customization for Better Outcomes: By optimizing LLMs, organizations can tailor the models to produce more accurate and relevant outputs, thereby improving the overall effectiveness of their AI applications.
Agent-Based Modeling
Agent-based modeling in the context of AI guardrails refers to the use of automated systems that can enforce rules and policies within AI applications, particularly in LLMs. This approach offers a dynamic and flexible way to manage and control AI systems.
Automated Governance: Agent-based models can automatically enforce governance policies and ethical standards, ensuring that AI systems operate within predefined boundaries.
Dynamic Adaptation: These models are capable of adapting to changes in policies, user behavior, and external environments, making them highly effective for managing complex AI systems.
Enhanced User Experience: By automating the governance process, agent-based models can simplify the user experience, making it easier for users to interact with AI systems without needing deep technical expertise.
How do you Maximize the benefits of LLMs with proper guardrails?
Large Language Models (LLMs) like GPT-3 and BERT have revolutionized various sectors by offering advanced capabilities in natural language processing. However, to fully harness their potential while ensuring safety, ethical integrity, and compliance, implementing proper AI guardrails is essential. These guardrails not only mitigate risks but also enhance the effectiveness and trustworthiness of LLMs. Let's explore how to maximize the benefits of LLMs with appropriate guardrails.
Establishing Clear and Ethical Guidelines
Defining Ethical Boundaries: Establishing clear ethical guidelines for LLMs is crucial. This involves setting parameters that prevent the generation of biased, discriminatory, or harmful content. By doing so, LLMs can be used in a way that aligns with societal norms and values.
Incorporating Fairness and Transparency: Implementing guardrails that ensure fairness and transparency in LLM operations enhances user trust. This includes mechanisms for explaining how the LLM arrives at certain outputs, making the AI's decision-making process more transparent.
Enhancing Data Security and Privacy
Robust Data Protection: Implementing guardrails that focus on data security and privacy is essential, especially when LLMs handle sensitive information. This involves encrypting data and ensuring that user privacy is maintained, thereby building trust and compliance with data protection regulations.
Continuous Monitoring and Adaptation
Real-Time Monitoring: Setting up real-time monitoring systems as part of AI guardrails helps in quickly identifying and addressing any ethical or safety issues that arise. This ensures that LLMs remain safe and reliable over time.
Adaptive Learning Mechanisms: Incorporating adaptive learning mechanisms allows LLMs to evolve and improve based on user feedback and changing environments. This adaptability ensures that LLMs continue to provide relevant and effective solutions.
User-Centric Approach
Educating Users: Providing comprehensive education and guidelines to users about the capabilities and limitations of LLMs ensures that they are used effectively and responsibly. Understanding how to interact with LLMs can significantly enhance user experience and outcome quality.
Feedback Loops: Establishing feedback loops where users can report issues or concerns contributes to the continuous improvement of LLMs. This user input is invaluable for refining the model and aligning it more closely with user needs.
Legal Compliance and Industry Standards
Regulatory Adherence: Ensuring that LLMs comply with existing laws and industry standards is a critical aspect of AI guardrails. This includes staying updated with evolving regulations and modifying the LLMs accordingly to maintain legal compliance.
Industry-Specific Customization: Tailoring LLMs to meet specific industry requirements can maximize their utility. For instance, in healthcare or legal services, LLMs can be customized to align with industry-specific language and ethical standards.
Conclusion
As we delve deeper into the era of advanced AI, the significance of AI guardrails cannot be overstated. They are the linchpins that ensure AI technologies, especially generative AI and LLMs, are not only technologically advanced but also operate in a manner that is beneficial, ethical, and aligned with societal values. The implementation of these guardrails is a shared responsibility that involves collaboration among various stakeholders, including AI developers, users, ethicists, and policymakers.
AI guardrails stand at the forefront of the ethical AI revolution, steering AI towards a future where its benefits are maximized, and its risks are effectively managed. They represent a commitment to responsible AI deployment, ensuring that as we step into the future with AI, we do so with a framework that promotes safety, ethics, and sustainability.