A/B Testing: The Power of Data-Driven Marketing
Written By
Published On
Read Time
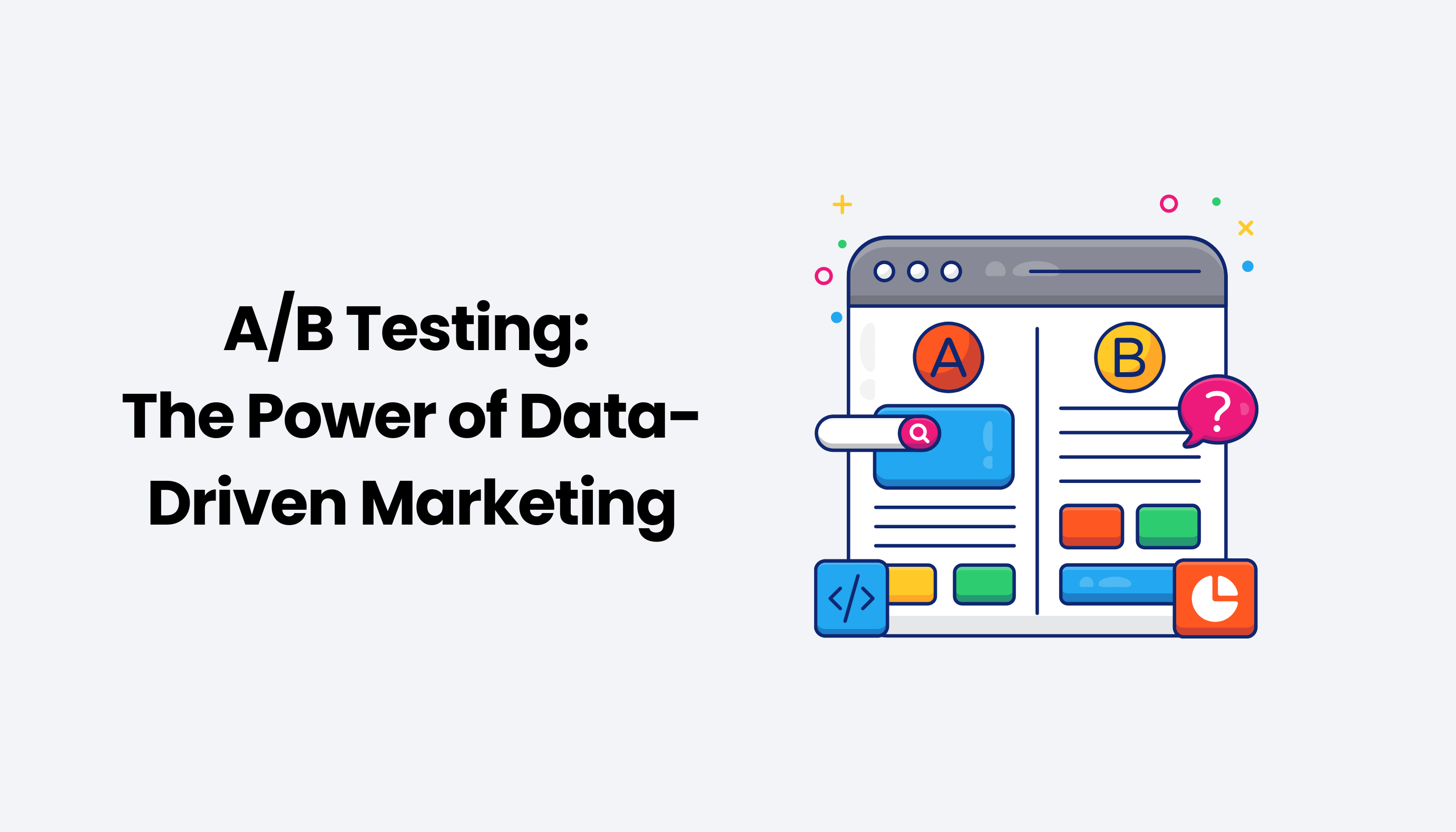
A/B testing: where data and marketing come together!
This guide will explain the different types of A/B testing, why it’s key to marketing success, and provide a step-by-step guide on how to run A/B tests and use the results to improve your strategies with data-driven decisions.
What is A/B Testing?
A/B testing, often referred to as split testing, is a critical technique in digital marketing that allows marketers to compare two variations of a web page, email, or other digital content to determine which one performs better. By isolating and testing individual elements such as headlines, call-to-action (CTA) buttons, or images, marketers can make data-driven decisions that optimize conversion rates, improve user experience, and ultimately enhance overall campaign performance.
A/B testing is fundamental in digital marketing because it enables businesses to understand which versions of their content resonate most with their audience. For instance, a typical A/B test might involve showing version A of a landing page to one group of users and version B to another, each differing in only one aspect, such as the CTA color or headline. The variation that drives more engagement or conversions is then used as the standard for future campaigns.
History of A/B Testing
The origins of A/B testing date back to early advertising practices, evolving significantly with the advent of digital marketing. Understanding the evolution of A/B testing is crucial to appreciating its role today in optimizing conversion rates, enhancing user experience, and refining marketing strategies.
Early Beginnings in Advertising
The roots of A/B testing can be traced back to the early 20th century when Claude Hopkins, a pioneer in advertising, introduced systematic approaches to testing in marketing. Hopkins is often credited with developing the foundation of what we now consider A/B testing marketing. He would test different versions of advertisements and track which performed better in driving conversions. However, his methods were more qualitative and lacked the statistical rigor that defines modern A/B testing methodology.
The Statistical Revolution
A significant leap in the AB testing methodology came from the work of Ronald Fisher, a statistician in the early 20th century. Fisher introduced concepts like the null hypothesis and statistical significance, which are core principles in AB testing digital marketing today. These statistical foundations allowed marketers to test changes scientifically and validate results, ensuring that observed differences were not due to random chance but had actual significance.
The Shift to Digital Marketing
While A/B testing saw sporadic use in traditional marketing, it gained momentum in the digital age. The rapid growth of the internet and digital marketing opened new avenues for testing different variations of webpages, emails, and online ads. In the late 1990s and early 2000s, companies like Google played a pivotal role in popularizing AB testing website techniques. For example, Google’s early A/B tests focused on determining the optimal number of search results to display. These experiments laid the groundwork for how AB testing in digital marketing is conducted today.
A/B Testing in the Early 2000s
The early 2000s marked a turning point for A/B testing in marketing as more businesses adopted AB testing methodologies to improve their digital presence. The rise of dedicated tools like Optimizely, Google Optimize, and other conversion rate optimization (CRO) platforms provided marketers with the ability to easily conduct A/B tests on websites, landing pages, emails, and more. These tools allowed for automated testing and real-time data analysis, significantly improving the accessibility and effectiveness of AB testing for websites.
The Growth of Multivariate Testing and Complex Experimentation
As AB testing in digital marketing matured, it expanded beyond simple comparisons between two variants (A and B). Marketers began adopting more complex methodologies like multivariate testing, which tests multiple variables simultaneously, offering deeper insights into how different combinations of changes impact results. While multivariate testing requires more traffic and data to yield statistically significant results, it provides a more comprehensive understanding of how various elements interact.
Present-Day A/B Testing
Today, A/B testing is a staple of digital marketing strategies across industries. Marketers routinely use AB testing to refine everything from website A/B testing to email marketing campaigns and ad creatives. It is widely recognized as one of the most reliable methods for optimizing digital content and user experience.
The sophistication of A/B testing tools has also grown, allowing for more granular audience segmentation, predictive analytics, and even automated decision-making based on machine learning algorithms. These advancements make it easier for businesses to run continuous A/B tests that adapt to changing consumer behaviors in real-time.
Why is A/B Testing Important?
In the competitive landscape of digital marketing, making data-driven decisions is essential for optimizing performance and maximizing ROI. A/B testing—often referred to as split testing—is a critical tool in this process, allowing marketers to experiment with different versions of their content and determine what resonates best with their audience. The importance of A/B testing in digital marketing cannot be overstated, as it provides actionable insights that lead to improved conversion rates, better user experience, and more efficient marketing strategies.
Data-Driven Decision Making
One of the key reasons A/B testing is important is its ability to replace guesswork with data-driven decision-making. Marketers are often faced with choices—such as which headline to use, which call-to-action (CTA) to prioritize, or what design elements drive engagement. Instead of relying on intuition, AB testing methodology allows marketers to test these variables scientifically and determine which option yields better results. For instance, running an AB testing marketing campaign to compare two landing page designs can reveal which layout drives more conversions, leading to more informed decisions that are backed by hard data.
Conversion Rate Optimization (CRO)
A/B testing is foundational to conversion rate optimization (CRO), a process aimed at improving the percentage of users who take a desired action, such as making a purchase or signing up for a newsletter. Whether it’s tweaking the color of a CTA button, refining the headline, or optimizing the placement of key elements, A/B testing allows marketers to make small, incremental changes that collectively drive significant improvements in conversion rates. By identifying which elements perform best through AB testing, businesses can increase their return on investment (ROI) without necessarily increasing their marketing spend.
Enhancing User Experience
Another significant benefit of A/B testing in marketing is its role in improving user experience (UX). A well-optimized website or marketing asset is not just visually appealing; it’s also intuitive and easy to navigate. A/B testing on websites can reveal friction points that may be hindering user interaction, such as confusing navigation menus or poorly placed CTAs. By testing different design elements and layouts, marketers can create a more seamless and enjoyable experience for users, leading to higher engagement and satisfaction.
Reducing Bounce Rates and Improving Engagement
Bounce rates—when visitors leave a website after viewing just one page—can be a major concern for marketers. A/B testing can be used to address this by experimenting with different versions of landing pages, headlines, and content layouts to determine what keeps users engaged. For example, testing different opening lines for blog posts or varying the length of content can help reduce bounce rates and encourage deeper engagement. AB testing allows marketers to identify which variations are most effective at retaining visitors and encouraging them to explore more content, thereby improving overall website performance.
Maximizing the Impact of Marketing Campaigns
In the realm of AB testing digital marketing, optimizing each element of a marketing campaign is crucial. From email subject lines to ad creatives, A/B testing enables marketers to fine-tune their campaigns for maximum impact. For instance, A/B testing in email marketing might involve testing different subject lines or CTA placements to see which variant leads to higher open rates and click-through rates. Similarly, testing different ad copy and visuals can lead to higher engagement and conversions in paid search or social media campaigns.
Risk Mitigation
Launching new marketing initiatives always carries an element of risk, especially when changes are based purely on assumptions or trends. A/B testing mitigates this risk by allowing marketers to validate their ideas on a small scale before rolling them out more broadly. By testing one change at a time and gathering real user feedback, businesses can avoid costly mistakes and implement changes that are proven to be effective. This controlled approach to testing ensures that resources are allocated to strategies that are most likely to deliver results.
Long-Term Benefits and Continuous Improvement
A/B testing is not a one-time activity but a continuous process of optimization. As market conditions, user preferences, and digital trends evolve, so too should a company’s marketing strategies. Regular A/B testing provides ongoing insights that can be applied across various campaigns, ensuring that marketing efforts remain effective and aligned with audience needs. Whether it’s how to AB test new design features or optimize existing ones, continuous testing enables businesses to stay ahead of the curve and maintain a competitive edge.
How Does A/B Testing Work?
A/B testing is a fundamental methodology in digital marketing that allows businesses to make data-driven decisions by comparing two variations of a webpage, email, or other digital assets. The goal of A/B testing is to identify which version performs better in terms of user engagement, conversion rates, and other key performance metrics. Understanding how A/B testing works is crucial for marketers looking to optimize their campaigns and enhance user experience.
Step 1: Identifying a Variable to Test
The first step in A/B testing in marketing is to decide what you want to test. This variable could be anything that potentially impacts user behavior, such as a headline, call-to-action (CTA), button color, layout, or image. For instance, if you notice that a landing page has a low conversion rate, you might hypothesize that changing the CTA text or button color could improve performance. In this case, these elements would become the focus of your AB testing methodology.
Step 2: Creating Two Versions - Control and Variation
Once the variable is identified, the next step is to create two versions of the content: the control (Version A) and the variation (Version B). The control represents the original version, while the variation includes the change you want to test. For example, if your hypothesis is that a red CTA button will generate more clicks than a blue one, Version A would have the blue button, and Version B would have the red button. In AB testing digital marketing, it’s essential to change only one element at a time to ensure that any differences in performance can be attributed to that specific change.
Step 3: Splitting the Audience
To ensure accurate results, A/B testing requires that your audience be randomly split into two groups: one group interacts with Version A, while the other interacts with Version B. This randomization is key to eliminating biases and ensuring that both versions are tested under similar conditions. Tools like Google Optimize, Optimizely, and HubSpot’s built-in A/B testing feature make it easy to split traffic and deliver the right version to the right segment of your audience.
Step 4: Running the Test
Once your audience is divided, the test is run over a predetermined period. The duration of the test is crucial in determining the validity of the results. The testing period should be long enough to gather a statistically significant amount of data, but not so long that external factors—like seasonal changes—skew the results. In most cases, tests are run until a confidence level of at least 95% is reached, which indicates that the winning variation’s performance is not due to random chance.
Step 5: Measuring and Analyzing Results
After the test is complete, the next step is to measure and analyze the results based on predefined metrics, such as conversion rate, click-through rate (CTR), or bounce rate. Tools used in AB testing for websites often provide built-in analytics that help marketers determine which version performed better. If Version B shows a statistically significant improvement over Version A, you’ve found a winner. However, if the results are inconclusive, you may need to run additional tests or refine your hypothesis.
Step 6: Implementing the Winning Variation
If one variation proves to be superior, the next logical step is to implement it across your marketing assets. This could involve updating your landing pages, emails, or ad campaigns with the winning design or content. The key here is that A/B testing enables continuous optimization—marketers can keep refining their content to achieve better results over time. For example, after finding that a red CTA button works better, you might next test different CTA copy or placement to further enhance conversions.
The Iterative Nature of A/B Testing
A core principle of A/B testing in digital marketing is its iterative nature. The process doesn’t end with a single test; instead, it’s a cycle of continuous improvement. Marketers are encouraged to test new hypotheses regularly, optimizing each element of their campaigns incrementally. For instance, after improving the CTA button color, the next step could involve testing different headline variations or experimenting with layout changes. This iterative process helps refine marketing strategies and aligns them with changing consumer preferences.
Common Use Cases for A/B Testing
Typical use cases for A/B testing in marketing include optimizing landing pages, improving email open rates, and refining digital ads. For example:
Website A/B Testing: Testing different homepage designs, product descriptions, or checkout flows to enhance user experience and increase conversions.
Email Campaigns: Testing subject lines, preview text, and email content to improve open rates and click-through rates.
Digital Ads: Experimenting with different ad copy, headlines, and visuals to maximize engagement and ROI.
A/B Testing in Marketing
A/B testing in marketing is a powerful strategy that enables marketers to make data-driven decisions and optimize their campaigns for maximum effectiveness. By comparing two different versions (A and B) of a marketing element—whether it’s a webpage, email, or digital ad—A/B testing helps determine which version performs better. This process is fundamental to enhancing conversion rates, improving user experience, and refining marketing strategies across digital channels.
What is A/B Testing in Marketing?
A/B testing in digital marketing involves presenting two versions of a marketing asset (e.g., a landing page, email, or ad) to segments of your audience to see which performs better based on predefined metrics like click-through rate (CTR), conversion rate, or bounce rate. The two versions are typically identical except for one variable, such as a headline, CTA button color, or image. By isolating and testing these variables, marketers can gain insights into what drives user behavior and refine their strategies accordingly.
The Role of A/B Testing in Marketing Campaigns
In digital marketing, optimization is key to success. A/B testing marketing strategies allow businesses to fine-tune every element of their campaigns, leading to more personalized, impactful interactions with their audience. Whether testing email subject lines or website layouts, A/B testing provides valuable insights that drive better outcomes.
Optimizing Conversion Rates: A primary application of A/B testing in marketing is conversion rate optimization (CRO). By testing elements like CTAs, headlines, or form fields, marketers can determine which variations lead to more sign-ups, purchases, or other desired actions. For example, testing different CTA button colors or positions can reveal what catches users' attention and drives more conversions.
Improving Email Marketing Campaigns: A/B testing is essential for optimizing email campaigns. By experimenting with subject lines, preview text, and email body content, marketers can improve open rates and click-through rates. For instance, an A/B test might reveal that personalized subject lines perform better than generic ones, leading to more effective email marketing strategies.
Enhancing Paid Advertising Campaigns: In paid search and display advertising, A/B testing allows marketers to experiment with different ad creatives, headlines, and copy. Testing various elements can lead to higher click-through rates and lower costs per acquisition. For example, testing multiple ad headlines helps identify the most compelling message for the target audience, ensuring that ad spend is directed toward high-performing versions.
How to Run an A/B Test in Marketing
Running a successful A/B test involves several key steps:
Identify the Variable to Test: Start by choosing a single variable that you want to test. This could be a headline, CTA, layout, or any other element that could influence user behavior. Focusing on one variable ensures that the results can be attributed directly to that change.
Create the Control and Variation: The control (Version A) is the original version, while the variation (Version B) introduces the change. For example, if you are testing a landing page, the control might have a blue CTA button, while the variation has a red button.
Split the Audience: Use tools like Google Optimize, Optimizely, or HubSpot to randomly divide your audience between the control and variation. It’s important that the audience segments are evenly distributed and representative to avoid skewing the results.
Run the Test and Gather Data: The test should run for a period long enough to collect statistically significant data. Depending on your traffic and the nature of the change, this could take days or weeks. Aim for a confidence level of at least 95% to ensure the results are reliable.
Analyze the Results: Once the test concludes, analyze the performance based on your key metrics—whether it’s conversion rate, CTR, or another KPI. If the variation outperforms the control, you can confidently implement the change across your marketing assets.
Iterate and Continue Testing: A/B testing is an iterative process. Even after finding a winning variation, there are always more opportunities to optimize. You might start by testing the CTA text, then move on to testing different images, copy, or layouts. Continuous testing leads to incremental improvements that add up over time.
Typical Uses of A/B Testing in Marketing
A/B testing has broad applications across digital marketing, making it a versatile tool for improving performance:
Website A/B Testing: Optimizing landing pages, homepages, product pages, and checkout processes. Marketers often use A/B testing to reduce bounce rates, increase conversions, and enhance user experience.
Email Campaigns: Testing subject lines, email body content, and CTA placement to improve open rates and click-through rates.
SEO and Content Marketing: Experimenting with meta descriptions, headlines, and content formats to improve search engine rankings and engagement.
Paid Advertising: Refining ad copy, creatives, and targeting options to maximize the return on ad spend (ROAS).
The Importance of A/B Testing in Digital Marketing
A/B testing in digital marketing is not just about making incremental improvements; it’s about understanding your audience at a deeper level. Every test provides insights into what resonates with your users, allowing you to tailor your marketing efforts more effectively. By systematically testing and optimizing various elements, marketers can improve user experience, increase conversions, and drive better business results.
A/B Testing Goals
In AB testing digital marketing, setting clear and precise goals is essential for determining the success of your tests and guiding the optimization of your marketing campaigns. A/B testing is a methodical process designed to refine and improve various aspects of digital marketing initiatives, from website performance to email campaign effectiveness. By defining specific A/B testing goals, marketers can focus on optimizing key metrics that directly impact business outcomes.
Increased Website Traffic
Driving more traffic to a website is one of the primary goals of A/B testing marketing strategies. Higher traffic usually means more opportunities for conversions, whether that’s generating leads, making sales, or driving engagement. A/B testing can be used to refine various elements that directly impact a website’s ability to attract and retain visitors.
Optimizing Headlines and Meta Tags: One of the most typical uses of A/B testing is to experiment with different headlines, page titles, and meta descriptions. These elements play a significant role in search engine optimization (SEO) and can influence click-through rates (CTR) from search results. By testing variations of headlines and meta descriptions, you can determine which ones attract more clicks and, therefore, increase organic traffic. For instance, testing whether a headline focused on benefits ("How to Increase Your Revenue by 50%") performs better than one focused on features can lead to insights that improve overall SEO performance.
Improving Content Relevance and Engagement: AB testing digital marketing also involves experimenting with different content formats, such as blog post structures, use of multimedia, or even the placement of key information. For example, testing whether a video introduction increases engagement compared to text-based content can help determine which format draws more visitors. The goal is to make your content more appealing to your target audience, leading to longer session durations, lower bounce rates, and ultimately more traffic.
Testing Call-to-Action (CTA) Placement for Engagement: CTAs that prompt users to explore more pages, sign up for newsletters, or share content on social media can significantly impact website traffic. By A/B testing on websites, marketers can experiment with different CTA placements, styles, and messages to see which configuration generates more clicks and deeper user engagement. For instance, a CTA that’s prominently displayed above the fold might attract more attention than one placed at the bottom of a page.
Optimizing Landing Pages for Higher Search Rankings: Website A/B testing can be particularly effective in optimizing landing pages for search engines and user engagement. Testing different layouts, keyword usage, and page elements like navigation can lead to a more SEO-friendly design that not only attracts more visitors but also keeps them on the site longer.
Higher Conversion Rate
While increased traffic is essential, it’s only valuable if those visitors convert into leads, customers, or subscribers. Optimizing for higher conversion rates is another critical goal in A/B testing in digital marketing. Conversion optimization focuses on refining specific elements that encourage users to take desired actions, whether that’s filling out a form, making a purchase, or signing up for a service.
Optimizing Call-to-Actions (CTAs) for Conversions: CTAs are a crucial part of any conversion funnel. Whether it’s a button on a landing page or a link in an email, the design, placement, and wording of your CTAs can significantly impact conversion rates. A/B testing allows marketers to experiment with different versions of CTAs, such as changing the color, text, or positioning, to determine which variation generates the most conversions. For instance, testing whether a more urgent phrase like “Get Started Now” outperforms a softer approach like “Learn More” can lead to actionable insights that improve conversion rates.
Testing Form Length and Complexity: Forms are often a barrier to conversions, especially if they are lengthy or complicated. A/B testing can help determine the optimal number of form fields, the placement of the form, and even the type of information requested. For example, testing a shorter form with only essential fields against a more detailed one can reveal which version leads to higher form completions. Reducing friction in the sign-up or checkout process can have a significant positive impact on conversion rates.
Experimenting with Landing Page Variations: A/B testing marketing strategies often focus on landing pages, as they are typically where conversions happen. Testing different layouts, imagery, headlines, and value propositions can help identify the design and messaging that resonates most with your audience. For instance, testing whether a landing page with a single clear CTA outperforms one with multiple options can guide you toward a more streamlined and effective conversion path.
Personalizing User Experiences: Personalization is becoming increasingly important in AB testing digital marketing. By leveraging user data to create personalized content, offers, and recommendations, you can enhance the relevance of your marketing. For example, testing personalized product recommendations against generic ones can show whether tailoring content to individual preferences boosts conversion rates.
Optimizing Pricing and Offers: Pricing and promotional offers are critical factors in conversion rates. A/B testing different pricing models, discount strategies, or payment plans can provide insights into what drives more purchases. For instance, testing whether a limited-time offer generates more urgency and conversions compared to a more permanent discount can guide your promotional strategies.
Lower Bounce Rate
A high bounce rate indicates that visitors are leaving a webpage shortly after arriving, often without taking any action or exploring further. In AB testing digital marketing, reducing bounce rates is a primary goal, as it directly correlates with improved user engagement, longer session durations, and ultimately better conversion rates.
Optimizing Page Layout and Content Structure: The way information is presented on a webpage greatly affects whether users stay or leave. By testing different page layouts, such as the placement of key elements like headers, CTAs, and images, marketers can determine which design retains visitors better. For example, testing whether a single-column layout with focused content outperforms a multi-column design can reveal which structure leads to lower bounce rates.
Enhancing Headline and Value Proposition: The headline is often the first element visitors notice, making it a crucial factor in retaining their attention. Through A/B testing, marketers can experiment with different headlines and messaging strategies to determine which variation resonates most with their audience. For instance, testing a headline that emphasizes benefits ("Save Time and Money with Our Solution") against one focused on features ("Advanced Automation Tools Available") can guide content optimization efforts.
Testing Navigation and User Flow: A poorly structured navigation system can frustrate users and cause them to bounce. A/B testing different navigation styles, such as sticky menus versus traditional dropdowns or simplified versus comprehensive options, can help identify what keeps users engaged and encourages further exploration. Testing these elements ensures that users can easily find what they’re looking for, reducing the likelihood of them leaving prematurely.
Improving Page Load Times: Page speed is a critical factor in reducing bounce rates. Slow-loading pages often lead to high bounce rates, especially on mobile devices. While AB testing website content, it’s also important to test page performance enhancements, such as image compression, lazy loading, and optimized scripts. By comparing user behavior on faster versus slower-loading versions of a page, marketers can quantify the impact of speed on bounce rates.
Tailoring Content for Audience Segments: Personalizing content for different audience segments is another effective way to reduce bounce rates. By using A/B testing to compare personalized content (e.g., dynamic text or tailored offers) against generic content, marketers can determine which approach better aligns with user expectations and keeps them engaged longer.
Perfect Product Images
Product images are one of the most influential factors in the decision-making process for e-commerce customers. Selecting the perfect product images that resonate with your audience can significantly enhance user engagement, build trust, and increase conversions. A/B testing in digital marketing is an invaluable tool for identifying which images work best for your products.
Testing Different Image Angles and Perspectives: The angle or perspective of a product image can influence how well it communicates key features. For example, in an e-commerce setting, testing a front-facing image against an angled shot or a close-up detail can reveal which view resonates more with potential buyers. A/B testing can help determine whether lifestyle images (products in use) or standard product shots drive more engagement and conversions.
Experimenting with Image Quality and Resolution: High-quality, sharp images often lead to better performance, but there’s a balance to be struck between quality and load time. Through A/B testing, marketers can evaluate whether higher-resolution images lead to increased sales despite potentially longer load times. This testing can also extend to image formats, such as comparing JPEGs to WebP formats, to see which provides the best combination of speed and clarity.
Testing Image Variants for Different Audiences: Different customer segments may respond better to different types of images. For example, testing images with models of varying demographics can reveal which photos appeal most to specific audience segments. By AB testing these image variants, marketers can personalize their visual content to better align with the preferences of their target audience, ultimately leading to improved engagement and conversions.
Comparing Image Backgrounds and Context: The background and context of a product image can impact its effectiveness. For instance, testing images with clean white backgrounds against those with contextual settings (e.g., a coffee cup in a kitchen setting) can reveal which approach drives better engagement. A/B testing different backgrounds, colors, and settings helps marketers identify the visual style that best conveys the product’s value and appeals to the target audience.
Evaluating the Impact of Image Size and Placement: The size and placement of product images on a webpage also play a crucial role in conversions. By testing large hero images versus smaller thumbnails or experimenting with image placements within product pages (e.g., above the fold versus within galleries), marketers can determine the optimal configuration that captures attention and drives purchasing decisions.
Lower Cart Abandonment
Cart abandonment is a significant challenge for e-commerce businesses, and reducing it is often a top priority in A/B testing digital marketing strategies. Cart abandonment occurs when users add products to their cart but leave the website without completing the purchase. This behavior can be influenced by various factors such as complex checkout processes, unexpected costs, or lack of trust. A/B testing allows marketers to identify and address these issues by experimenting with different elements of the checkout process to find the optimal configuration that encourages customers to complete their transactions.
Understanding Cart Abandonment
Before diving into the specifics of how A/B testing can reduce cart abandonment, it’s essential to understand why it happens. Some common reasons for cart abandonment include:
Unexpected shipping costs or additional fees
Complex or lengthy checkout processes
Lack of payment options
Mandatory account creation before checkout
Concerns about payment security
By focusing A/B testing in marketing efforts on these pain points, businesses can systematically reduce friction in the purchasing process and improve the overall user experience.
Key Areas to A/B Test for Reducing Cart Abandonment
Streamlining the Checkout Process
A cumbersome or multi-step checkout process is one of the most common reasons for cart abandonment. A/B testing on websites allows marketers to test different checkout flows, such as single-page checkouts versus multi-step checkouts. By comparing conversion rates between these variations, businesses can determine which checkout process leads to fewer drop-offs. For instance, testing whether reducing the number of form fields (e.g., removing optional fields) improves conversion rates can provide actionable insights into how to streamline the process.
Testing Payment Options and Methods
Limited payment options can deter customers from completing a purchase. A/B testing can be used to experiment with offering multiple payment gateways (e.g., PayPal, Apple Pay, credit cards) or introducing alternative payment methods like buy now, pay later (BNPL) services. By testing different combinations of payment options and analyzing their impact on conversion rates, businesses can identify the payment methods that most appeal to their target audience.
Optimizing Shipping Information and Costs
Unexpected shipping costs are a leading cause of cart abandonment. A/B testing different ways of displaying shipping information—such as showing estimated costs earlier in the process or offering free shipping thresholds—can help determine what encourages customers to proceed with their purchase. For example, testing a banner that highlights free shipping on orders over a certain amount against one that shows flat-rate shipping may reveal which approach is more effective at reducing abandonment.
Simplifying Account Creation Requirements
Forcing customers to create an account before purchasing is another common barrier that A/B testing in marketing can help address. By testing variations like guest checkout options versus mandatory account creation, businesses can determine which process leads to higher completion rates. Many customers prefer a quick checkout experience without the hassle of setting up an account, so offering a guest checkout option often results in a significant reduction in cart abandonment.
Enhancing Trust Signals and Security Features
Trust plays a critical role in whether a customer completes a purchase. A/B testing the inclusion of trust badges, SSL certificates, and secure payment seals in the checkout process can help determine which elements boost customer confidence. Testing different placements of these trust signals—whether it’s at the top of the checkout page, near the payment form, or in the footer—can reveal where they are most effective in reducing concerns and abandonment rates.
Testing Cart and Checkout Reminders
Cart abandonment emails and pop-up reminders are effective strategies to bring customers back. A/B testing the timing, messaging, and design of these reminders can help businesses identify the most compelling way to encourage users to return and complete their purchase. For instance, testing whether a reminder that includes a discount code or free shipping incentive outperforms a simple nudge can guide how to craft the most effective recovery strategy.
Optimizing Product Descriptions and Reviews at Checkout
Even during the checkout process, customers might hesitate due to lingering doubts about the product itself. A/B testing the inclusion of product descriptions, key benefits, or customer reviews within the checkout flow can help alleviate these doubts. By testing whether these additional details lead to a higher conversion rate, marketers can ensure that customers have all the information they need to feel confident in their purchase decision.
Reducing Distractions in the Checkout Process
Distractions like unnecessary navigation links, promotional banners, or unrelated offers can cause users to leave the checkout page. A/B testing variations that simplify the page layout—by removing excess elements and focusing solely on the transaction—can lead to better results. Testing a minimalistic design against one with more distractions can highlight the best approach to keep users focused on completing their purchase.
How to Implement A/B Testing for Cart Abandonment
When implementing A/B testing for websites aimed at reducing cart abandonment, it’s crucial to follow a structured approach:
Identify Key Metrics: Focus on metrics such as cart abandonment rate, checkout completion rate, and overall conversion rate.
Create Hypotheses: Based on user data and behavior analysis, develop hypotheses on why customers are abandoning their carts and how changes could improve the process.
Design Variations: Create the different versions (A and B) for the checkout process, payment methods, or other elements you want to test.
Run the Test: Use A/B testing tools to split traffic evenly between the control and variation, ensuring a statistically significant sample size for accurate results.
Analyze Results: Compare the performance of each variation based on your predefined metrics. Implement the winning version and continue testing new hypotheses for further optimization.
Core Components of A/B Testing
A/B testing is a powerful methodology in digital marketing that allows businesses to make data-driven decisions by comparing two variations of a web page, email, ad, or other marketing elements to determine which performs better. Understanding the core components of A/B testing is essential for designing and executing successful experiments that yield actionable insights. This approach is widely used in AB testing marketing strategies to optimize conversion rates, improve user experience, and enhance the effectiveness of marketing campaigns.
1. Hypothesis Formulation
Before conducting an A/B test, it’s crucial to define a clear hypothesis. The hypothesis sets the foundation for the experiment by identifying what you want to test, why you’re testing it, and what results you expect. A well-defined hypothesis might look like, “Changing the color of the call-to-action (CTA) button from blue to red will increase the click-through rate by 15%.” This statement provides a specific, testable prediction that can be validated through experimentation.
In A/B testing digital marketing, the hypothesis is based on insights from user behavior data, historical performance metrics, or UX best practices. A strong hypothesis directly addresses the problem or objective you aim to solve, guiding the direction of your AB testing methodology.
2. Control and Variation
In A/B testing, the two primary components are the control (Version A) and the variation (Version B). The control is the original version of the element you’re testing, while the variation introduces a single change designed to improve performance. For example, if you’re testing a landing page, the control might feature a blue CTA button, while the variation might use a red button.
The goal is to isolate one change in the variation so that any differences in performance can be attributed directly to that change. By keeping all other elements constant, A/B testing ensures that you can identify the impact of the tested variable with clarity and precision.
3. Audience Segmentation and Traffic Distribution
Audience segmentation and traffic distribution are critical in AB testing in digital marketing. The audience for the test is typically split randomly into two groups: one group is shown the control, and the other is shown the variation. Randomization helps eliminate biases and ensures that the test results are statistically valid.
Traffic distribution can be adjusted depending on the nature of the test and the risk associated with making changes. For example, in high-stakes tests, marketers might start by directing only a small portion of traffic to the variation before scaling up. A/B testing tools like Google Optimize and Optimizely allow marketers to control traffic distribution, making it easier to manage how many users see each version.
4. Key Metrics and Goals
Every A/B test should have clearly defined metrics and goals that determine success. These metrics should align with your overall business objectives, such as increasing conversion rates, reducing bounce rates, or improving user engagement. Typical metrics in A/B testing marketing include:
Conversion Rate: The percentage of users who complete a desired action, like signing up for a newsletter or making a purchase.
Click-Through Rate (CTR): The percentage of users who click on a link, button, or CTA.
Bounce Rate: The percentage of users who leave a webpage after viewing only one page.
Choosing the right metrics is crucial for measuring the impact of the changes being tested. These metrics should be closely monitored throughout the test to track performance and determine when a variation has produced statistically significant results.
5. Statistical Significance and Sample Size
Statistical significance is a core component of A/B testing methodology. It indicates how confident you can be that the results of your test are not due to random chance. To achieve statistical significance, you need to gather enough data (sample size) to ensure that the observed differences between the control and variation are meaningful.
Determining the appropriate sample size is critical. Too small a sample size can lead to misleading results, while too large a sample size can waste time and resources. A/B testing tools often include calculators that help determine the required sample size based on your desired confidence level and minimum detectable effect.
6. Test Duration and Timing
The duration of an A/B test plays a significant role in ensuring reliable results. The test should run long enough to account for normal variations in user behavior, such as differences between weekdays and weekends or seasonal fluctuations. Prematurely ending a test can lead to incorrect conclusions, while running it too long can delay decision-making.
It’s generally recommended to let the test run until you reach a predefined sample size and confidence level. The timing of the test is also important; launching a test during an unusual period (e.g., a holiday sale) can skew the results, leading to inaccurate insights.
7. Analyzing Results and Drawing Conclusions
Once the test is complete, the next step is to analyze the results to determine which version performed better. In A/B testing in marketing, this analysis involves comparing key metrics between the control and variation, considering factors like statistical significance, and identifying the winning version.
The insights gained from the test should be used to inform future marketing strategies and optimizations. Even if the variation doesn’t outperform the control, the results provide valuable insights into user behavior and preferences. Continuous A/B testing allows for incremental improvements that, over time, lead to significant gains in performance.
8. Implementation and Iteration
After identifying the winning variation, it’s essential to implement the changes across your marketing assets. A/B testing is an iterative process; once a test is completed, the insights gained often lead to new hypotheses and further testing opportunities. This continuous cycle of testing, analyzing, and optimizing is what drives sustained growth and improvement in AB testing digital marketing.
How to Run a Basic A/B Test?
A/B testing is an essential practice in digital marketing that allows businesses to make data-driven decisions by comparing two versions of a webpage, email, or ad to determine which performs better. Understanding how to run an A/B test effectively is crucial for optimizing conversion rates, improving user experience, and enhancing overall marketing performance. This guide covers the fundamental steps to conducting a successful A/B test.
Look for Improvement Opportunities
The first step in conducting A/B testing in marketing is to identify areas where improvements can be made. This typically involves analyzing existing performance data and pinpointing elements that are underperforming or could be optimized further. In AB testing digital marketing, improvement opportunities often come from high-impact areas such as landing pages, email campaigns, or product pages.
Common Improvement Areas:
Landing Page Optimization: If a landing page has a high bounce rate or low conversion rate, that’s a clear indicator that something can be improved. Key elements to focus on include headlines, CTA buttons, form fields, and content structure.
Email Campaigns: Low open rates or click-through rates in email campaigns suggest that subject lines, preview text, or email content may need to be tested and optimized.
Ad Performance: In paid advertising, low CTRs or high cost-per-click (CPC) can indicate that your ad copy, images, or targeting strategy needs refinement.
Tools like Google Analytics, HubSpot, or heat mapping software can provide valuable insights into user behavior and highlight where your audience is dropping off. For instance, if data shows that users abandon your page during the checkout process, that’s a prime area for testing and improvement.
By focusing on areas where you’re not achieving desired results, you can target your A/B testing efforts more effectively, leading to better outcomes.
Identify a Variable
Once you’ve identified where improvements are needed, the next step is to pinpoint the specific variable you want to test. The success of A/B testing in digital marketing hinges on isolating one element at a time, which allows you to accurately determine the impact of that specific change. Testing multiple variables simultaneously can lead to inconclusive results because you won’t know which change affected the outcome.
Common Variables to Test:
Headlines and Copy: Testing different headlines or messaging strategies can reveal which approach resonates most with your audience. For example, a headline that focuses on benefits ("Save Time with Our Tool") might perform better than one that emphasizes features ("Advanced Features for Productivity").
Call-to-Action (CTA) Buttons: CTAs are crucial for driving conversions. Testing variables such as button color, size, text, and placement can help you determine the most effective configuration. For instance, testing whether a prominent “Sign Up Now” button outperforms a more subtle “Learn More” button can provide insights into user preferences.
Form Length and Fields: For lead generation pages, the number of fields in a form can impact conversion rates. Testing shorter forms against longer ones can reveal the optimal balance between collecting enough information and maintaining user engagement.
Page Layout and Visual Elements: Testing different layouts, such as single-column versus multi-column designs, or experimenting with image placement can enhance user experience and reduce bounce rates.
After identifying the variable, clearly define both the control (Version A) and the variation (Version B). For example:
Control (Version A): The original landing page with a blue CTA button.
Variation (Version B): A new landing page with an orange CTA button.
The goal is to change only one element at a time so that any difference in performance can be attributed directly to that change.
Settle on a Test Hypothesis
The hypothesis is the foundation of your A/B test. It provides a clear direction for your experiment by defining what you want to test, why you are testing it, and what outcome you expect. A strong hypothesis is not just a guess; it’s a statement based on data, user behavior, or observed trends that can be tested in a controlled environment.
How to Formulate a Strong Hypothesis
When setting a hypothesis in A/B testing in marketing, consider the following:
Identify the Problem: Start by pinpointing an issue or area for improvement, such as a low conversion rate or high bounce rate.
Propose a Solution: Your hypothesis should propose a specific change aimed at improving the identified issue. For example, “Changing the color of the call-to-action (CTA) button from blue to green will increase click-through rates by 20%.”
Anticipate the Outcome: Predict the result of the change. This prediction will guide how you measure success. In this case, the success metric would be an increase in clicks on the CTA.
An effective hypothesis for AB testing digital marketing could look like this:
Hypothesis Example: "If we change the headline on our landing page to focus more on customer benefits rather than features, the conversion rate will increase by 15%."
The hypothesis serves as the benchmark for the test. If the variation outperforms the control, the hypothesis is confirmed; if not, it might need to be revised or tested further.
Set Your Goals, Test Period, and Sample Size
Once you have your hypothesis, the next step in A/B testing is to define clear goals, determine the test period, and calculate the appropriate sample size. These elements are crucial for ensuring that your test results are statistically significant and actionable.
1. Define Your Goals
Your goals should align directly with the hypothesis and address the specific metric you aim to improve. Typical goals in A/B testing marketing include:
Increase Conversion Rates: Such as sign-ups, purchases, or form completions.
Improve Click-Through Rates (CTR): For buttons, links, or ad elements.
Reduce Bounce Rates: By optimizing landing page elements.
For example, if your hypothesis is focused on improving the CTA button’s performance, the primary goal might be to increase the click-through rate by a certain percentage. Ensure your goals are specific, measurable, and directly tied to the test's success.
2. Determine the Test Period
The length of your test is critical. Running the test for too short a period can lead to unreliable data, while running it too long can waste resources. The duration of the test should account for daily and weekly variations in user behavior, such as changes in traffic on weekdays versus weekends.
AB testing methodology suggests that a test should run until it reaches statistical significance. A common guideline is to run the test for at least one full business cycle (e.g., a week or month) to capture the full range of user behaviors.
Short-Term Tests: Useful for high-traffic websites where results can be quickly gathered.
Longer-Term Tests: Necessary for lower-traffic sites where more time is needed to gather enough data.
3. Calculate the Sample Size
To achieve statistically significant results, it’s important to test your control and variation on a large enough sample size. If the sample size is too small, the results may not be reliable, leading to incorrect conclusions. Tools like A/B sample size calculators can help determine how many visitors are needed for each version to reach statistical significance.
When calculating sample size, consider:
Baseline Conversion Rate: The current conversion rate helps estimate the sample size required to detect a meaningful change.
Minimum Detectable Effect: The smallest improvement you would consider a success, often expressed as a percentage change.
Confidence Level: Typically set at 95%, this indicates how sure you want to be that your results are not due to random chance.
In A/B testing for websites, a test might require thousands of visitors per version to achieve meaningful insights, depending on the expected effect size.
Implementing the Test
With your hypothesis, goals, test period, and sample size defined, you’re ready to implement your A/B test. Use A/B testing tools like Google Optimize, Optimizely, or HubSpot to evenly split traffic between your control and variation while monitoring key performance indicators.
Create Variations Based on Your Hypothesis
Once you have defined a clear hypothesis, the next step in the A/B testing methodology is to create variations that will test your hypothesis. In AB testing digital marketing, the control (Version A) represents the original version of the webpage, email, or ad, while the variation (Version B) is a modified version based on your hypothesis.
Steps to Creating Effective Variations
Focus on One Variable: The essence of A/B testing is to isolate and test one variable at a time. For example, if your hypothesis suggests that changing the color of your CTA button from blue to orange will improve conversion rates, that should be the only difference between the control and the variation. Testing multiple changes simultaneously can lead to inconclusive results, as you won’t be able to determine which specific change influenced the outcome.
Align Variations with Your Hypothesis: Ensure that the variation directly reflects the change proposed in your hypothesis. For instance, if your hypothesis states that rewording your headline to emphasize benefits instead of features will increase engagement, create a variation that introduces this exact change while keeping all other elements constant.
Design and Implement Variations with Consistency: Use A/B testing tools like Google Optimize, Optimizely, or HubSpot to easily create and manage variations. These tools provide user-friendly interfaces that allow you to modify design elements, copy, and layouts while ensuring the rest of the page remains unchanged. For AB testing websites, consistency is key, as this prevents external factors from affecting the results.
Preview and Test for Errors: Before launching the test, thoroughly review both the control and variation for errors. Small issues like broken links, incorrect images, or formatting problems can skew results and invalidate your test. A clean, consistent setup is essential for accurate data collection.
Run Your Test
After creating the variations, the next step in A/B testing in marketing is to run the test. This stage is crucial for gathering the data needed to determine which version performs better. Running the test requires careful planning and attention to several factors, including sample size, traffic distribution, and test duration.
Steps to Running a Successful A/B Test
Split Your Audience Evenly: The audience should be divided randomly and evenly between the control and variation. In A/B testing, randomization helps eliminate biases and ensures that each version is exposed to a representative sample of your target audience. Most A/B testing tools automate this process, allowing you to control how traffic is distributed. For example, 50% of visitors see Version A, and the other 50% see Version B.
Determine the Test Duration: The test duration depends on your traffic volume and the changes being tested. Running the test for too short a period can lead to misleading results, while running it too long can introduce external variables, such as seasonal changes, that might affect the outcome. A typical A/B testing methodology recommends running the test until it reaches statistical significance, ensuring that the results are reliable. Generally, you should aim for a confidence level of at least 95% before drawing any conclusions.
Monitor the Test in Real Time: During the test, monitor key metrics in real-time using your chosen A/B testing tool. While it’s important to let the test run without interference, tracking performance metrics like click-through rates, conversion rates, and bounce rates can help you identify any unexpected issues. However, avoid making changes mid-test, as this can introduce biases and invalidate your results.
Analyze Test Data for Statistical Significance: Once the test is complete, the data should be analyzed to determine which version performed better. Statistical significance is key here; it indicates whether the observed differences between the control and variation are likely due to the change you made rather than random chance. Most testing platforms calculate statistical significance for you, providing confidence levels that guide your decision-making.
Interpret the Results and Implement the Winning Version: After the test reaches statistical significance, the next step is to implement the winning version across your marketing assets. Whether the control or variation wins, the insights gained from the test should inform future optimizations. For example, if the variation significantly outperformed the control, you can confidently roll out that change across your website, email campaigns, or ads.
Plan for Continuous Testing and Iteration: A/B testing in marketing is an ongoing process. Once one test is complete, use the insights to formulate new hypotheses and run additional tests. This iterative approach allows for continuous improvement, leading to better overall marketing performance and user experience.
Analyze the Results and Plot Your Next Steps
The analysis stage is where the value of A/B testing in marketing truly becomes apparent. After your test has run for an appropriate duration and collected sufficient data, the next step is to evaluate the performance of the control (Version A) against the variation (Version B). The goal is to identify whether the changes you made had a statistically significant impact on the key metrics you defined at the beginning of the test.
Key Steps in Analyzing A/B Test Results:
Check for Statistical Significance: Before drawing any conclusions, it’s essential to ensure that the results are statistically significant. Statistical significance indicates that the observed difference between the control and variation is unlikely to have occurred by chance. Most A/B testing tools automatically calculate this for you, providing a confidence level—typically set at 95%—that shows how confident you can be in the results. If the confidence level is below 95%, it’s generally recommended to continue the test until you reach this threshold.
Evaluate Performance Metrics: Review the primary metrics you set for the test, such as conversion rate, click-through rate (CTR), or bounce rate. Compare the performance of the control and variation to see if the hypothesis was validated. For example, if you were testing a new CTA design, did the variation lead to more conversions? If so, by how much? It’s important to consider both absolute numbers and percentage changes when analyzing the results.
Look Beyond the Primary Metric: While the primary metric is key, don’t overlook secondary metrics that can offer additional insights. For instance, an increase in conversions may be accompanied by changes in user behavior elsewhere, such as increased page engagement or lower bounce rates. Understanding these related metrics can give you a more holistic view of how the change impacted user experience.
Segment Your Data: In A/B testing digital marketing, it’s often useful to segment the results by audience characteristics, such as device type, geographic location, or traffic source. A change that works well for one segment might not perform as well for others. Analyzing results by segment can uncover deeper insights and reveal opportunities for further optimization.
Identify Patterns and Anomalies: As you review the data, look for patterns that support or contradict your hypothesis. Also, be mindful of anomalies—unexpected spikes or dips in performance that could indicate external factors influencing the results. Understanding these nuances can help refine future tests.
Plot Your Next Steps
Once you’ve analyzed the data, the next phase is deciding on the course of action. The insights gained from A/B testing in digital marketing should directly influence your next steps, whether it’s implementing the winning variation or iterating on your strategy for further optimization.
Key Steps in Planning Your Next Actions:
Implement the Winning Variation: If the variation outperformed the control with statistical significance, the next logical step is to implement the winning version across your marketing assets. For example, if a new headline increased conversion rates, update all relevant pages, emails, or ads with the improved version.
Iterate and Test Further: A/B testing is an iterative process. Even after a successful test, there’s always room for further refinement. Use the insights gained to form new hypotheses and plan the next round of tests. For example, if a CTA color change worked well, the next test could focus on the CTA’s text or placement. Continuous testing leads to incremental improvements, which over time, significantly enhance performance.
Document the Results: Keeping a detailed record of each A/B test is critical for future reference. Document the hypothesis, metrics, results, and conclusions, as well as any insights gained. This creates a knowledge base that can guide future tests and help avoid repeating tests with similar hypotheses.
Communicate the Findings: Share the results and insights with your team. This helps align marketing, product, and design teams around what works and what doesn’t. Clear communication ensures that everyone is on the same page and can incorporate these learnings into their work.
Consider Broader Applications: Sometimes, the insights from a single A/B test can be applied more broadly across different channels or campaigns. For instance, if a particular messaging strategy performs well in an email test, you might consider applying it to your website copy or ad campaigns.
Monitor Long-Term Impact: After implementing the winning variation, it’s essential to monitor its performance over time. In some cases, the initial uplift might taper off, or new changes in user behavior might necessitate further adjustments. Regular monitoring ensures that the improvements are sustained and continue to deliver value.
What Elements to A/B Test?
A/B testing is a powerful strategy in digital marketing that allows businesses to optimize their content and campaigns by comparing two variations of a single element. The key to successful A/B testing in marketing lies in choosing the right elements to test. By identifying which components have the greatest impact on user engagement, conversions, and overall performance, you can make data-driven decisions that enhance your marketing strategy. Below, we explore the most critical elements to consider when conducting an A/B test.
Headlines
Headlines are one of the most crucial elements in A/B testing marketing because they are often the first thing users see. A compelling headline can grab attention, engage visitors, and encourage them to explore further. Conversely, a weak headline can lead to high bounce rates and missed opportunities. In AB testing digital marketing, headlines are frequently tested to determine which messaging resonates best with the target audience.
Key Aspects to Test in Headlines:
Tone and Style: Different audiences respond to different tones. For example, a more formal and authoritative headline might work better for B2B marketing, while a casual and conversational tone might resonate with B2C audiences. A/B testing can reveal which tone drives more engagement.
Example Test: "Boost Your Business with Our Software" (formal) vs. "Ready to Grow? Let’s Do It Together" (conversational).
Value Proposition: Headlines that clearly communicate the benefits of your product or service often perform better. Testing variations that emphasize the value you offer can help determine the most effective messaging.
Example Test: "Save Time with Our Automation Tool" vs. "Increase Efficiency with Automated Workflows".
Length and Structure: The length of the headline can influence how well it captures attention. Short, punchy headlines might work well in some contexts, while longer, more descriptive headlines could be more effective in others.
Example Test: "Simplify Your Marketing" vs. "Simplify Your Marketing with AI-Driven Solutions".
Keywords and Phrasing: Including relevant keywords in your headlines can improve SEO and align with what users are searching for. Testing different keyword combinations can help enhance both organic reach and engagement.
Example Test: "Marketing Automation Software" vs. "Best Marketing Automation Tool for Small Businesses".
In A/B testing on websites, experimenting with headlines can lead to a significant improvement in metrics such as click-through rates (CTR), bounce rates, and conversion rates. The headline sets the stage for user experience, so optimizing it through rigorous testing is critical.
Call to Action
The call to action (CTA) is another vital element in A/B testing. CTAs guide users toward completing a desired action, whether it’s signing up for a newsletter, making a purchase, or downloading content. Testing different aspects of your CTAs can significantly impact conversion rates.
Key Aspects to Test in CTAs:
Text and Messaging: The language used in your CTA plays a major role in driving action. Testing variations that range from direct commands to more subtle suggestions can help you identify what drives the most conversions.
Example Test: "Get Started Now" vs. "Learn More" vs. "Try for Free".
Color and Design: Visual elements like the color, size, and shape of your CTA button can influence user behavior. Bright, contrasting colors typically draw more attention, but the ideal color scheme should align with your brand while still standing out.
Example Test: A green CTA button vs. a red CTA button.
Placement and Positioning: The placement of the CTA on your webpage can be critical. Testing whether a CTA performs better above the fold (where users see it immediately) or below the fold (after they’ve engaged with the content) is a common approach in A/B testing in marketing.
Example Test: A CTA placed at the top of a landing page vs. one positioned at the end of a long-form content piece.
Personalization: Personalized CTAs, tailored based on user behavior, preferences, or demographics, can lead to higher conversion rates. Testing different levels of personalization in your CTAs can help determine the best approach for your audience.
Example Test: "Start Your Free Trial" vs. "Start Your Free Trial, [User’s Name]".
Urgency and Scarcity: Adding elements of urgency or scarcity can prompt users to take immediate action. Testing CTAs that incorporate phrases like "Limited Time Offer" or "Only 3 Spots Left" can boost conversions by creating a sense of urgency.
Example Test: "Claim Your Offer" vs. "Claim Your Offer – Expires Today".
The goal of A/B testing CTAs is to optimize them for maximum impact. Since CTAs are directly tied to conversions, even minor improvements can lead to significant gains in revenue and user engagement.
Email Subject Lines
Email subject lines are one of the most critical factors in determining whether recipients open an email. In A/B testing in digital marketing, subject lines are frequently tested to improve open rates and, subsequently, click-through rates. The subject line is the first impression, so optimizing it can lead to higher engagement and better overall email campaign performance.
Key Aspects to Test in Email Subject Lines:
Length and Word Count: The length of your subject line can impact how it’s perceived. Short and concise subject lines may catch attention quickly, while longer ones can provide more context. Testing different lengths helps identify which works best for your audience.
Example Test: "Get 20% Off Today" vs. "Special Offer: Save 20% on Your Next Purchase with Us Today!"
Personalization: Personalized subject lines that include the recipient’s name or other tailored details often perform better. Testing variations that include personalized content versus generic messaging is a typical use in A/B testing marketing.
Example Test: "John, Your Exclusive Deal Awaits!" vs. "Your Exclusive Deal Awaits!"
Tone and Messaging: The tone of your subject line can be critical, depending on your brand and audience. A/B testing can help determine whether a formal, professional tone or a friendly, conversational tone drives better open rates.
Example Test: "Last Chance to Register for Our Webinar" vs. "Don't Miss Out – Register Now!"
Use of Emojis and Symbols: Incorporating emojis or special characters can make your subject lines stand out in a crowded inbox, but they may not resonate with every audience. Testing subject lines with and without emojis can reveal which approach yields better results.
Example Test: "🔥 Big Savings Inside!" vs. "Big Savings Inside!"
Urgency and Scarcity: Adding elements of urgency (e.g., “Limited Time Offer”) can encourage recipients to act quickly. A/B testing different levels of urgency can help identify which messaging drives more immediate engagement.
Example Test: "Only 24 Hours Left to Save!" vs. "Last Chance to Get 50% Off!"
Optimizing email subject lines through A/B testing in marketing can lead to higher open rates, better engagement, and more conversions, making it a crucial component of any email marketing strategy.
Layout and Navigation
The layout and navigation of your website directly impact how users interact with your content and ultimately determine whether they convert. In A/B testing digital marketing, testing different layouts and navigation structures is essential for improving user experience, reducing bounce rates, and driving conversions.
Key Aspects to Test in Layout and Navigation:
Menu Structure and Placement: The way your website’s menu is organized can significantly influence user behavior. Testing different menu structures, such as horizontal versus vertical navigation or simplifying versus expanding menu options, can help determine what makes it easier for users to find what they need.
Example Test: A simple menu with a few core categories vs. a detailed menu with multiple subcategories.
Content Layout: The layout of your website’s pages—especially key landing pages—affects how users engage with your content. Testing single-column versus multi-column layouts, as well as the placement of important information (e.g., placing CTAs above the fold versus below), is a common strategy in A/B testing on websites.
Example Test: A product page with large images and minimal text vs. a page with more detailed descriptions and smaller images.
Visual Hierarchy: Testing how you structure visual elements on a page, such as images, headlines, and CTAs, can reveal the most effective way to guide users toward desired actions. A well-organized hierarchy can reduce friction and improve conversion rates.
Example Test: A layout with a prominent hero image and centered CTA vs. a layout with text-focused content and a side CTA.
Simplified Navigation vs. Comprehensive Navigation: Some users prefer straightforward, minimalistic navigation, while others appreciate more detailed menus that allow them to explore deeper. A/B testing methodology can help identify which approach works better for your audience.
Example Test: A streamlined homepage with limited navigation options vs. a homepage featuring a comprehensive, detailed navigation bar.
Mobile vs. Desktop Navigation: Testing how navigation behaves across devices is crucial for optimizing the user experience. Different navigation styles might be more effective depending on whether the user is accessing your site on a mobile device or a desktop.
Example Test: A sticky header navigation on mobile vs. a collapsible menu that only expands when clicked.
Optimizing layout and navigation through A/B testing in marketing can dramatically improve user experience, leading to lower bounce rates, longer session durations, and higher conversion rates.
Social Proof
Social proof is a critical element in A/B testing digital marketing strategies because it directly impacts how much trust your audience places in your brand. Social proof includes testimonials, reviews, ratings, case studies, and customer logos that signal credibility and influence purchasing decisions. In A/B testing marketing, optimizing the presentation, placement, and content of social proof can significantly enhance conversion rates and build trust among potential customers.
Social proof serves as a powerful persuasion tool. When users see that others have had positive experiences with a product or service, they are more likely to feel confident in making a purchase or engaging with your brand. Testing how you present social proof on your website or marketing materials can reveal what resonates most with your audience and ultimately drives better results. By strategically experimenting with different types of social proof, you can determine the most effective ways to increase trust and conversions.
Key Aspects of Social Proof to A/B Test
Types of Social Proof Displayed
In AB testing in digital marketing, you can experiment with different kinds of social proof to see which has the greatest impact. The types of social proof include:
Customer Testimonials: Direct quotes from satisfied customers.
Star Ratings and Reviews: User ratings and reviews, often displayed on product pages or landing pages.
Case Studies: In-depth examples of how your product or service has benefited other businesses or individuals.
Customer Logos: Displaying well-known brands that use your product can add credibility.
Testing different combinations of these elements can reveal which type of social proof resonates best with your audience and boosts conversion rates.
Placement and Positioning
Where you place social proof on your website or landing pages can greatly influence its effectiveness. Testing different placements is a typical use of A/B testing in marketing. For example:
Above the Fold vs. Below the Fold: Placing social proof above the fold can catch the user’s attention immediately, while positioning it below the fold can serve as reinforcement after they’ve engaged with your content.
Sidebar vs. Inline Content: Testing whether social proof performs better as part of the main content or in a sidebar can help determine the ideal placement for your audience.
Checkout Pages and Product Pages: Including social proof on key conversion points like checkout or product pages can reassure potential buyers and reduce friction.
Content Format and Style
The format and style of social proof content play a crucial role in how persuasive it is. A/B testing can help determine whether text, video testimonials, or image-based reviews work best. Testing variables include:
Text vs. Video: Does your audience respond more to written testimonials or video case studies?
Detailed Reviews vs. Short Quotes: Longer, detailed testimonials may provide more convincing proof, but they could also overwhelm users. Testing these variations helps you find the right balance.
Single vs. Multiple Reviews: Displaying a single, impactful testimonial versus a carousel of reviews can have different effects depending on your target audience’s preferences.
Design Elements and Visual Cues
Design elements like color, typography, and icons can enhance or detract from the effectiveness of social proof. In A/B testing methodology, testing the visual presentation of social proof is essential to determine what grabs attention and communicates trust. Consider testing:
Emphasis on Ratings: Highlighting star ratings with bold colors or larger icons to make them more noticeable.
Trust Badges and Icons: Adding icons like “Verified Purchase” or trust badges alongside reviews.
Customer Photos: Including images of the customers providing testimonials can add a layer of authenticity.
Contextual Integration
The context in which social proof is presented can affect its impact. For example, A/B testing how customer logos or case studies are integrated within the content versus in isolation can provide insights into the best approach. Testing different copy that surrounds social proof, such as introducing it with phrases like “Our customers love us!” versus simply listing the reviews, can influence how it’s perceived.
Relevance and Specificity
The relevance of social proof to the user’s needs can also be tested. For instance, featuring testimonials from customers who share similar pain points or use cases as your target audience may have a greater impact than generic positive feedback. In A/B testing for websites, testing customer quotes that are industry-specific or address particular objections can help refine your approach.
How to A/B Test Social Proof
Define Your Hypothesis: Start with a clear hypothesis. For example, “Including customer testimonials above the fold will increase the conversion rate by 10%.”
Create Variations: Design different versions of the page with varying placements, formats, and types of social proof. For example, one variation might feature video testimonials above the fold, while another includes customer logos in a sidebar.
Split Traffic and Run the Test: Use an A/B testing tool like Google Optimize, Optimizely, or HubSpot to split traffic between the control and variations. Monitor how each performs in terms of key metrics like conversion rate, bounce rate, and time on page.
Analyze Results and Iterate: Once the test reaches statistical significance, analyze which variation performed best. If a specific approach outperforms others, implement it across your marketing assets. Continue testing different elements to refine your use of social proof.
A/B Testing Best Practices
A/B testing is a critical component of digital marketing that allows businesses to make data-driven decisions by comparing two variations of a webpage, email, or other marketing elements. However, running effective A/B tests requires careful planning, execution, and analysis. By following best practices, marketers can ensure they gather reliable data and generate actionable insights that drive meaningful improvements. Below are some key best practices for A/B testing in digital marketing.
Segment Your Audience Appropriately
Effective A/B testing digital marketing involves not only splitting your traffic evenly between the control and variation but also considering how different audience segments might respond. Segmenting your audience allows you to uncover deeper insights and tailor your strategies to different user groups, such as those based on demographics, behavior, or device type.
Why Audience Segmentation Matters
Different segments of your audience may respond differently to changes depending on their needs, preferences, or behaviors. For example, mobile users might engage more with a simplified CTA, while desktop users might prefer detailed information before taking action. By segmenting your audience during A/B testing, you can:
Identify which variations work best for specific groups.
Personalize marketing strategies based on segment-specific insights.
Avoid drawing generalized conclusions from results that might only apply to one segment.
Best Practices for Segmenting Audiences in A/B Testing:
Segment by Device Type: Mobile, tablet, and desktop users often behave differently. Test how each segment responds to variations like page layouts, load times, and navigation options.
Segment by Traffic Source: Users arriving via organic search, paid ads, social media, or email campaigns may have different intents. Segmenting by traffic source helps you understand how variations perform based on where users come from.
Segment by Demographics or Behavior: Personalizing tests based on user demographics (e.g., age, gender, location) or behavior (e.g., new vs. returning users) allows for more nuanced insights and targeted optimizations.
By tailoring your A/B testing methodology to different audience segments, you gain more precise data, leading to more impactful marketing decisions.
Ensure Statistical Significance
In A/B testing in digital marketing, statistical significance is a critical factor that determines whether the results of your test are valid and can be trusted. Achieving statistical significance means that the differences observed between the control and variation are unlikely to be due to random chance.
The Importance of Statistical Significance
Without statistical significance, your A/B test results may be unreliable, leading to inaccurate conclusions and misguided optimizations. For example, if you make a change based on inconclusive results, you risk implementing a variation that doesn’t actually perform better, which can negatively impact your overall marketing strategy.
Best Practices for Ensuring Statistical Significance:
Calculate Sample Size in Advance: Before launching your test, use a sample size calculator to determine the number of visitors needed to achieve statistical significance. This ensures you collect enough data to confidently measure differences between the control and variation.
Allow the Test to Run Long Enough: One common mistake in A/B testing marketing is ending the test too early. Running the test for a full business cycle (e.g., a week or month) accounts for natural fluctuations in user behavior and ensures that the data is comprehensive and representative.
Use a High Confidence Level: Typically, a confidence level of 95% is used in A/B testing methodology. This means you can be 95% confident that the results are not due to chance. If your confidence level is lower, consider extending the test until you reach the desired threshold.
Monitor and Validate Your Data: Continuously monitor your test data for consistency. Abrupt spikes or dips in performance could indicate external factors influencing your results, and it’s essential to account for these when analyzing the data.
By ensuring your test results reach statistical significance, you can make informed decisions and avoid costly mistakes in your digital marketing strategy.
Test Only One Variable at a Time
To accurately identify what drives changes in performance, it’s essential to focus on a single variable in each A/B test. Testing multiple variables simultaneously can lead to inconclusive results, as it becomes difficult to determine which change caused the observed effect.
The Role of Single-Variable Testing in A/B Testing
When you test just one element—such as a headline, CTA, or layout—any differences in performance between the control and variation can be confidently attributed to that change. This level of clarity is crucial for refining your marketing strategies incrementally and continuously improving your results.
Best Practices for Single-Variable Testing:
Start with High-Impact Elements: Focus on variables that are most likely to influence key metrics like conversion rate, click-through rate, or engagement. Common elements to test include headlines, CTA buttons, form fields, and landing page layouts.
Run Sequential Tests: Once you’ve identified the winning variation from one test, use that as the new control and run additional tests on other elements. This iterative approach allows for gradual but consistent optimization over time.
Avoid Multivariate Testing Unless Necessary: Multivariate testing (testing multiple elements at once) can be useful but requires significantly more traffic and sophisticated analysis. For most businesses, single-variable A/B tests are more manageable and yield clearer insights.
Document Each Change and Result: Carefully track each test, including the variable tested, the hypothesis, and the results. This documentation helps you understand which changes were most effective and prevents you from repeating similar tests unnecessarily.
By limiting each A/B test to one variable, you simplify the testing process, reduce the risk of inconclusive results, and gain more precise insights into what drives performance improvements.
Common A/B Testing Mistakes
A/B testing is an essential technique in digital marketing for optimizing conversion rates, improving user experiences, and driving better outcomes through data-driven decisions. However, many marketers make mistakes that can lead to misleading results, wasted resources, and flawed conclusions. Understanding and avoiding these common pitfalls is crucial to running successful A/B tests. Below are some of the most frequent mistakes in A/B testing in marketing and how to avoid them.
Testing Too Many Variables Simultaneously
One of the most frequent errors in A/B testing digital marketing is trying to test multiple variables at once. Marketers are often tempted to change several elements—such as the headline, CTA, and layout—all within a single test. While this approach might seem efficient, it usually results in inconclusive or misleading data.
Why Testing Too Many Variables is a Problem
When you test multiple variables simultaneously, it becomes difficult to determine which specific change led to the observed results. For example, if you change both the color of a CTA button and the headline text, and you see an increase in conversions, you won’t know whether the color change, the text change, or the combination of both was responsible for the improvement. This lack of clarity can lead to false assumptions and ineffective optimizations.
Typical use of A/B testing in marketing involves isolating a single variable at a time, allowing you to accurately attribute performance changes to the specific element being tested. This focused approach ensures that your findings are clear, actionable, and reliable.
Best Practices for Testing One Variable at a Time
Start with High-Impact Variables: Prioritize testing elements that are likely to have the biggest impact on user behavior, such as headlines, CTAs, or page layouts. For instance, in AB testing on websites, testing the headline or the main image can often lead to significant improvements in engagement or conversions.
Use Sequential Testing: If you want to test multiple variables, run separate A/B tests in sequence. For example, test the CTA button color first, and once you find the winning variation, move on to testing the headline. This step-by-step approach prevents overlapping influences and keeps your data clean.
Document Each Change and Its Impact: Keeping a detailed record of each test—including what was changed, the hypothesis, and the results—ensures that you can build on your findings over time. This documentation is especially helpful when running multiple tests across different elements.
Not Giving Your Tests Enough Time to Run
Another common mistake in A/B testing marketing is ending tests too early. Marketers may be eager to declare a winner based on early results, but doing so can lead to incorrect conclusions and wasted resources.
Why Ending Tests Early is a Problem
A/B testing methodology relies on gathering enough data to reach statistical significance. If you stop a test prematurely, you risk making decisions based on temporary fluctuations or insufficient data. Early results might show one variation outperforming the other, but these differences could even out as more data is collected. Ending a test too soon often leads to implementing changes that don’t truly improve performance—or worse, actually hurt it.
Statistical significance in A/B testing means you’re confident that the observed differences are real and not due to random chance. Reaching this level of confidence requires allowing the test to run long enough to account for natural variability in user behavior.
Best Practices for Allowing Tests to Run Fully
Set a Minimum Test Duration: Plan your tests to run for at least one full business cycle, typically a week or more, to capture daily and weekly variations in user behavior. For example, weekend traffic may behave differently than weekday traffic, and you need to account for these differences.
Determine the Required Sample Size: Use sample size calculators to estimate the number of visitors or actions needed to reach statistical significance. This ensures that your results are based on a sufficient amount of data, making them more reliable.
Monitor Without Interfering: While it’s important to track your test’s progress, avoid making changes or stopping the test early based on preliminary data. Let the test reach its natural conclusion before drawing any conclusions or declaring a winner.
Look for Statistical Confidence: Aim for a confidence level of at least 95% before determining a winner. This means there’s only a 5% chance that the observed difference is due to random variation rather than the changes you made.
Ignoring the Impact of External Factors
One of the most overlooked challenges in A/B testing is failing to account for external factors that can skew your results. External factors are events, trends, or circumstances outside your control that can affect user behavior during your test period. Examples include seasonal trends, major news events, holidays, and even unexpected changes in your competitors’ marketing strategies.
Why Ignoring External Factors is a Problem
External factors can have a significant influence on how users interact with your website or marketing campaigns, which in turn can distort the results of your A/B tests. For example, running a test during a holiday season might result in higher-than-usual traffic and conversions due to increased purchasing intent. Similarly, if a competitor launches a major promotion during your test, your traffic and conversions might drop, not because of the changes you tested, but due to the external competitive pressure.
When you ignore these factors, you risk making decisions based on data that doesn’t accurately reflect normal user behavior, leading to ineffective optimizations that don’t perform as expected when external influences subside.
How to Account for External Factors in A/B Testing
Avoid Testing During Unusual Periods: Schedule your A/B tests during stable periods when there are no major holidays, industry events, or seasonal trends that could impact user behavior.
Track External Influences: Keep a record of any external factors that might be impacting your test results, such as weather changes, public holidays, or significant news. This helps you contextualize the results and understand why certain variations might have performed differently.
Segment Data by Time and Context: Break down your test results by day, week, or user segment to identify any patterns that could be tied to external events. This segmentation can reveal whether a spike in conversions was truly due to your variation or if it coincided with an external factor.
Conduct Post-Test Analysis: After your test concludes, review any external influences that could have affected your results. If significant factors are identified, you might need to rerun the test during a more stable period or adjust your interpretation of the results.
By being mindful of external factors, you can better ensure that your A/B testing in marketing yields insights that are valid and applicable under normal circumstances.
Overlooking the User Experience
A significant mistake in A/B testing digital marketing is focusing solely on metrics like conversion rates or click-through rates while neglecting the broader user experience. While immediate metrics are crucial, they don’t always capture the full impact of your changes on user satisfaction, engagement, and long-term loyalty.
Why Overlooking User Experience is a Problem
Optimizing for quick wins like boosting conversions can sometimes come at the cost of user experience. For example, an aggressive pop-up might increase email sign-ups in the short term but also lead to higher bounce rates or reduced user satisfaction. Similarly, changes that improve one specific metric may lead to unintended consequences elsewhere, such as longer page load times, which frustrate users and increase abandonment rates.
Ignoring the overall user experience can lead to changes that hurt your brand in the long run, resulting in higher churn rates, lower customer satisfaction, and diminished lifetime value.
How to Incorporate User Experience in A/B Testing
Balance Short-Term Gains with Long-Term Goals: While conversion rate improvements are important, consider the broader implications of your changes. Test variations that enhance user experience alongside those that directly impact metrics like clicks or sales.
Track User Behavior Metrics: Beyond conversion-focused metrics, monitor user behavior indicators like bounce rate, session duration, and pages per session. If a variation leads to higher conversions but also results in more bounces or shorter session times, you might be sacrificing user experience for quick wins.
Gather Qualitative Feedback: Use surveys, heatmaps, and session recordings to gain insights into how users feel about your website changes. A variation that performs well in terms of conversions might still frustrate users in ways that quantitative data alone doesn’t reveal.
Consider Mobile and Desktop Experiences Separately: The user experience can differ greatly between mobile and desktop users. When running A/B testing on websites, ensure that your changes enhance usability across devices. For example, a layout change that works well on desktop might clutter the mobile experience, leading to higher drop-off rates.
By integrating user experience considerations into your A/B testing methodology, you can create a more balanced strategy that improves both immediate performance and long-term customer satisfaction.
How to Design an A/B Test?
A/B testing is a cornerstone strategy in digital marketing that allows businesses to make data-driven decisions by comparing two or more variations of a webpage, email, or other marketing elements. Properly designing an A/B test is crucial for gathering reliable data and deriving actionable insights. In this guide, we’ll walk through the essential steps for effectively designing an A/B test and ensuring your efforts lead to meaningful results.
Test Appropriate Items
To get the most out of your A/B testing in digital marketing, it’s important to focus on testing the right elements that have the potential to influence your key performance metrics significantly. The items you choose to test should directly align with your marketing objectives and the specific problem areas you want to address.
What Items to Test in A/B Testing
Headlines and Copy: One of the most common and effective elements to test is your headlines or copy. These are crucial for capturing user attention and communicating value propositions. For example, testing different headlines on a landing page or email subject lines can significantly affect click-through rates and conversions.
Call to Action (CTA) Buttons: CTAs are vital in guiding users toward the desired action, such as signing up for a newsletter or making a purchase. Testing variations in CTA text, color, size, and placement can help determine which combination maximizes conversions. For example, “Buy Now” might perform differently than “Get Started Today.”
Visual Elements: Images, videos, and other visual components are key drivers of user engagement. Testing different types of images (e.g., product photos vs. lifestyle images), video content, or even the placement of visuals can reveal what captures attention and encourages users to stay on the page longer.
Page Layout and Design: The layout and design of a webpage can dramatically impact user experience and conversion rates. A/B testing website elements such as navigation menus, content blocks, form placements, and color schemes can help identify the optimal layout that enhances usability and increases engagement.
Forms and Input Fields: If your goal is lead generation, testing different forms can be highly effective. You might experiment with the number of fields, required information, and form layout to find out which version minimizes friction and maximizes submissions.
Trust Elements: Testing the inclusion and placement of trust signals like customer testimonials, reviews, certifications, and security badges can build credibility and reduce user hesitancy. For example, experimenting with different types or locations of customer reviews can indicate which approach is most effective in reassuring potential buyers.
Best Practices for Choosing Test Items
Focus on High-Impact Changes: Prioritize elements that directly affect your key metrics, such as conversion rates, bounce rates, or click-through rates. Testing high-impact changes ensures that the results will be meaningful and actionable.
Start with Simple Tests: Begin with straightforward A/B tests before moving to more complex multivariate tests. For instance, test one headline against another before testing multiple combinations of headlines and CTAs.
Align with User Goals: Consider the user journey and what elements are most likely to influence decision-making at each stage. This will help you select test items that align with your users' needs and motivations.
Determine the Correct Sample Size
Determining the correct sample size is a critical step in designing an A/B test that yields statistically significant results. A test with too small a sample size may produce misleading results due to random chance, while a sample size that is too large could waste time and resources.
Why Sample Size Matters
The sample size determines how many visitors or actions are needed to detect a true difference between the control and the variation. If your sample size is too small, you risk not detecting a meaningful change (false negative) or finding a difference that doesn't exist (false positive). Conversely, a sample size that is too large can delay decision-making and lead to unnecessary costs.
How to Determine the Correct Sample Size
Use Sample Size Calculators: Several online tools, such as Optimizely or Google Optimize, offer sample size calculators that help you determine the minimum number of visitors required to achieve statistically significant results. These calculators consider factors like baseline conversion rate, minimum detectable effect, and desired confidence level.
Consider the Minimum Detectable Effect (MDE): The MDE is the smallest change in the metric you’re measuring that you consider significant. A smaller MDE requires a larger sample size, while a larger MDE requires a smaller one. Define what constitutes a meaningful difference for your business—e.g., a 5% increase in conversions vs. a 20% increase.
Set the Desired Confidence Level: In AB testing marketing, a confidence level of 95% is typically used, which means you are 95% sure that the results are not due to random chance. The higher the confidence level, the larger the sample size required.
Account for Variability in Conversion Rates: If the conversion rate of the current version (control) fluctuates significantly, you will need a larger sample size to detect a real difference. Stable conversion rates typically allow for smaller sample sizes.
Best Practices for Sample Size Calculation
Pre-Calculate the Sample Size: Before starting the test, always calculate the required sample size based on your specific test parameters. This ensures that the test has enough data to produce statistically significant results.
Monitor and Adjust as Needed: Keep an eye on the test as it runs, but avoid making changes mid-test unless absolutely necessary. If the test isn't reaching statistical significance within a reasonable timeframe, consider extending the duration or increasing the sample size.
Run Tests for a Sufficient Duration: Ensure the test runs long enough to capture a representative sample of user behavior. This may involve running the test for at least one business cycle to account for fluctuations in traffic or user behavior across different times of the day or week.
Check Your Data
Before you even begin an A/B test, it's essential to ensure that the data you rely on is accurate, comprehensive, and relevant. This step is crucial because the quality of your data will directly impact the validity and reliability of your test results.
Why Data Accuracy is Important in A/B Testing
Data forms the foundation of any A/B testing digital marketing strategy. If the data is flawed or incomplete, your test results will be unreliable, leading to poor decision-making and ineffective optimizations. For example, if there are discrepancies in your baseline data (like incorrect conversion rates or traffic sources), your test conclusions might be skewed, resulting in changes that do not improve, or even worsen, performance.
Best Practices for Checking Your Data:
Ensure Data Integrity: Confirm that the data being tracked is correct and accurately reflects user actions. This includes verifying that your analytics tools (like Google Analytics, HubSpot, or Optimizely) are properly set up and tracking all the necessary events, such as clicks, form submissions, and page views. For instance, if you’re conducting an A/B test on a website to improve the conversion rate, ensure that your conversion tracking is set up correctly and capturing all relevant data points.
Audit Your Metrics: Double-check the key metrics you plan to measure. Ensure that the definitions of these metrics are consistent across all platforms and stakeholders. For example, define what counts as a "conversion" to ensure all team members and tools are aligned. This clarity is essential for interpreting results correctly.
Clean Your Data: Remove any outliers or anomalies that could skew your results. For example, if a sudden spike in traffic is due to a bot or spam attack, exclude this data to maintain the integrity of your test. Make sure that all data being used in the test is clean and reliable.
Analyze Historical Data: Reviewing historical performance data can help set realistic expectations for your test and identify any trends or patterns that might impact the outcome. Understanding past performance helps you define benchmarks and anticipate how external factors might affect your test.
Run a Pre-Test Analysis: Conduct a preliminary analysis of your data to check for consistency and any existing biases. This helps you identify potential problems before running the full test and ensures that your starting point is accurate.
By checking your data thoroughly, you can ensure that your A/B testing methodology is grounded in accurate information, leading to more meaningful and actionable insights.
Schedule Your Tests
The timing of your A/B test can significantly impact its results. Understanding when to use A/B testing and scheduling tests properly is crucial for obtaining accurate and relevant data. Poor timing can introduce biases or skew results, leading to ineffective optimizations.
Why Scheduling Matters in A/B Testing
Scheduling your tests appropriately helps to capture a representative sample of user behavior. For example, user behavior can vary significantly based on the day of the week, time of day, season, or during special events like holidays or promotions. If your test runs only during a period of abnormal user activity, such as a holiday sale, the results may not be applicable to regular traffic patterns.
Best Practices for Scheduling A/B Tests:
Run Tests for a Full Business Cycle: To account for variations in user behavior, make sure your test runs for at least one full business cycle (e.g., a week or a month). This approach captures all possible fluctuations, such as differences in weekday vs. weekend traffic, and provides a more comprehensive picture of user behavior.
Avoid Peak Times for External Influences: Avoid scheduling your tests during periods of significant external influences, such as major holidays, product launches, or large promotional campaigns. These events can lead to abnormal traffic patterns and behavior that may not reflect your usual audience, skewing the test results.
Align with Marketing Activities: Coordinate your A/B tests with your overall marketing calendar. If you’re running a major campaign, such as a paid advertising push or email blast, ensure your test aligns with these activities. This coordination helps capture the true impact of your test without confounding factors.
Consider Time Zones and Global Audience: If your website serves a global audience, consider how different time zones might affect your test results. For example, traffic may peak at different times for different regions. Ensure that your test duration is long enough to capture data from all relevant time zones.
Monitor for Stability Before Launch: Before launching a test, monitor traffic and conversion stability for a few days to ensure there are no unusual fluctuations that could impact your results. This pre-test monitoring can help identify if an unexpected event (e.g., a sudden spike in traffic) might distort the test outcomes.
Set Start and End Dates Rigorously: Clearly define when your test will start and end, and stick to these dates. Avoid making adjustments mid-test, as this can introduce biases and invalidate the results. Set realistic timelines based on your calculated sample size and expected traffic.
By properly scheduling your A/B tests, you can ensure that the results are reliable and reflect normal user behavior, leading to more accurate conclusions and better optimization decisions.
Test Only One Element
One of the cardinal rules in A/B testing is to test only one element at a time. This approach, often referred to as "single-variable testing," ensures that any differences in performance between the control (Version A) and the variation (Version B) can be directly attributed to the specific change being tested.
Why Test Only One Element?
Testing multiple elements simultaneously can lead to inconclusive or misleading results. For example, if you change both the headline and the CTA button in an A/B test on a website, and you see an improvement in conversion rates, it’s impossible to know whether the headline change, the CTA button change, or the combination of both caused the uplift. This uncertainty undermines the reliability of your results and can result in implementing ineffective changes.
A/B testing in digital marketing relies on clarity and precision. By focusing on a single element, you can accurately identify which changes drive the desired outcomes and which do not, allowing for more targeted and effective optimizations.
Best Practices for Testing Only One Element
Prioritize High-Impact Elements: Focus on testing elements that are likely to have the most significant impact on your key metrics. Common elements to test in A/B testing marketing include:
Headlines: Different headlines can dramatically affect click-through rates and engagement.
Call to Action (CTA) Buttons: Changes to CTA text, color, size, or placement can significantly influence conversion rates.
Images and Visuals: Testing different types of images or visual content can impact how users interact with your site.
Page Layout and Design: Small changes to the layout or design, such as button placement or form arrangement, can lead to better usability and higher conversions.
Isolate the Variable: Ensure that the only difference between the control and variation is the single element being tested. Keep all other aspects of the test identical to accurately measure the impact of the change.
Document Each Test: Maintain a clear record of each test, including what element was changed, the hypothesis, and the results. This documentation helps track what has been tested and allows you to build on previous insights.
Run Sequential Tests: After testing one element, use the winning variation as the new control and test the next element. This iterative approach allows for continuous optimization without the confusion of testing multiple elements simultaneously.
Analyze the Data
After running an A/B test, analyzing the data correctly is crucial to determine whether the variation outperformed the control and by how much. This analysis helps you understand the effectiveness of the changes made and guides future testing decisions.
Steps to Effectively Analyze A/B Test Data
Ensure Statistical Significance: Before drawing any conclusions, ensure that your test results are statistically significant. In A/B testing, statistical significance indicates that the observed difference between the control and the variation is unlikely to have occurred by chance. Typically, a confidence level of 95% or higher is used in AB testing digital marketing to ensure the results are reliable.
Focus on Primary Metrics: Analyze the primary metrics you set at the beginning of the test, such as conversion rate, click-through rate (CTR), or bounce rate. These metrics should directly relate to your test hypothesis. For example, if your goal was to increase conversions by changing the CTA button color, the primary metric to analyze would be the conversion rate.
Consider Secondary Metrics: While your primary metric is the main focus, secondary metrics can provide additional insights into user behavior. For instance, if your test aimed to increase click-through rates, also consider metrics like time on page, bounce rates, and page views to understand the broader impact of the change.
Segment Your Data: Analyzing data by different segments—such as device type, traffic source, or user demographics—can reveal deeper insights. For example, a change that works well for desktop users might not perform as well on mobile devices. Segmenting your data helps you understand how different user groups respond to the variations.
Look for Patterns and Trends: Go beyond the surface-level data and look for patterns and trends that can help explain the results. For example, a variation might perform well on weekdays but poorly on weekends. Understanding these nuances allows you to make more informed decisions about when and where to implement changes.
Validate Your Findings with Confidence Intervals: Use confidence intervals to measure the precision of your test results. A narrow confidence interval indicates that the test result is likely to be closer to the true value, while a wider interval suggests more variability. Confidence intervals help gauge the reliability of your findings.
Document and Report Your Findings: Create a detailed report of the test results, including the hypothesis, methodology, key metrics, statistical significance, and conclusions. This documentation is vital for sharing insights with stakeholders, guiding future tests, and maintaining a record of what has been learned.
How to Conduct A/B Testing?
A/B testing is a fundamental technique in digital marketing that helps businesses optimize their websites, marketing strategies, and user experiences. By comparing two versions of a webpage, email, or other marketing element, you can determine which variation performs better and make data-driven decisions to enhance your overall strategy. To effectively conduct an A/B test, follow a structured process that includes defining objectives, selecting variables, running the test, and analyzing the results.
1. Define Your Objectives and Hypothesis
The first step in conducting an A/B test is to clearly define what you want to achieve and establish a hypothesis. This ensures that your test has a specific goal and provides a foundation for analyzing the results.
Best Practices:
Identify the Problem: Understand the issue you’re trying to solve, such as low conversion rates, high bounce rates, or low user engagement.
Set Clear Objectives: Determine the specific outcomes you want to measure, such as an increase in conversion rates, higher click-through rates (CTR), or reduced bounce rates. Align these objectives with your overall business goals.
Formulate a Testable Hypothesis: Create a hypothesis that defines what you believe will happen and why. For example, “Changing the color of the CTA button from blue to green will increase the conversion rate by 20%.” This hypothesis should be specific, measurable, and testable.
2. Select the Right Variable to Test
To accurately measure the impact of a change, focus on testing one variable at a time. In A/B testing in digital marketing, common elements tested include headlines, CTAs, images, layouts, and form fields.
Best Practices:
Prioritize High-Impact Variables: Choose elements that are likely to have the most significant impact on your key performance indicators (KPIs). For instance, test elements like CTA buttons, headlines, or page layouts that directly affect user behavior and conversions.
Ensure Relevance: The variable you choose to test should be directly related to the objectives you set. For example, if your goal is to increase click-through rates, testing different versions of email subject lines or ad copy would be appropriate.
3. Create Control and Variation
In A/B testing, the control is the original version of the element you are testing, while the variation is the modified version that includes the change you want to test.
Best Practices:
Design the Variation Thoughtfully: Ensure that the change in your variation is significant enough to potentially impact your key metrics. For example, if you’re testing headlines, make sure the variations differ substantially in wording or tone to yield clear results.
Keep Other Elements Consistent: Aside from the variable being tested, all other elements should remain the same between the control and variation to isolate the impact of the change.
4. Determine Your Sample Size and Duration
Calculating the correct sample size and setting an appropriate test duration are crucial to achieving statistically significant results in A/B testing. Too small a sample size or too short a test duration can lead to inconclusive results.
Best Practices:
Calculate the Required Sample Size: Use a sample size calculator to determine the minimum number of visitors or actions needed for statistically significant results. This calculation should consider factors such as the baseline conversion rate, minimum detectable effect (MDE), and desired confidence level.
Plan for an Appropriate Duration: The test should run long enough to account for natural variations in user behavior, such as weekday vs. weekend traffic. Ending the test prematurely can result in inaccurate data.
5. Randomly Distribute Traffic
To ensure the test results are reliable, it’s important to randomly split your traffic between the control and variation. This distribution helps eliminate biases and ensures that each version is exposed to a representative sample of your target audience.
Best Practices:
Use Reliable A/B Testing Tools: Tools like Google Optimize, Optimizely, or HubSpot provide built-in functionality for random traffic distribution, ensuring even and unbiased splits.
Consider Audience Segmentation: Depending on your objectives, segmenting your audience by device type, geographic location, or traffic source can provide deeper insights into how different groups respond to the variations.
6. Run the Test and Monitor Performance
Once the test is live, monitor the performance of both the control and variation in real-time. However, avoid making any changes during the test, as this can introduce biases and invalidate the results.
Best Practices:
Let the Test Run Its Course: Resist the temptation to stop the test early, even if preliminary results look promising. Allow the test to run until it reaches the required sample size and duration to ensure reliable data.
Track Key Metrics: Monitor the primary metric you are testing, such as conversion rate or CTR, and also keep an eye on secondary metrics like bounce rate and time on page to gain additional insights.
7. Analyze the Data
After the test concludes, it’s time to analyze the data to determine whether the variation outperformed the control. This analysis helps you understand the effectiveness of the changes and guides future testing decisions.
Best Practices:
Ensure Statistical Significance: Check that your test results are statistically significant before drawing conclusions. A confidence level of 95% or higher is typically used to ensure the observed differences are not due to random chance.
Evaluate Both Primary and Secondary Metrics: Focus on the primary metric that aligns with your objective, but also consider secondary metrics to understand the broader impact of the changes.
Segment Your Results: Analyze the data by different segments—such as device type, traffic source, or user demographics—to gain a more granular understanding of how various user groups responded to the changes.
8. Implement the Winning Variation and Iterate
If the variation proves to be more effective, implement it across your marketing channels. However, optimization is an ongoing process, and the insights gained from one test should inform future tests.
Best Practices:
Document Your Findings: Keep a detailed record of the test setup, hypothesis, results, and conclusions. This helps build a knowledge base for future tests and ensures that valuable insights are not lost.
Continue Testing: A/B testing is an iterative process. Use the results of one test to refine your approach and develop new hypotheses for continuous optimization.
Five A/B Testing Use Cases
A/B testing is a powerful tool in digital marketing that allows businesses to make data-driven decisions to optimize their strategies and improve performance. By testing different versions of a webpage, email, ad, or other marketing elements, you can determine which variation performs better and make informed changes that enhance user experience and conversions. Here are five common use cases for A/B testing in digital marketing that illustrate how this method can be applied to various aspects of your marketing strategy.
Email Marketing
A/B testing is an essential tool for optimizing email marketing campaigns. Emails are a vital part of any digital marketing strategy, serving to engage customers, promote products, and drive conversions. Through A/B testing, marketers can test various elements within emails to find the most effective ways to boost open rates, click-through rates (CTR), and conversion rates.
Key Elements to Test in Email Marketing:
Subject Lines: The subject line is the first thing a recipient sees, and it often determines whether the email will be opened or ignored. A/B testing subject lines is a typical use of A/B testing in marketing. Variations might include testing different lengths, tones (e.g., casual vs. formal), personalization (e.g., including the recipient's name), and incorporating emojis or punctuation.
Example: Testing a subject line like "Don't Miss Out: Exclusive Offer Just for You!" against "Your Special Discount Awaits, [Name]!" can help determine which phrasing resonates more with your audience.Content Layout and Length: The design and length of the email content can significantly influence engagement. Testing different layouts—such as text-heavy emails vs. image-centric emails—can reveal what drives more clicks and conversions. Short, concise emails may lead to better engagement, while longer, more detailed emails might work better for specific audiences. Example: Testing an email with a single-column layout featuring a large image and brief text against a multi-column format with detailed descriptions can highlight which structure performs better.
Call to Action (CTA) Buttons: The CTA is the primary driver for the desired action in an email, such as “Buy Now,” “Learn More,” or “Sign Up Today.” Testing different CTA texts, colors, button sizes, and placements can reveal which combinations are most effective in encouraging user clicks and actions. Example: Testing a CTA that says “Start Your Free Trial” against another that says “Claim Your Free Trial Now” helps identify which wording prompts more clicks.
Send Time and Frequency: The timing and frequency of email sends can impact open and click-through rates. A/B testing can help determine the best time of day or day of the week to send emails, as well as the optimal frequency to avoid overwhelming the audience or causing them to unsubscribe. Example: Testing email sends at different times—such as 8 a.m. vs. 3 p.m.—or different days of the week can provide insights into when your audience is most likely to engage.
Benefits: By applying A/B testing to email marketing, businesses can enhance the effectiveness of their campaigns, increase open and click-through rates, and ultimately drive more conversions. Testing different elements provides a clear understanding of audience preferences and behavior, enabling continuous optimization.
Landing Page Design
Landing pages are one of the most critical components in digital marketing for converting visitors into leads or customers. They are often the first point of interaction with potential customers, making their design and functionality crucial. A/B testing is an effective way to determine which landing page elements—such as headlines, images, CTAs, and forms—are most effective at driving conversions.
Key Elements to Test in Landing Page Design:
Headlines and Subheadings: The headline is often the first element visitors see and can greatly influence their decision to stay or leave the page. A/B testing different headlines and subheadings allows marketers to determine which messages best capture attention and convey the value proposition. Example: Testing a headline like “Boost Your Sales with Our Marketing Tool” against “Transform Your Business with Our All-in-One Solution” can show which phrasing generates more engagement.
Hero Images and Visual Content: Visual elements such as hero images, videos, and graphics can significantly impact user experience and conversions. A/B testing different types of images or videos—such as a product-focused image vs. a lifestyle image—can help identify what resonates more with visitors. Example: Testing a hero image featuring a product in use against a customer testimonial video can help determine which visual approach leads to better engagement.
Form Placement and Length: Forms are often used to capture visitor information, such as email addresses or other contact details. Testing form placement (e.g., above the fold vs. below the fold) and length (e.g., shorter forms with fewer fields vs. longer forms with more fields) can help identify the best balance between collecting valuable data and reducing friction for the user. Example: Testing a short form with only three fields (name, email, phone number) against a longer form that also asks for additional information (e.g., company size, industry) can reveal which option drives more submissions.
Call to Action (CTA) Buttons: Like in email marketing, CTA buttons on landing pages play a vital role in driving conversions. A/B testing different button texts, colors, sizes, and placements can help identify which combinations are most effective at motivating visitors to take action. Example: Testing a green “Get Started Now” button vs. a red “Learn More” button can help determine which color and text combination performs better.
Layout and Navigation: The overall layout and navigation of a landing page can greatly influence user behavior. Testing different layouts—such as a single-column vs. multi-column format or adding/removing navigation menus—can help determine which design provides the best user experience and drives the most conversions. Example: Testing a page layout with minimal navigation options to keep users focused on the CTA vs. a layout with multiple navigation links can help identify the most effective design.
Benefits: By using A/B testing on landing page elements, businesses can continuously refine their designs to maximize conversions. Understanding which headlines, visuals, CTAs, and layouts resonate most with visitors enables marketers to create more compelling and effective landing pages that align with user preferences and behaviors.
Text Ad Optimization
Text ads are a fundamental component of many digital marketing strategies, particularly in search engine advertising platforms like Google Ads and Microsoft Advertising. They consist of a headline, description, and sometimes extensions (like callouts or sitelinks) and are designed to capture user attention and drive clicks. A/B testing in marketing for text ads is essential for refining ad copy and improving performance metrics such as click-through rates (CTR), quality scores, and conversion rates.
Key Elements to Test in Text Ad Optimization:
Headlines: The headline is the most prominent part of a text ad and can significantly impact its effectiveness. A/B testing different headlines—such as varying the length, wording, or style (e.g., including numbers, questions, or emotional triggers)—can help determine which version resonates most with the target audience.
Example: Testing a headline like "Save 20% on Your First Order" against "Exclusive Discount for New Customers" can reveal which phrasing drives more clicks.Description Text: The ad description provides additional details about the offer or product. Testing variations in description text, such as different calls to action (CTAs), value propositions, or benefits, can help optimize the ad's performance.
Example: Comparing a description that emphasizes "Free Shipping on All Orders" versus one that highlights "24/7 Customer Support" can indicate which benefit is more appealing to users.Display URL and Paths: Some advertisers test different display URLs or paths (the customizable part of a URL that appears in the ad) to see if they affect CTR. While the actual URL remains the same, varying the path—such as "/Sale" vs. "/Exclusive-Deals"—can make the ad seem more relevant and attractive.
Example: Testing "/New-Arrivals" against "/Best-Sellers" can help identify which path garners more user interest.Ad Extensions: Ad extensions, like sitelinks, callouts, and structured snippets, provide additional information and enhance the ad's visibility. Testing different extensions or their arrangements can help identify which combinations lead to better engagement.
Example: Testing sitelinks like “About Us,” “Customer Reviews,” and “FAQs” against “Free Consultation,” “Instant Quote,” and “Contact Us” to determine which set drives more clicks.
Benefits: By applying A/B testing methodology to text ad optimization, marketers can continually refine their ad copy to improve CTR, quality score, and overall ad performance. This process leads to more efficient ad spending, higher relevance, and better ROI.
Display Ad Optimization
Display ads are visual advertisements that appear on websites, social media platforms, or apps. They often include images, graphics, videos, and text, and are designed to capture attention and drive awareness, engagement, or conversions. A/B testing display ads is crucial for understanding what visual elements, messaging, and layouts are most effective for engaging the target audience.
Key Elements to Test in Display Ad Optimization:
Images and Visuals: Visual content is a key component of display ads. Testing different types of images—such as product photos, lifestyle images, or illustrations—can reveal what resonates most with the audience. Marketers can also test visual elements like color schemes, image quality, and placement within the ad.
Example: Testing a display ad featuring a close-up of a product against one showing the product in use by a customer can help determine which approach generates more engagement.Ad Copy and Text Overlays: The text included in display ads, whether it’s overlaid on the image or presented alongside it, plays a critical role in communicating the ad’s message. Testing different taglines, CTAs, or benefit statements can help identify which copy drives higher engagement.
Example: Comparing an ad with a simple "Buy Now" CTA against one that says "Limited Time Offer—Shop Now!" can show which drives more clicks.Call to Action (CTA) Buttons: Just like in text ads, CTA buttons in display ads are vital for encouraging users to take the desired action. Testing different button texts, sizes, shapes, and colors can help determine which combinations are most effective.
Example: Testing a red “Learn More” button versus a green “Get Started” button can help identify which design yields a better click-through rate.Ad Formats and Sizes: Display ads come in various formats and sizes, including banners, skyscrapers, rectangles, and squares. Testing different formats and dimensions can help determine which sizes work best on specific platforms or for particular audiences.
Example: Running an A/B test comparing a 300x250 rectangle ad against a 728x90 leaderboard ad on the same website can show which format has a higher engagement rate.Animation vs. Static Ads: Animated ads often attract more attention than static ones, but they can also be more distracting. Testing animated ads (like GIFs or video ads) against static images can help determine which type drives better results for a given campaign.
Example: Comparing a static display ad with a simple product image to an animated ad showing multiple product features can highlight which type performs better in terms of engagement and conversion.
Benefits: Using A/B testing for display ad optimization allows marketers to continuously refine and improve their ad creative, ensuring they capture audience attention and drive engagement. By identifying the best-performing visuals, messaging, and formats, businesses can maximize their display ad effectiveness, reduce costs, and increase ROI.
eCommerce Websites
For eCommerce businesses, optimizing every element of the website is critical to converting visitors into paying customers. A/B testing in digital marketing is essential for identifying which changes will increase sales, reduce cart abandonment, and enhance user experience. By systematically testing different variations of key elements on an eCommerce website, marketers can make data-driven decisions that lead to better outcomes.
Key Elements to Test on eCommerce Websites:
Product Page Layout and Design
Product pages are a crucial part of any eCommerce website. The way information is presented—such as product images, descriptions, pricing, and reviews—can have a significant impact on a visitor’s decision to make a purchase. A/B testing can help determine the optimal layout and design elements to maximize conversions.
Product Images: Test different types of product images, such as lifestyle images versus plain product shots, or varying the number of images displayed. Experiment with the size, zoom functionality, and placement of images to see which version drives more engagement and conversions.
Example: Comparing a product page with a single large hero image against another with a carousel of multiple images to determine which layout increases the likelihood of adding the product to the cart.Product Descriptions: Experiment with different lengths and styles of product descriptions—such as bullet points versus paragraphs, or benefits-focused versus feature-focused copy—to see which version leads to more purchases.
Example: Testing a concise product description highlighting the top three benefits against a longer, detailed description covering all features can help identify the ideal length and format.
Call to Action (CTA) Buttons
CTA buttons are critical for guiding users through the purchasing process. Testing different aspects of CTA buttons—such as text, color, size, and placement—can help identify the most effective design to encourage conversions.
Button Text: Test variations of CTA text, such as “Buy Now,” “Add to Cart,” or “Shop Now,” to see which phrasing results in higher conversion rates.
Example: Testing “Buy Now” against “Add to Cart” can provide insights into which action resonates more with customers and drives them to complete a purchase.Button Color and Size: Experiment with different colors (e.g., green vs. red) and sizes (e.g., small vs. large) to determine which combination attracts the most attention and leads to more clicks.
Example: Testing a large, bright-colored CTA button against a smaller, more muted one can help determine which design catches the eye and drives action.
Checkout Process
The checkout process is where many eCommerce sites lose potential customers due to friction or confusion. A/B testing can be used to optimize this process by testing different checkout flows, form fields, and payment options.
Checkout Flow: Test single-page checkout vs. multi-step checkout to see which version results in fewer cart abandonments. Analyze which flow provides a smoother and quicker experience for users.
Example: Testing a single-page checkout that displays all fields on one page against a multi-step checkout process can help determine which method is more effective in reducing drop-offs.Form Fields: Experiment with the number of required fields during checkout to find the optimal balance between gathering necessary information and minimizing user friction.
Example: Testing a checkout form with minimal fields (e.g., name, address, payment information) against one with additional fields (e.g., phone number, promotional code) can reveal which version leads to higher completion rates.
Pricing and Promotions
Pricing and promotional strategies are crucial for influencing buying decisions. A/B testing different price points, discount offers, and promotional messages can help identify what motivates customers to make a purchase.
Discount Messaging: Test different ways of presenting discounts, such as a percentage off (e.g., “20% Off”) vs. a dollar amount off (e.g., “$10 Off”). Experiment with how discounts are displayed on the product page or in the cart.
Example: Testing a “20% Off” banner at the top of a product page against a “Save $10” message near the CTA button can show which format is more compelling.Pricing Display: Experiment with how prices are presented, such as showing the original price with a strikethrough next to the discounted price, or using psychological pricing (e.g., $9.99 vs. $10).
Example: Testing “$9.99” against “$10.00” can help determine which pricing strategy is more appealing to customers and leads to more sales.
Navigation and Search Functionality
Effective navigation and search functionality are vital for helping customers find the products they want quickly and easily. A/B testing different navigation structures and search features can improve user experience and increase the likelihood of conversions.
Navigation Menus: Test different types of navigation menus, such as horizontal vs. vertical menus or mega menus vs. simple dropdowns, to see which structure helps users find products more efficiently.
Example: Testing a sticky navigation menu that stays at the top of the screen against a standard, non-sticky menu can help determine which design improves site navigation and reduces bounce rates.Search Bar Placement and Functionality: Experiment with the placement of the search bar, such as at the top center vs. the top right, and test different search functionalities, like predictive search or category-specific filters, to see what leads to more successful searches and higher conversions.
Example: Testing a search bar with auto-suggest features against a basic search bar can help identify which option leads to better user experiences and more completed purchases.
Benefits: By applying A/B testing on eCommerce websites, businesses can optimize critical elements that directly impact user experience and conversion rates. Testing different layouts, CTAs, checkout processes, pricing, and navigation options enables continuous refinement, ensuring that the website is always aligned with customer preferences and behavior.
A/B Testing Process Overview
A/B testing is a crucial method in digital marketing that involves comparing two versions of a webpage, email, ad, or other marketing elements to determine which performs better. The A/B testing process allows marketers to make data-driven decisions by testing specific variables and measuring their impact on key performance indicators (KPIs). By following a systematic approach, businesses can effectively optimize their digital marketing strategies to improve conversion rates, enhance user experience, and achieve better overall results. Here is an overview of the A/B testing process:
Identify Opportunities for Improvement
The first step in the A/B testing process is to identify areas where there is potential for improvement. This involves analyzing current performance metrics to pinpoint where your marketing efforts are falling short and where changes could lead to significant gains.
How to Identify Opportunities:
Analyze Current Performance Data: Start by reviewing your analytics data to identify underperforming areas. Look for high bounce rates, low conversion rates, poor click-through rates (CTR), or low engagement levels. These metrics can indicate pages or elements that may benefit from optimization through A/B testing.
For example, a landing page with a high bounce rate may indicate that visitors are not finding the content relevant or engaging. This could be an opportunity to test different headlines, layouts, or images.
Understand User Behavior: Use tools like heatmaps, session recordings, and user surveys to understand how visitors interact with your website or marketing content. Identifying patterns—such as where users click most, where they drop off, or which elements they ignore—can provide valuable insights into areas that may need improvement.
For instance, if a heatmap shows that users are not clicking on a CTA button, this might be an indication that the button is not prominent enough or that the messaging isn't compelling.
Prioritize High-Impact Elements: Focus on elements that have the potential to make a significant impact on key performance indicators (KPIs). Common areas to target in A/B testing digital marketing include headlines, call-to-action (CTA) buttons, images, navigation menus, and form fields. These elements are directly tied to user experience and conversion rates.
For example, testing different variations of a CTA button's color, text, or placement could lead to a higher conversion rate.
Consider Business Goals: Align your testing opportunities with broader business objectives. If your goal is to increase sales, focus on testing elements that directly influence the purchasing process, such as checkout flows or product descriptions. If your goal is to generate leads, test landing page forms or email sign-up offers.
For instance, if the objective is to grow an email list, test different lead magnet offers or form placements to determine what drives more sign-ups.
By systematically identifying opportunities for improvement, you ensure that your A/B testing efforts are focused on areas with the highest potential for positive impact.
Create a Hypothesis
Once you have identified the opportunities for improvement, the next step in the A/B testing process is to create a hypothesis. A hypothesis is a clear, testable statement that predicts how a specific change will affect your KPIs. It serves as the foundation for your test, guiding what you test, how you test it, and how you measure success.
How to Create an Effective Hypothesis:
Define the Problem: Begin by clearly defining the problem you want to solve or the metric you want to improve. For example, if your analysis shows that your website has a high bounce rate, your problem might be that visitors are not engaging with the content.
A clear problem definition helps focus your hypothesis on specific outcomes, ensuring the test is targeted and relevant.
Make It Specific and Measurable: A good hypothesis should be specific, measurable, and directly related to your business objectives. Avoid vague or broad statements. Instead, pinpoint the exact change you want to test and the expected outcome.
Example: "Changing the headline of the landing page from 'Welcome to Our Website' to 'Discover Your Perfect Solution' will reduce the bounce rate by 10%." This hypothesis is specific (headline change), measurable (bounce rate reduction by 10%), and directly tied to an objective (improving engagement).
Identify the Variable to Test: Focus on one variable at a time to ensure clarity and precision in your results. For instance, if you want to increase conversions, you might test variations of the CTA button, such as different colors, sizes, or text.
Testing one variable at a time allows you to attribute any change in performance to that specific variable, providing a clear understanding of what works and what doesn’t.
Explain the Reasoning: Include a rationale for why you believe the proposed change will result in the desired outcome. Use data, user behavior insights, or best practices to support your hypothesis.
For example: "Changing the CTA button color to red may increase conversions because red is known to create a sense of urgency and attract attention, as indicated by previous studies."
Outline the Expected Impact: Describe what you expect to happen if your hypothesis is correct. Define the primary metric that will be used to measure success, such as a 15% increase in conversions or a 20% reduction in bounce rate.
An example might be: "If we add customer testimonials to the product page, we expect to see a 15% increase in conversion rates because social proof enhances trust and credibility."
By crafting a well-defined hypothesis, you establish a clear direction for your A/B testing efforts, ensuring that each test is purposeful and aligned with your marketing goals.
Craft Variants
Crafting variants is an essential step in the A/B testing methodology. In this phase, you create different versions of the element you want to test based on your hypothesis. The goal is to design variations that will allow you to measure the impact of specific changes on your key performance indicators (KPIs).
How to Craft Effective Variants:
Start with the Control Version: The control is the original version of the element you are testing, such as the current version of a webpage, email, or ad. The control serves as the baseline against which all other variants will be compared.
For example, if you are testing a landing page, the control version would be the existing page that you believe could perform better.
Design the Variation(s): The variation is the modified version that incorporates the change you want to test. To ensure clarity in your results, it is essential to test only one variable at a time. For instance, if you want to test a headline, only change the headline text while keeping other elements constant.
Examples of Variations:
Headlines: Test different headline versions to determine which captures more attention.
CTA Buttons: Experiment with various call-to-action (CTA) buttons, such as changing their color, size, or text.
Images: Test different images on a landing page to see which ones resonate best with the audience.
Ensure Distinct Differences: The variants you create should have clear and noticeable differences from the control. Minor tweaks may not produce significant changes in user behavior, making it harder to identify a winning variant.
For example, if you are testing a CTA button, a meaningful change might involve altering both the button color and text (e.g., from "Learn More" in blue to "Get Started" in green) rather than just a slight shade change.
Align Variants with Hypothesis: Ensure that the changes made in the variants directly relate to your hypothesis. The variations should be designed to test the specific aspect you believe will impact your KPIs.
For instance, if your hypothesis is that changing the headline will reduce bounce rates, then your variants should focus solely on different headline texts while keeping all other elements the same.
Keep the User Experience in Mind: While crafting variants, consider the overall user experience. Changes that might increase conversions but negatively impact usability or satisfaction should be avoided. Aim to enhance both performance and user experience.
For example, if you test a more aggressive pop-up for collecting email addresses, monitor not only the subscription rate but also any changes in bounce rates or user feedback.
By crafting well-defined variants, you can create meaningful experiments that provide clear, actionable insights into what elements work best in your digital marketing efforts.
Run a Content Experiment
Once you have crafted your variants, the next step is to run a content experiment. This phase involves launching the test, distributing traffic between the control and variants, and gathering data to determine which version performs best.
How to Run a Successful Content Experiment:
Use an A/B Testing Tool: Utilize an A/B testing tool like Google Optimize, Optimizely, or HubSpot to run your content experiment. These tools provide the functionality needed to set up the test, randomly distribute traffic between the control and variants, and track key metrics.
Website A/B Testing tools automatically handle traffic distribution, ensuring that each variant receives a representative sample of visitors.
Set Clear Parameters: Define the parameters of your experiment, including the sample size, test duration, and confidence level. The sample size should be large enough to ensure statistically significant results, while the test duration should capture variations in user behavior across different days and times.
For example, if your goal is to test a new headline on a landing page, you might set the test to run for two weeks with a minimum sample size of 1,000 unique visitors to ensure reliable data.
Randomly Distribute Traffic: Ensure that visitors are randomly assigned to either the control or one of the variants. Random distribution eliminates biases and ensures that each version is exposed to a representative sample of your target audience.
For example, half of your visitors could see the control version, while the other half sees the variant. This even split ensures that any differences in performance can be attributed to the changes you made.
Monitor the Experiment in Real Time: Keep an eye on the test as it runs to ensure there are no technical issues, such as broken links or tracking errors, that could affect the results. However, avoid making changes mid-test, as this can introduce biases and invalidate your findings.
Monitoring tools can help track metrics like conversion rate, bounce rate, and click-through rate (CTR) to ensure that everything is functioning correctly.
Analyze the Results: Once the experiment has reached its predetermined duration or sample size, analyze the data to determine which variant performed better. Focus on the primary metric that aligns with your hypothesis, but also consider secondary metrics to understand the broader impact of the changes.
For example, if your primary metric was the conversion rate, also look at other metrics like average session duration and page views to gain additional insights.
Check for Statistical Significance: Ensure that the results are statistically significant before making any conclusions. A typical confidence level is 95%, which means you are 95% confident that the observed differences are not due to random chance.
Statistical tools and calculators provided by A/B testing platforms can help you verify significance and avoid acting on false positives.
Implement the Winning Variant: If one variant clearly outperforms the control, implement it across your marketing channels. Use the insights gained to inform future tests and continuous optimization.
For instance, if a new CTA button color led to a 20% increase in conversions, roll out this change site-wide and consider testing other elements to drive further improvements.
Measure and Analyze Results
After running an A/B test, the most important step is to measure and analyze the results. This phase is critical because it determines whether the changes you made in your variant had the desired effect on your key performance indicators (KPIs) and helps you decide what to do next. Accurate measurement and analysis will allow you to make data-driven decisions and optimize your digital marketing efforts.
How to Measure and Analyze A/B Test Results:
Collect Relevant Data
The first step in analyzing an A/B test is to collect all relevant data from your testing tool, such as Google Optimize, Optimizely, or HubSpot. These tools provide a comprehensive overview of key metrics, such as conversion rates, click-through rates (CTR), bounce rates, and other engagement metrics, which will help you understand the performance of each variant.
Focus on Primary Metrics: Ensure you have clear data for the primary metric you set at the start of the test, such as an increase in conversion rates or a reduction in bounce rates. This metric is directly aligned with your hypothesis and represents the main goal of the test.
Consider Secondary Metrics: While the primary metric is the focus, secondary metrics can provide additional insights. For example, if your primary metric is the conversion rate, also look at the average session duration, page views, and user engagement to understand the broader impact of the changes.
Ensure Statistical Significance
Before concluding that one variant is better than the other, ensure that the results are statistically significant. Statistical significance means that the observed difference between the control and variant is unlikely to have occurred by chance and can be confidently attributed to the changes made in the test.
Set a Confidence Level: A standard confidence level in A/B testing digital marketing is 95%. This level means you can be 95% confident that the results are reliable and not due to random chance. Most A/B testing tools provide a built-in calculator to determine statistical significance.
Calculate Sample Size: Make sure your sample size is large enough to achieve statistical significance. Running tests with too small a sample size may lead to misleading results. Use sample size calculators provided by A/B testing platforms to determine the minimum sample needed to achieve reliable results.
Compare Performance Between Variants
Once you have confirmed statistical significance, compare the performance of the control and variant based on the primary metric. Look for any meaningful differences between the two to determine which variant performed better.
Calculate Percentage Difference: Calculate the percentage difference in performance between the control and the variant. For example, if the conversion rate for the control is 3% and the conversion rate for the variant is 4.5%, the variant has a 50% higher conversion rate than the control.
Review Secondary Metrics: Analyze secondary metrics to identify any unintended consequences or additional benefits of the change. For example, a variant may have a higher conversion rate but also a higher bounce rate. Understanding these trade-offs helps you make more informed decisions.
Segment Your Data for Deeper Insights
Segmenting your data allows you to understand how different groups within your audience responded to the test. This segmentation can reveal valuable insights that a general overview might miss.
Analyze by Demographics: Review the results by demographic segments, such as age, gender, location, or device type. For example, a new CTA might perform well for mobile users but poorly for desktop users.
Consider Traffic Sources: Look at how different traffic sources (e.g., organic, paid, social) responded to the variants. For instance, one version might work better for visitors from social media, while another performs better for organic search traffic.
Behavioral Segmentation: Segment results based on user behavior, such as new vs. returning visitors or high-value vs. low-value customers. This analysis helps identify how different user segments interact with the changes made.
Interpret the Results and Draw Conclusions
Once you have analyzed the data, interpret the results to determine whether the hypothesis was correct and which variant is the winner. Understanding what worked and why is crucial for applying these insights to future tests and optimizations.
Validate Your Hypothesis: Determine whether the changes made in the variant led to the expected outcome defined in your hypothesis. For example, if your hypothesis was that a new headline would increase conversions, check whether the data supports this claim.
Understand the Broader Impact: Beyond validating your hypothesis, consider the broader impact of the changes. Did the winning variant improve other areas, such as engagement or user satisfaction? This broader perspective ensures you are optimizing for overall user experience, not just a single metric.
Make Data-Driven Decisions and Plan Next Steps
Based on the findings, make informed decisions about the next steps for your digital marketing strategy. If the test results show a clear winner, implement the winning variant across your channels. If the results are inconclusive, consider running additional tests to refine your approach.
Implement the Winning Variant: If a variant outperforms the control, implement it permanently on your website or marketing channel. This decision should align with your business goals and objectives.
Iterate and Test Further: Use the insights gained from the test to plan future A/B tests. Continuous testing allows you to build on previous successes and learn from past experiments. For example, if changing the CTA text increased conversions, your next test might focus on optimizing the CTA button color or placement.
Document Your Findings: Keep a detailed record of the test setup, hypothesis, results, and conclusions. This documentation helps build a knowledge base for future tests and ensures valuable insights are not lost.
Conclusion
A/B testing in digital marketing serves as a cornerstone for data-driven decision-making, allowing marketers to refine and optimize their campaigns with precision. By systematically comparing two versions of a marketing asset—be it a webpage, email, or advertisement—marketers can identify which variant resonates more effectively with their audience, leading to higher engagement, increased conversions, and improved ROI. The iterative nature of A/B testing encourages continuous experimentation and optimization, which is vital in the ever-evolving digital landscape. Each test provides valuable insights into consumer preferences and behaviors, enabling businesses to make informed decisions that enhance user experience and drive better business outcomes.
Ultimately, A/B testing is not just a technique but a strategic necessity in digital marketing. It empowers businesses to understand their audience better, optimize every element of their marketing campaigns, and achieve sustained growth. By leveraging A/B testing, marketers can continuously refine their strategies to align with evolving consumer behaviors and preferences, ensuring they remain relevant and competitive in a dynamic digital marketplace. The ability to run multiple tests, analyze data in real-time, and adapt quickly is what makes A/B testing an indispensable tool for any business looking to maximize its digital marketing efforts.
FAQs
What is a good sample size for A/B testing?
A good sample size for A/B testing depends on your desired confidence level and the expected impact of the change; tools like a sample size calculator can help determine this based on your current traffic and conversion rates.
How long should an A/B test run?
An A/B test should run long enough to gather a statistically significant amount of data, typically until a confidence level of at least 95% is reached, which often means running the test for 1-2 weeks or longer.
What are some common mistakes to avoid in A/B testing?
Common mistakes in A/B testing include testing too many variables at once, stopping the test too early, ignoring statistical significance, and not segmenting the audience properly.